A Swarm Variant for the Schr\"odinger Solver
arxiv(2021)
摘要
This paper introduces application of the Exponentially Averaged Momentum Particle Swarm Optimization (EM-PSO) as a derivative-free optimizer for Neural Networks. It adopts PSO's major advantages such as search space exploration and higher robustness to local minima compared to gradient-descent optimizers such as Adam. Neural network based solvers endowed with gradient optimization are now being used to approximate solutions to Differential Equations. Here, we demonstrate the novelty of EM-PSO in approximating gradients and leveraging the property in solving the Schr\"odinger equation, for the Particle-in-a-Box problem. We also provide the optimal set of hyper-parameters supported by mathematical proofs, suited for our algorithm.
更多查看译文
关键词
swarm variant
AI 理解论文
溯源树
样例
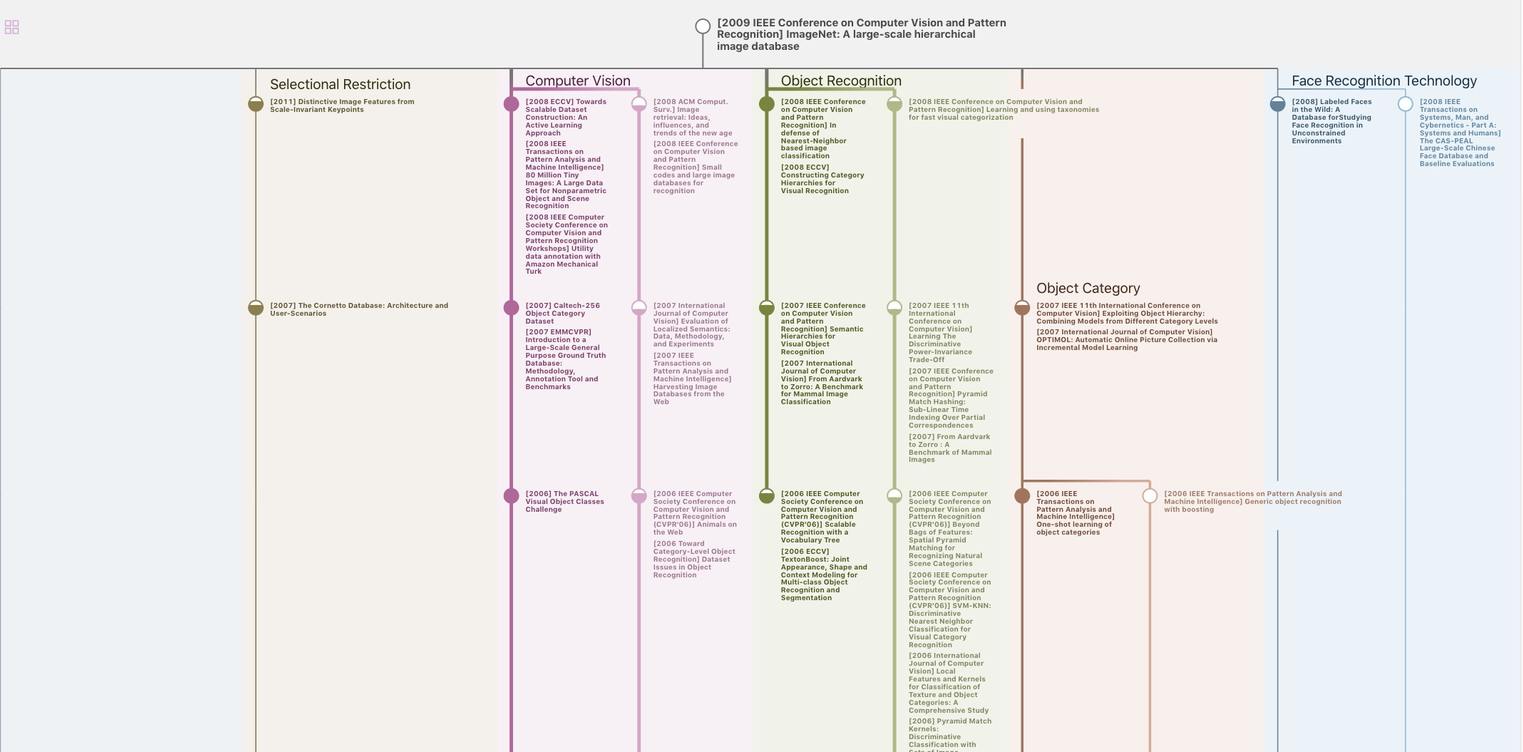
生成溯源树,研究论文发展脉络
Chat Paper
正在生成论文摘要