Multi-Label Classification And Label Dependence In In Silico Toxicity Prediction
TOXICOLOGY IN VITRO(2021)
摘要
Most computational predictive models are specifically trained for a single toxicity endpoint and lack the ability to learn dependencies between endpoints, such as those targeting similar biological pathways. In this study, we compare the performance of 3 multi-label classification (MLC) models, namely Classifier Chains (CC), Label Powersets (LP) and Stacking (SBR), against independent classifiers (Binary Relevance) on Tox21 challenge data. Also, we develop a novel label dependence measure that shows full range of values, even at low prior probabilities, for the purpose of data-driven label partitioning.Using Logistic Regression as the base classifier and random label partitioning (k = 3), CC show statistically significant improvements in model performance using Hamming and multi-label accuracy scores (p<0.05), while SBR show significant improvements in multi-label accuracy scores. The weights in the Logistic Regression and Stacking models are positively associated with label dependencies, suggesting that learning label dependence is a key contributor to improving model performance.An original quantitative measure of label dependency is combined with the Louvain community detection method to learn label partitioning using a data-driven process. The resulting MLCs with learned label partitioning were generally found to be non-inferior to their corresponding random or no label partitioning counterparts. Additionally, using the Random Forest classifier in a 10-fold stratified cross validation Stacking model, we find that the top-performing stacking model out-performs the corresponding base model in 11 out of 12 Tox21 labels. Taken together, these results suggest that MLC models could potentially boost the performance of current single endpoint predictive models and that label partitioning learning may be used in place of random label partitionings.
更多查看译文
关键词
Multi-label classification, Tox21, Label dependence, Toxicity prediction
AI 理解论文
溯源树
样例
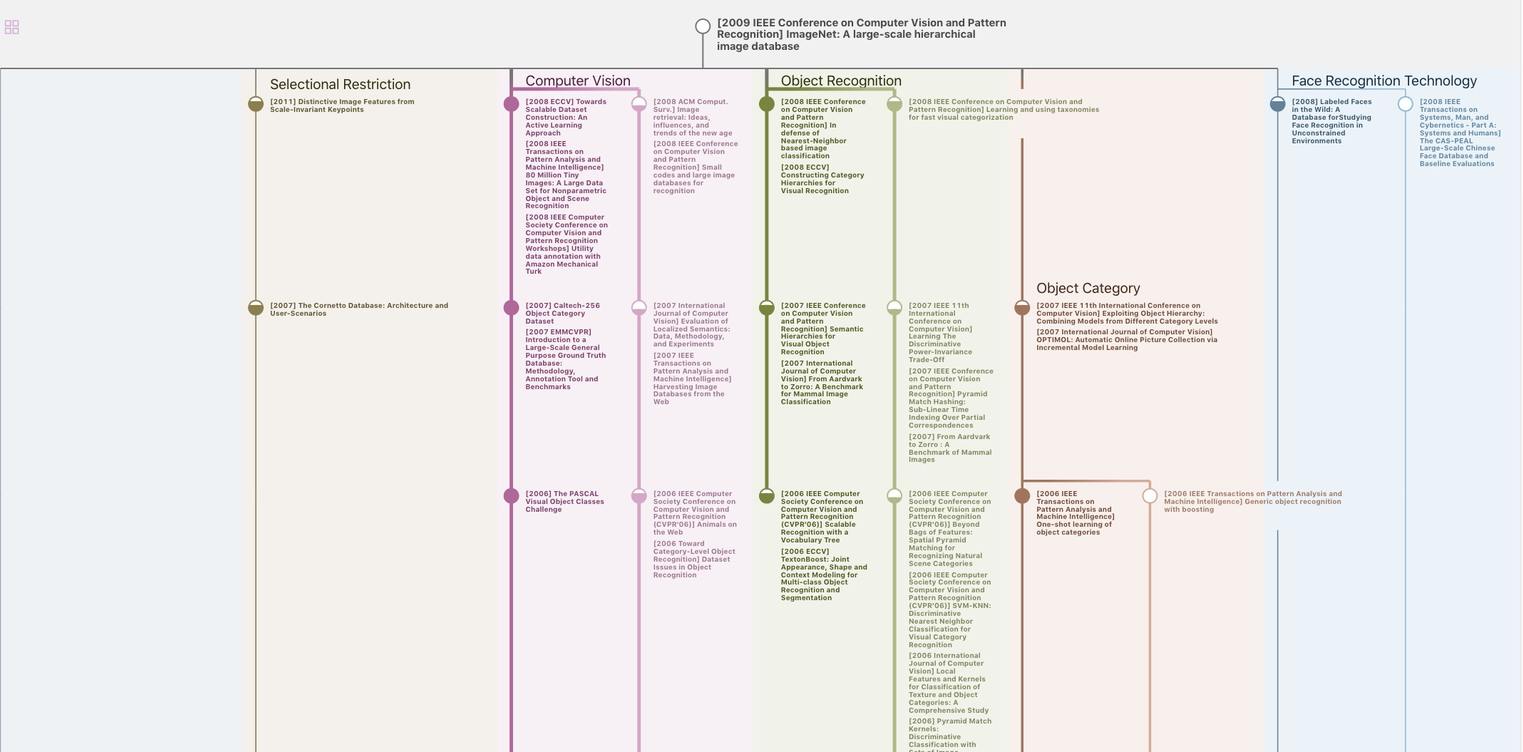
生成溯源树,研究论文发展脉络
Chat Paper
正在生成论文摘要