Machine Learning and Systems Biology Approaches to Characterize Dosage-Based Gene Dependencies in Cancer Cells.
Journal of bioinformatics and systems biology : Open access(2021)
摘要
Mapping of cancer survivability factors allows for the identification of novel biological insights for drug targeting. Using genomic editing techniques, gene dependencies can be extracted in a high-throughput and quantitative manner. Dependencies have been predicted using machine learning techniques on -omics data, but the biological consequences of dependency predictor pairs has not been explored. In this work we devised a framework to explore gene dependency using an ensemble of machine learning methods, and our learned models captured meaningful biological information beyond just gene dependency prediction. We show that dosage-based dependent predictors (DDPs) primarily belonged to transcriptional regulation ontologies. We also found that anti-sense RNAs and long- noncoding RNA transcripts display DDPs. Network analyses revealed that SOX10, HLA-J, and ZEB2 act as a triad of network hubs in the dependent-predictor network. Collectively, we demonstrate the powerful combination of machine learning and systems biology approach can illuminate new insights in understanding gene dependency and guide novel targeting avenues.
更多查看译文
关键词
Gene dependencies,Machine learning
AI 理解论文
溯源树
样例
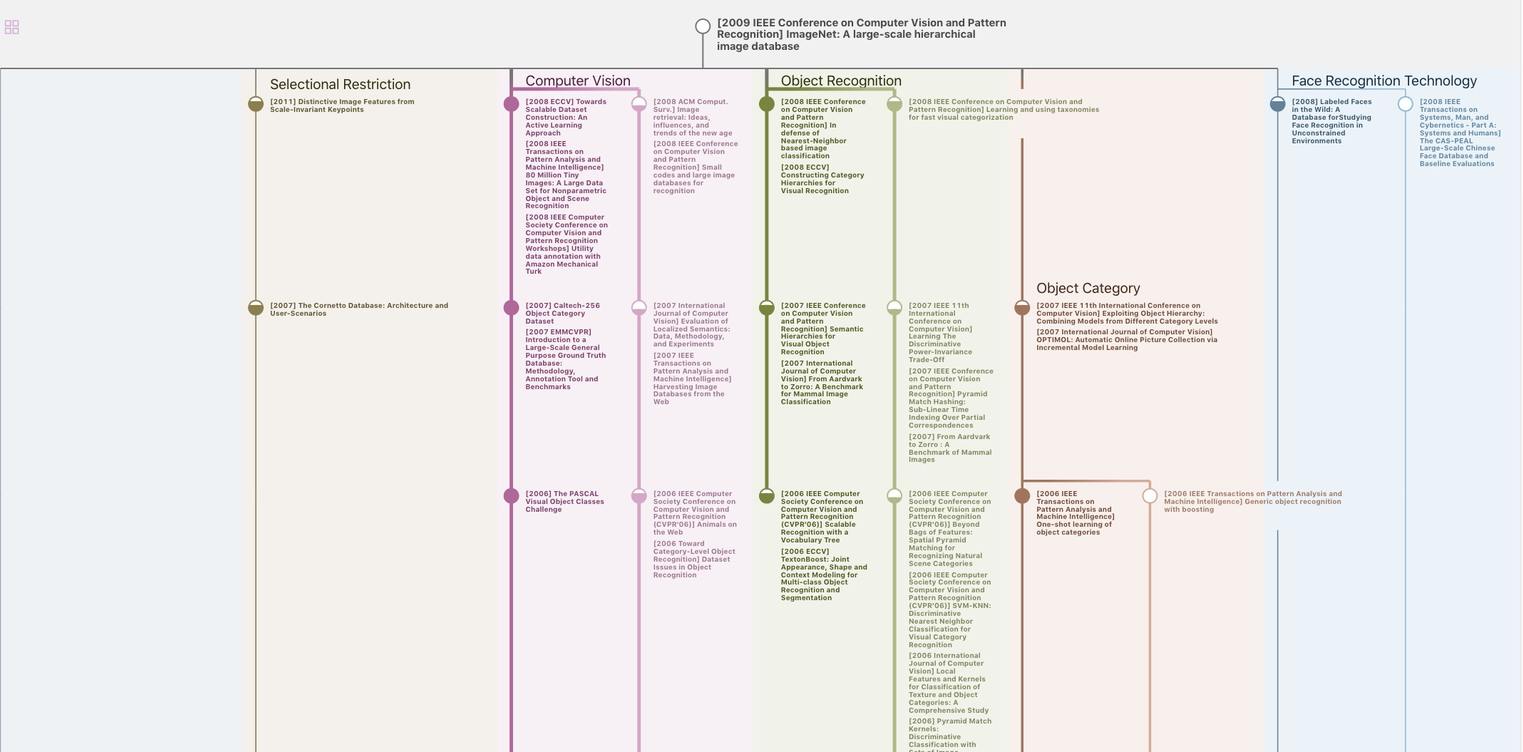
生成溯源树,研究论文发展脉络
Chat Paper
正在生成论文摘要