Bayesian exponential random graph models for populations of networks
arxiv(2021)
摘要
The collection of data on populations of networks is becoming increasingly common, where each data point can be seen as a realisation of a network-valued random variable. A canonical example is that of brain networks: a typical neuroimaging study collects one or more brain scans across multiple individuals, each of which can be modelled as a network with nodes corresponding to distinct brain regions and edges corresponding to structural or functional connections between these regions. Most statistical network models, however, were originally proposed to describe a single underlying relational structure, although recent years have seen a drive to extend these models to populations of networks. Here, we propose one such extension: a multilevel framework for populations of networks based on exponential random graph models. By pooling information across the individual networks, this framework provides a principled approach to characterise the relational structure for an entire population. To perform inference, we devise a novel exchange-within-Gibbs MCMC algorithm that generates samples from the doubly-intractable posterior. To illustrate our framework, we use it to assess group-level variations in networks derived from fMRI scans, enabling the inference of age-related differences in the topological structure of the brain's functional connectivity.
更多查看译文
关键词
random graph models,bayesian exponential,networks,populations
AI 理解论文
溯源树
样例
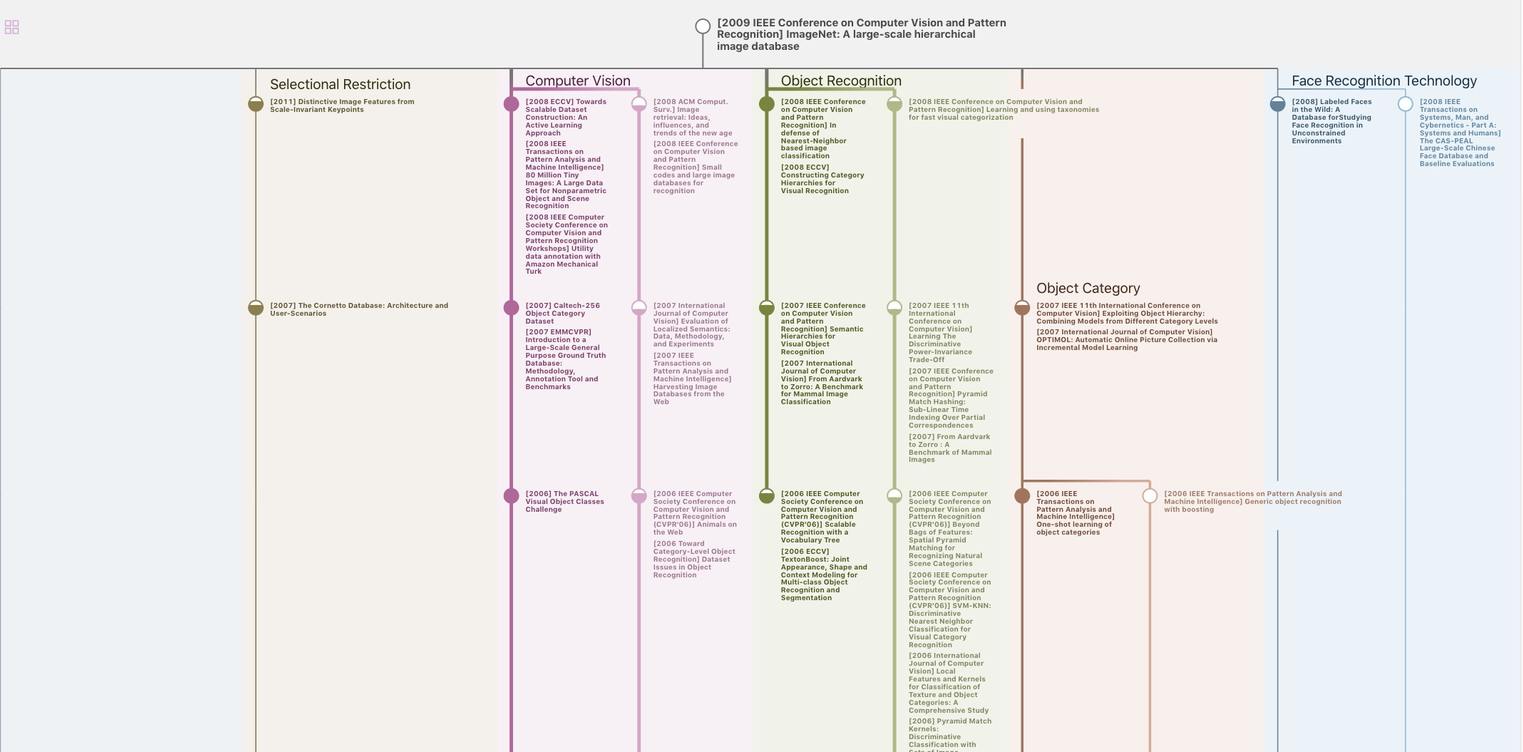
生成溯源树,研究论文发展脉络
Chat Paper
正在生成论文摘要