Deep Transformer Networks for Time Series Classification: The NPP Safety Case
arXiv (Cornell University)(2021)
摘要
A challenging part of dynamic probabilistic risk assessment for nuclear power plants is the need for large amounts of temporal simulations given various initiating events and branching conditions from which representative feature extraction becomes complicated for subsequent applications. Artificial Intelligence techniques have been shown to be powerful tools in time-dependent sequential data processing to automatically extract and yield complex features from large data. An advanced temporal neural network referred to as the Transformer is used within a supervised learning fashion to model the time-dependent NPP simulation data and to infer whether a given sequence of events leads to core damage or not. The training and testing datasets for the Transformer are obtained by running 10,000 RELAP5-3D NPP blackout simulations with the list of variables obtained from the RAVEN software. Each simulation is classified as "OK" or "CORE DAMAGE" based on the consequence. The results show that the Transformer can learn the characteristics of the sequential data and yield promising performance with approximately 99% classification accuracy on the testing dataset.
更多查看译文
关键词
time series classification,time series,npp safety
AI 理解论文
溯源树
样例
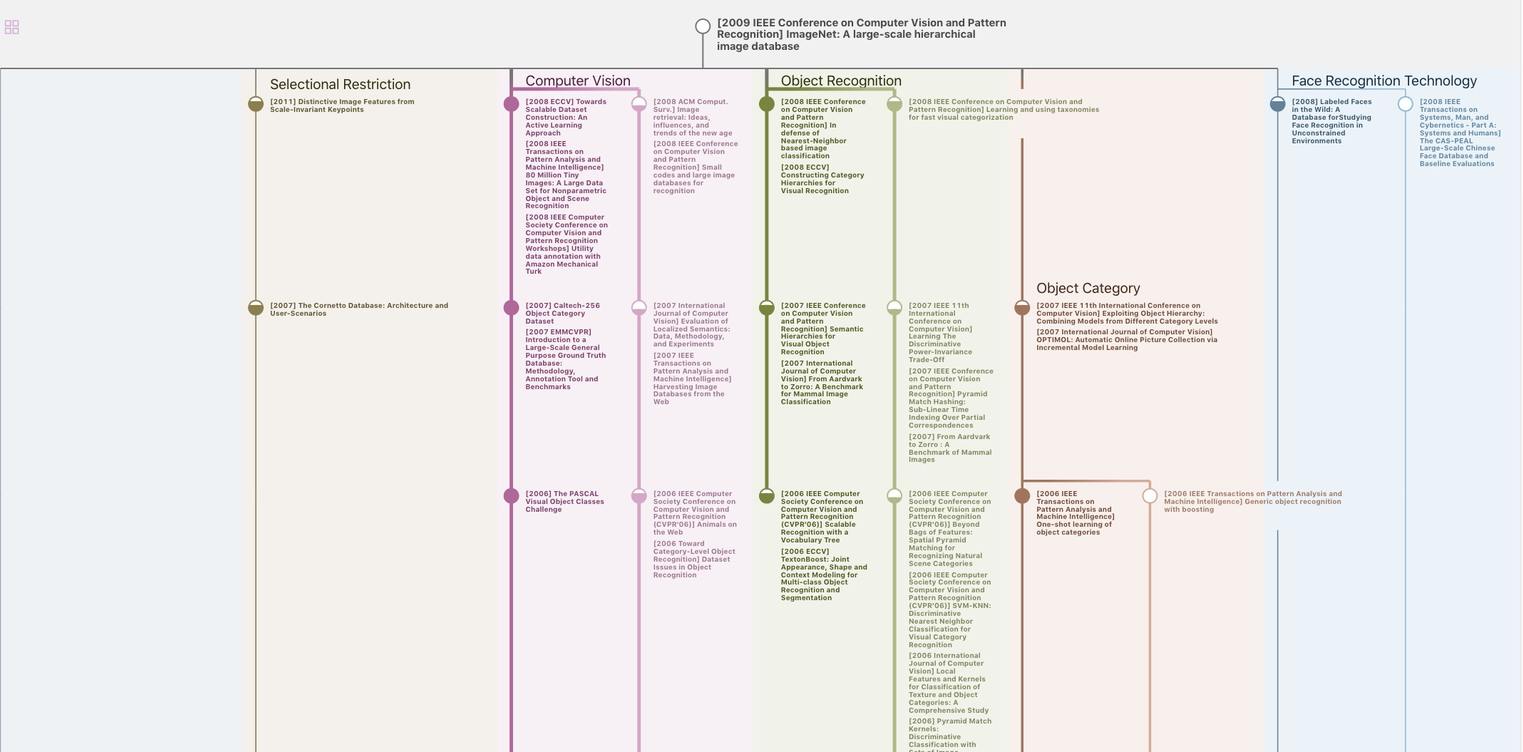
生成溯源树,研究论文发展脉络
Chat Paper
正在生成论文摘要