Extremely Low Footprint End-to-End ASR System for Smart Device.
Interspeech(2021)
摘要
Recently, end-to-end (E2E) speech recognition has become popular, since it can integrate the acoustic, pronunciation and language models into a single neural network, as well as outperforms conventional models. Among E2E approaches, attention-based models, $e.g.$ Transformer, have emerged as being superior. The E2E models have opened the door of deployment of ASR on smart device, however it still suffers from large amount model parameters. This work proposes an extremely low footprint E2E ASR system for smart device, to achieve the goal of satisfying resource constraints without sacrificing recognition accuracy. We adopt cross-layer weight sharing to improve parameter-efficiency. We further exploit the model compression methods including sparsification and quantization, to reduce the memory storage and boost the decoding efficiency on smart device. We have evaluated our approach on the public AISHELL-1 and AISHELL-2 benchmarks. On the AISHELL-2 task, the proposed method achieves more than 10x compression (model size from 248MB to 24MB) while shuffer from small performance loss (CER from 6.49% to 6.92%).
更多查看译文
关键词
speech recognition,san-m,weight sharing,sparsification,quantization
AI 理解论文
溯源树
样例
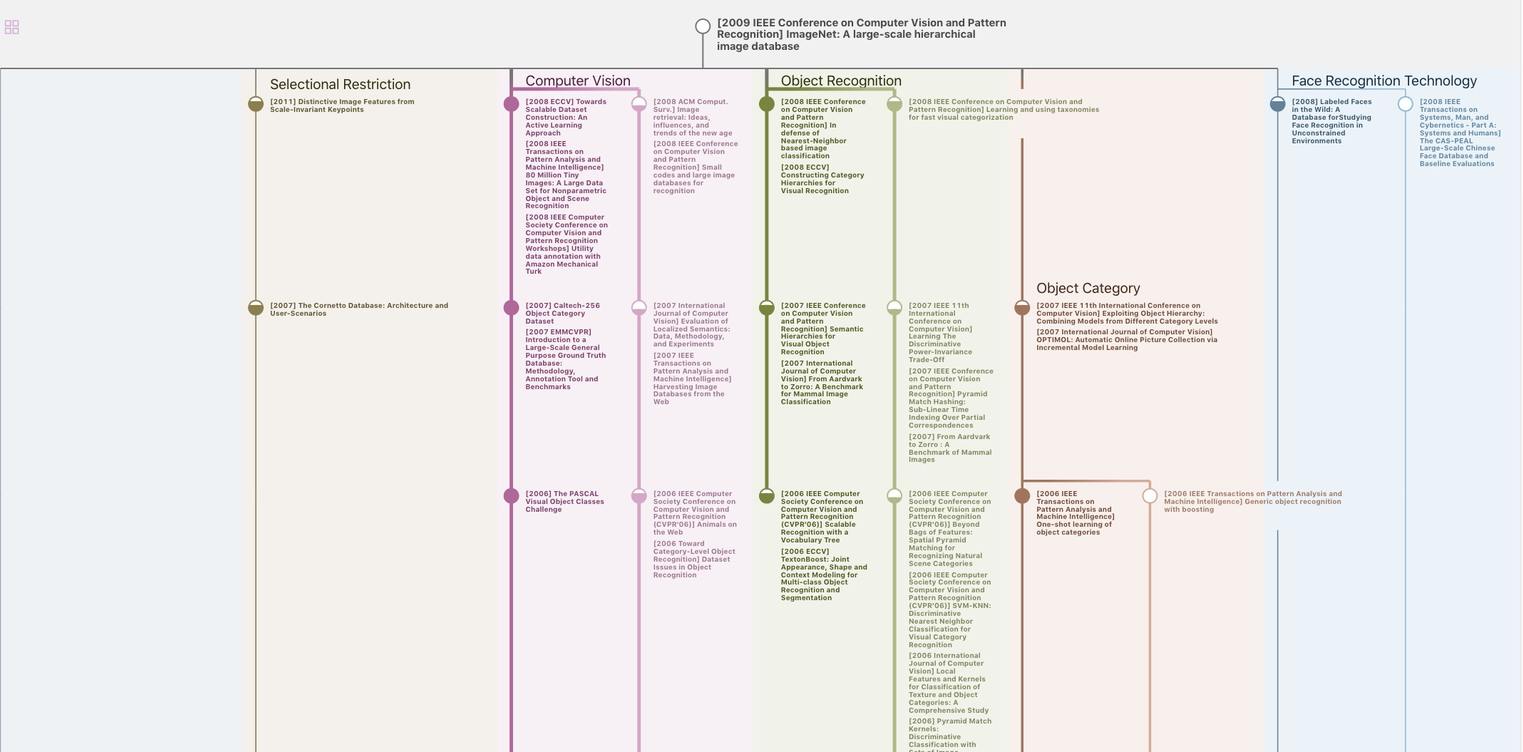
生成溯源树,研究论文发展脉络
Chat Paper
正在生成论文摘要