Numerical solution and bifurcation analysis of nonlinear partial differential equations with extreme learning machines
JOURNAL OF SCIENTIFIC COMPUTING(2021)
摘要
We address a new numerical method based on a class of machine learning methods, the so-called Extreme Learning Machines (ELM) with both sigmoidal and radial-basis functions, for the computation of steady-state solutions and the construction of (one-dimensional) bifurcation diagrams of nonlinear partial differential equations (PDEs). For our illustrations, we considered two benchmark problems, namely (a) the one-dimensional viscous Burgers with both homogeneous (Dirichlet) and non-homogeneous boundary conditions, and, (b) the one- and two-dimensional Liouville–Bratu–Gelfand PDEs with homogeneous Dirichlet boundary conditions. For the one-dimensional Burgers and Bratu PDEs, exact analytical solutions are available and used for comparison purposes against the numerical derived solutions. Furthermore, the numerical efficiency (in terms of numerical accuracy, size of the grid and execution times) of the proposed numerical machine-learning method is compared against central finite differences (FD) and Galerkin weighted-residuals finite-element (FEM) methods. We show that the proposed numerical machine learning method outperforms in terms of numerical accuracy both FD and FEM methods for medium to large sized grids, while provides equivalent results with the FEM for low to medium sized grids; both methods (ELM and FEM) outperform the FD scheme. Furthermore, the computational times required with the proposed machine learning scheme were comparable and in particular slightly smaller than the ones required with FEM.
更多查看译文
关键词
Numerical analysis, Nonlinear partial differential equations, Numerical bifurcation analysis, Machine learning, Extreme learning machines
AI 理解论文
溯源树
样例
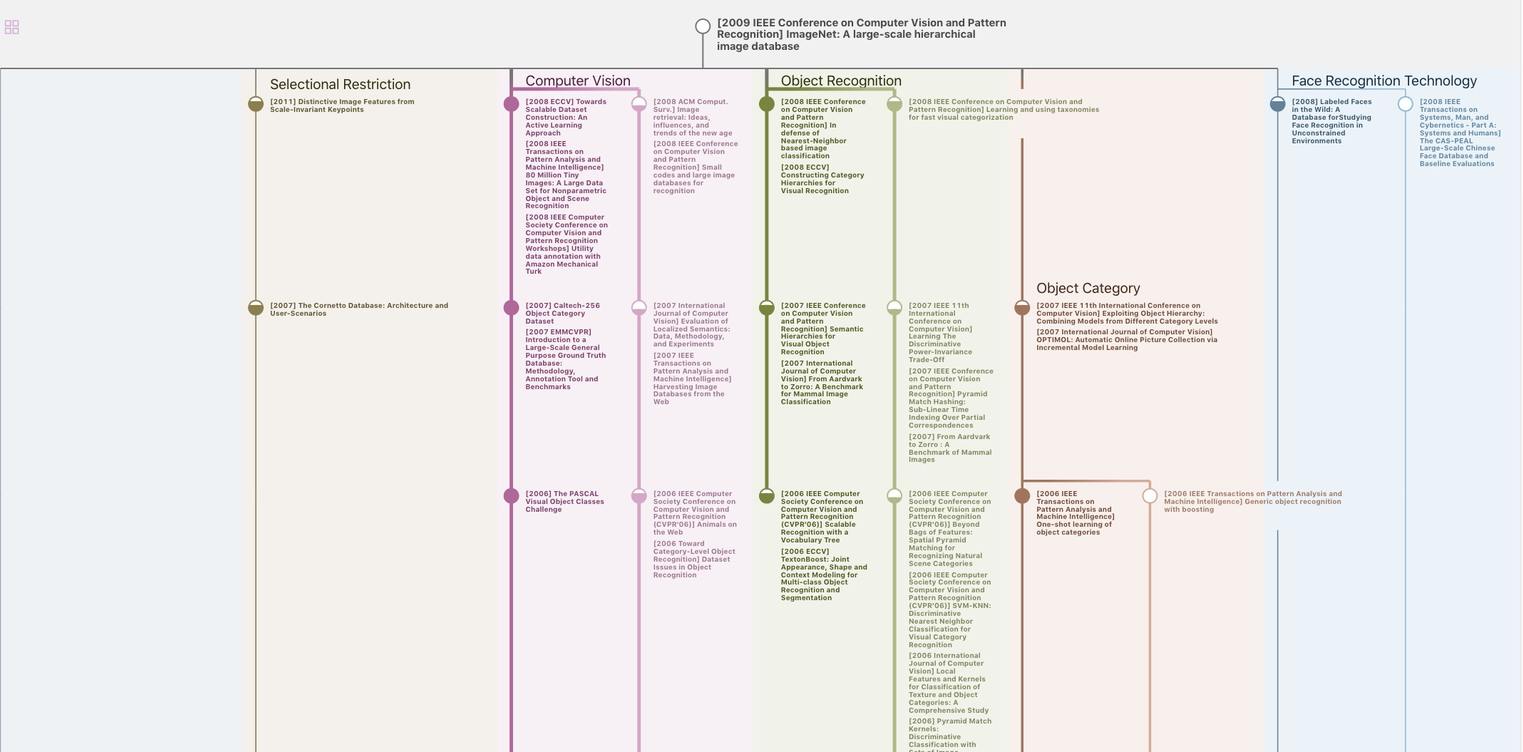
生成溯源树,研究论文发展脉络
Chat Paper
正在生成论文摘要