Estimating Levels of Engagement for Social Human-Robot Interaction using Legendre Memory Units
ACM/IEEE International Conference on Human-Robot Interaction(2021)
摘要
ABSTRACTIn this study, we examine whether the data requirements associated with training a system to recognize multiple 'levels' of an internal state can be reduced by training systems on the 'extremes' in a way that allows them to estimate "intermediate" classes as falling in-between the trained extremes. Specifically, this study explores whether a novel recurrent neural network, the Legendre Delay Network, added as a pre-processing step to a Multi-Layer Perception, produces an output which can be used to separate an untrained intermediate class of task engagement from the trained extreme classes. The results showed that identifying untrained classes after training on the extremes is feasible, particularly when using the Legendre Delay Network.
更多查看译文
关键词
Task Engagement, Intensity, Legendre Memory Units, MLP, Activity Recognition
AI 理解论文
溯源树
样例
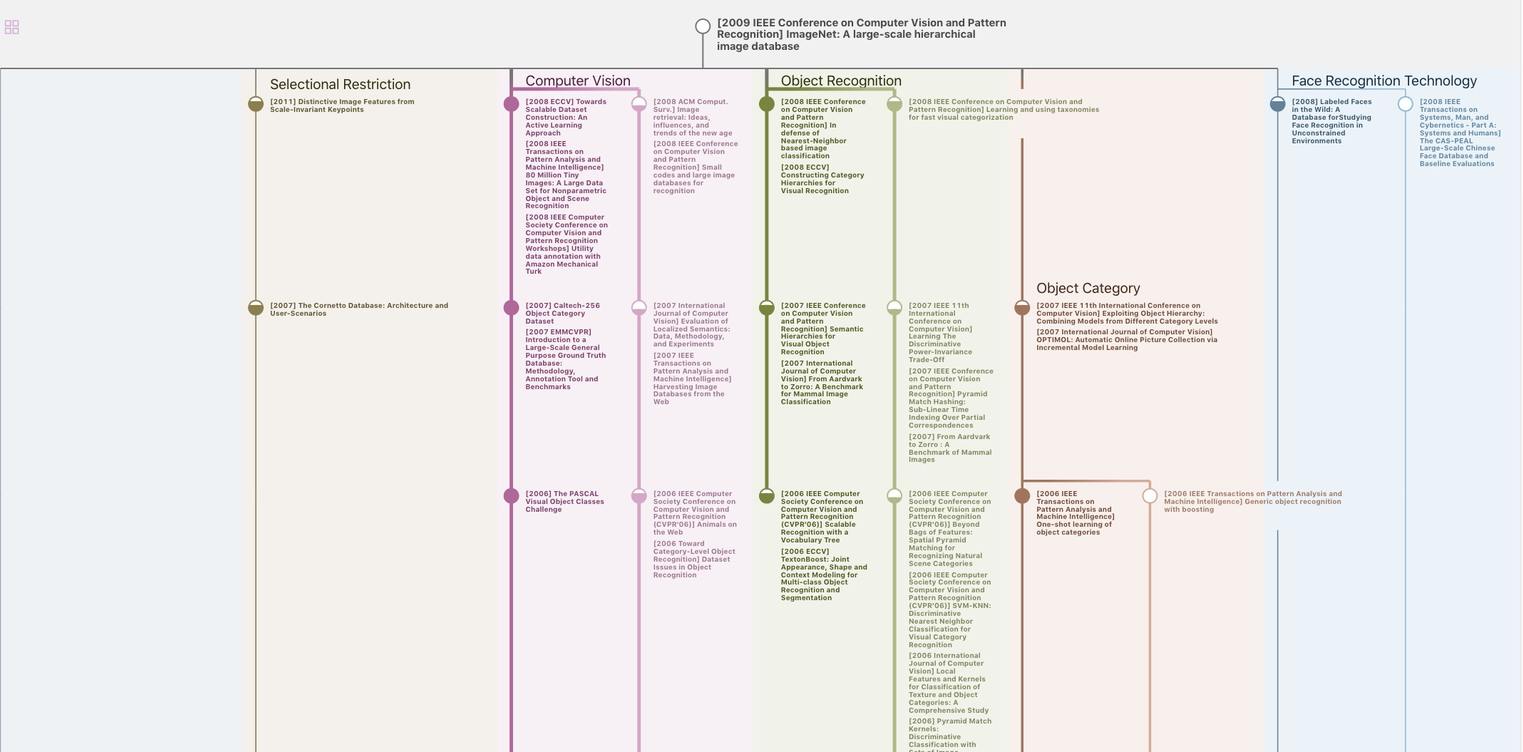
生成溯源树,研究论文发展脉络
Chat Paper
正在生成论文摘要