When Oracles Go Wrong: Using Preferences as a Means to Explore
HRI(2021)
摘要
ABSTRACTWhen a robot is deployed to learn a new task in a "real-word" environment, there may be multiple teachers and therefore multiple sources of feedback. Furthermore, there may be multiple optimal solutions for a given task and teachers may have preferences among those various solutions. We present an Interactive Reinforcement Learning (I-RL) algorithm, Multi-Teacher Activated Policy Shaping (M-TAPS), which addresses the problem of learning from multiple teachers and leverages differences between them as a means to explore the environment. We show that this algorithm can significantly increase an agent's robustness to the environment and quickly adopt to a teacher's preferences. Finally, we present a formal model for comparing human teachers and constructed oracle teachers and the way that they provide feedback to a robot.
更多查看译文
关键词
human-robot interaction, interactive reinforcement learning
AI 理解论文
溯源树
样例
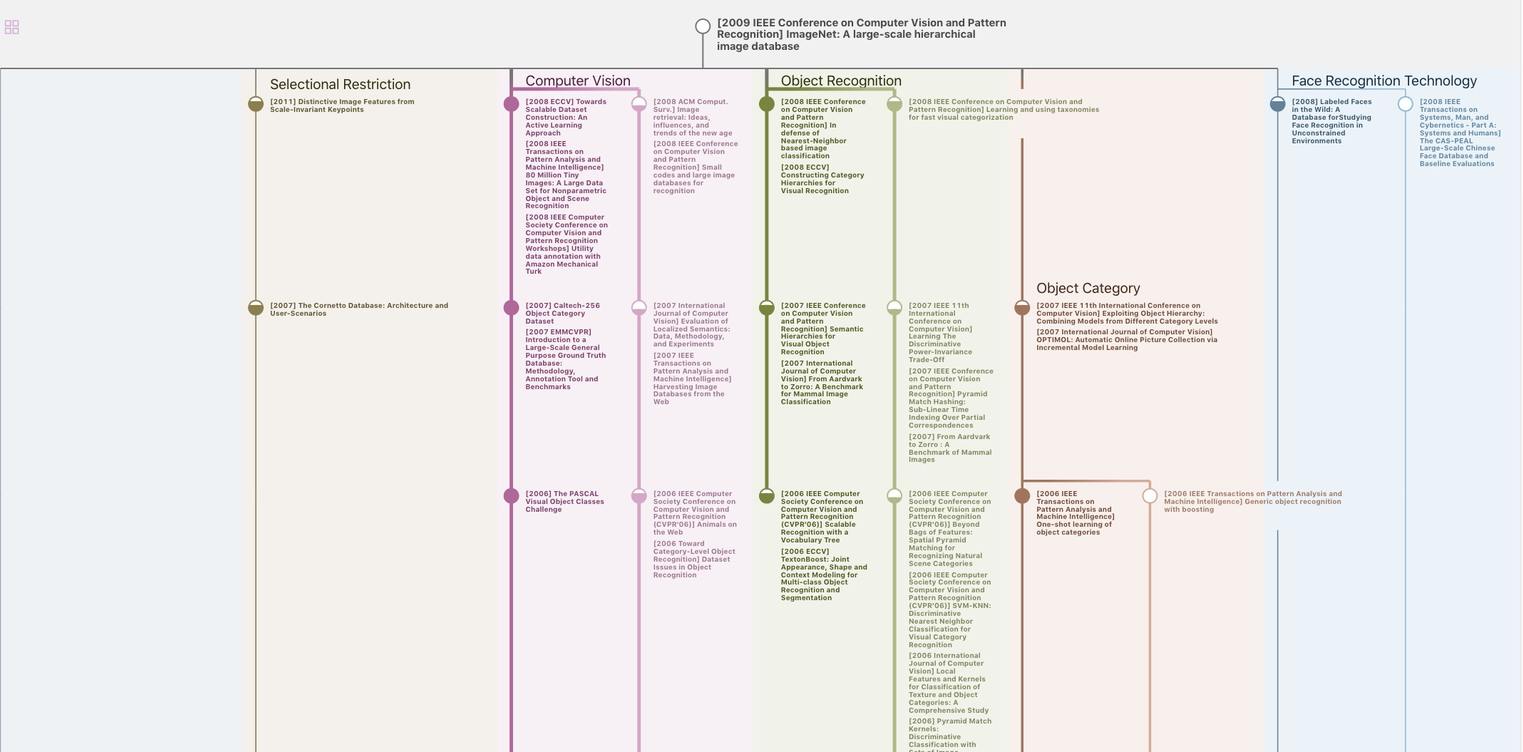
生成溯源树,研究论文发展脉络
Chat Paper
正在生成论文摘要