Measuring Students' Self-Regulatory Phases in LMS with Behavior and Real-Time Self Report.
LAK(2021)
摘要
Research has emphasized that self-regulated learning (SRL) is critically important for learning. However, students have different capabilities of regulating their learning processes and individual needs. To help students improve their SRL capabilities, we need to identify students' current behaviors. Specifically, we applied instructional design to create visible and meaningful markers of student learning at different points in time in LMS logs. We adopted knowledge engineering to develop a framework of proximal indicators representing SRL phases and evaluated them in a quasi-experiment in two different learning activities. A comparison of two sources of collected students' SRL data, self-reported and trace data, revealed a relatively high agreement between our classifications (weighted kappa, k = .74 and k = .68). However, our indicators did not always discriminate adjacent SRL phases, particularly for enactment and adapting phases, compared with students' real-time self-reported behaviors. Our behavioral indicators also were comparably successful at classifying SRL phases for different self-regulatory engagement levels. This study demonstrated how the triangulation of various sources of students' self-regulatory data could help to unravel the complex nature of metacognitive processes.
更多查看译文
关键词
self-regulated learning,pattern recognition,knowledge-engineered trace measures,self-reported measures
AI 理解论文
溯源树
样例
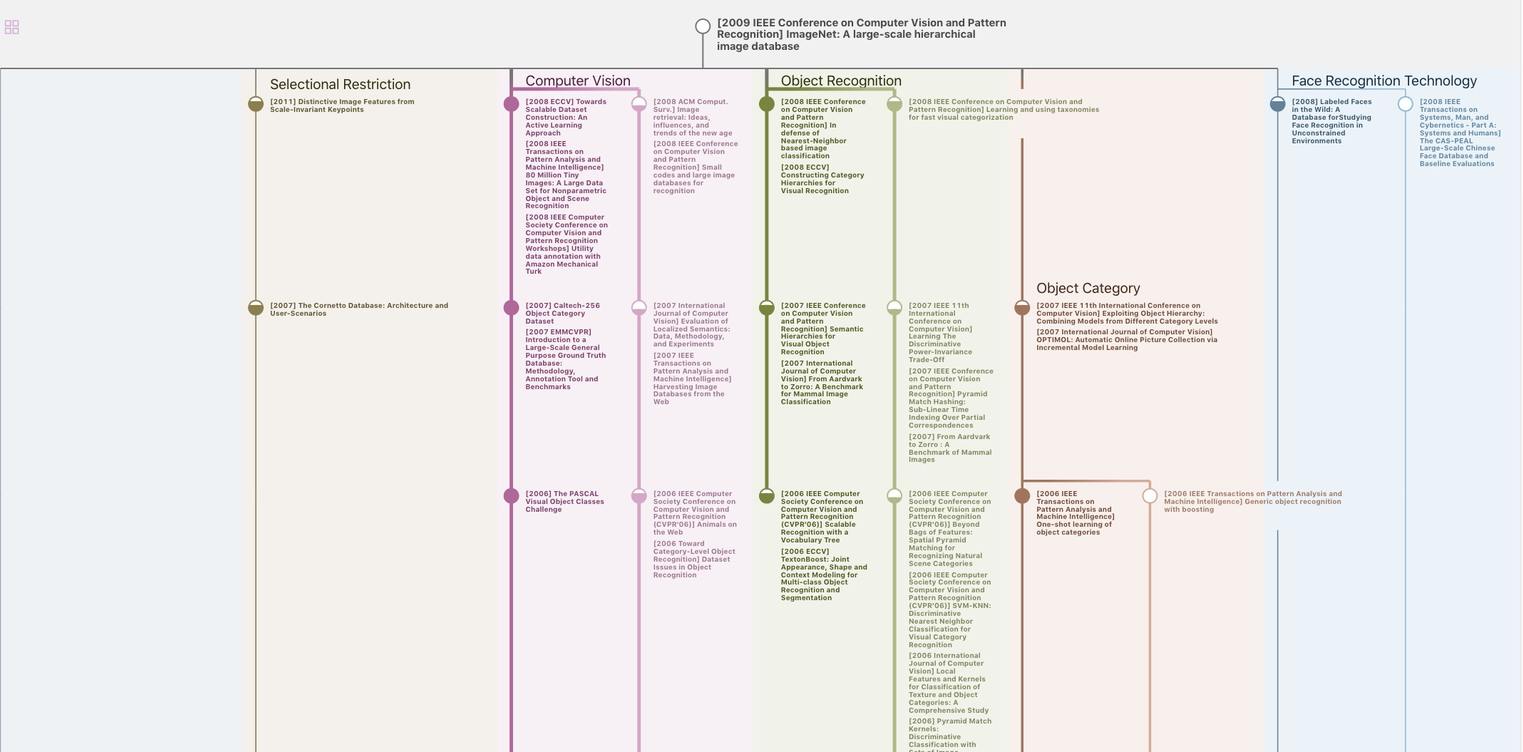
生成溯源树,研究论文发展脉络
Chat Paper
正在生成论文摘要