Quantitative analysis based on chest CT classifies common and severe patients with coronavirus disease 2019 pneumonia in Wuhan, China
Chinese Journal of Academic Radiology(2021)
摘要
Objective This study aimed to compare quantifiable radiologic findings and their dynamic change throughout the clinical course of common and severe coronavirus disease 2019 (COVID-19), and to provide valuable evidence for radiologic classification of the two types of this disease. Methods 112 patients with laboratory-confirmed COVID-19 were retrospectively analyzed. Volumetric percentage of infection and density of the lung were measured by a computer-aided software. Clinical parameters were recorded to reflect disease progression. Baseline data and dynamic change were compared between two groups and a decision-tree algorithm was developed to determine the cut-off value for classification. Results 93 patients were finally included and were divided into common group ( n = 76) and severe group ( n = 17) based on current criteria. Compared with common patients, severe patients experienced shorter advanced stage, peak time and plateau, but longer absorption stage. The dynamic change of volume and density coincided with the clinical course. The interquartile range of volumetric percentage of the two groups were 1.0–7.2% and 11.4–31.2%, respectively. Baseline volumetric percentage of infection was significantly higher in severe group, and the cut-off value of it was 10.10%. Conclusions Volumetric percentage between severe and common patients was significantly different. Because serial CT scans are systemically performed in patients with COVID-19 pneumonia, this quantitative analysis can simultaneously provide valuable information for physicians to evaluate their clinical course and classify common and severe patients accurately.
更多查看译文
关键词
Coronavirus disease 2019, Multidetector computed tomography, Artificial intelligence, Numerical analysis, Computer-assisted, Decision trees
AI 理解论文
溯源树
样例
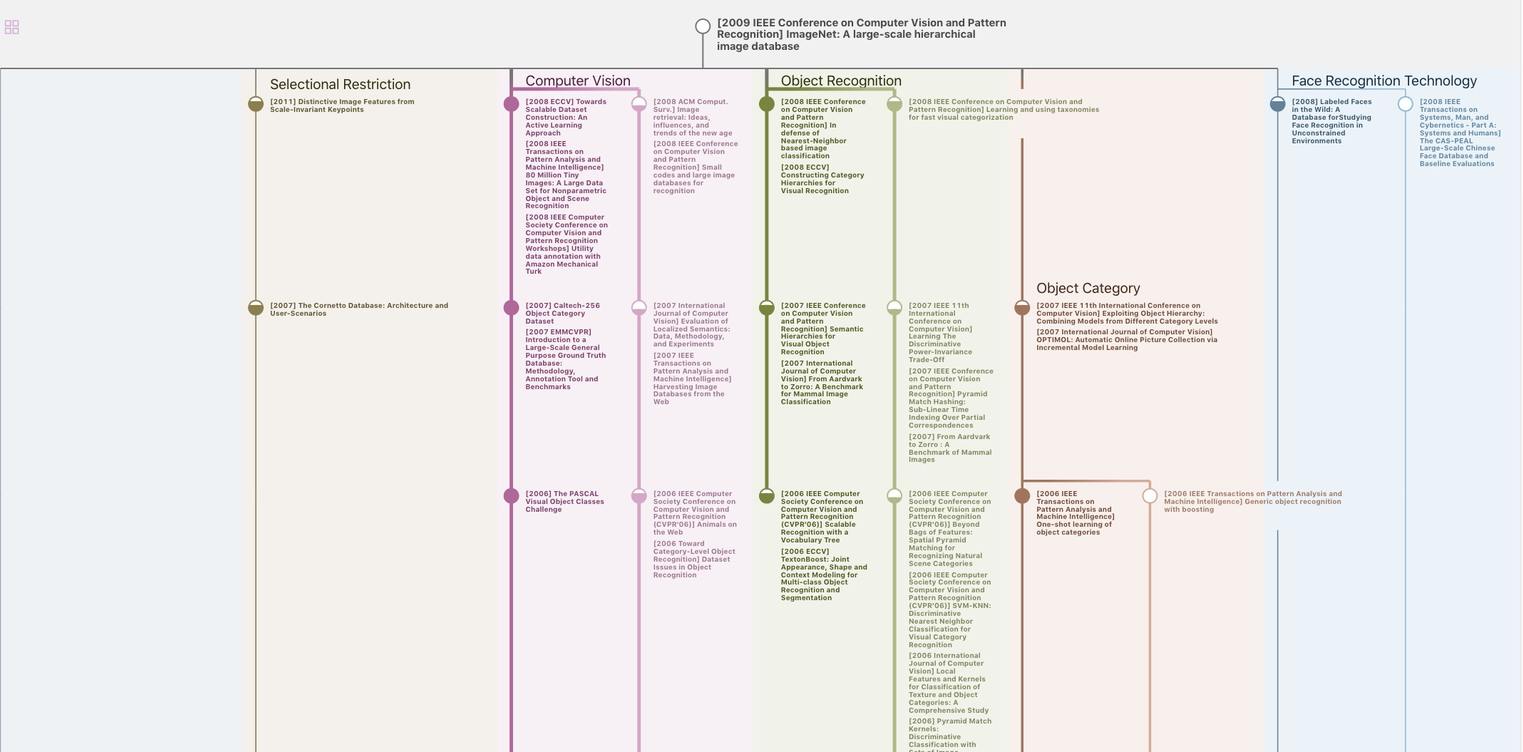
生成溯源树,研究论文发展脉络
Chat Paper
正在生成论文摘要