Stealthy False Data Injection Attack Detection in Smart Grids with Uncertainties: A Deep Transfer Learning Based Approach
arxiv(2021)
摘要
Most traditional false data injection attack (FDIA) detection approaches rely on static system parameters or a single known snapshot of dynamic ones. However, such a setting significantly weakens the practicality of these approaches when facing the fact that the system parameters are dynamic and cannot be accurately known during operation due to the presence of uncertainties in practical smart grids. In this paper, we propose an FDIA detection mechanism from the perspective of transfer learning. Specifically, the known initial/approximate system is treated as a source domain, which provides abundant simulated normal and attack data. The real world's unknown running system is taken as a target domain where sufficient real normal data are collected for tracking the latest system states online. The designed transfer strategy that aims at making full use of data in hand is divided into two optimization stages. In the first stage, a deep neural network (DNN) is built by simultaneously optimizing several well-designed terms with both simulated data and real data, and then it is fine-tuned via real data in the second stage. Several case studies on the IEEE 14-bus power system verify the effectiveness of the proposed mechanism.
更多查看译文
AI 理解论文
溯源树
样例
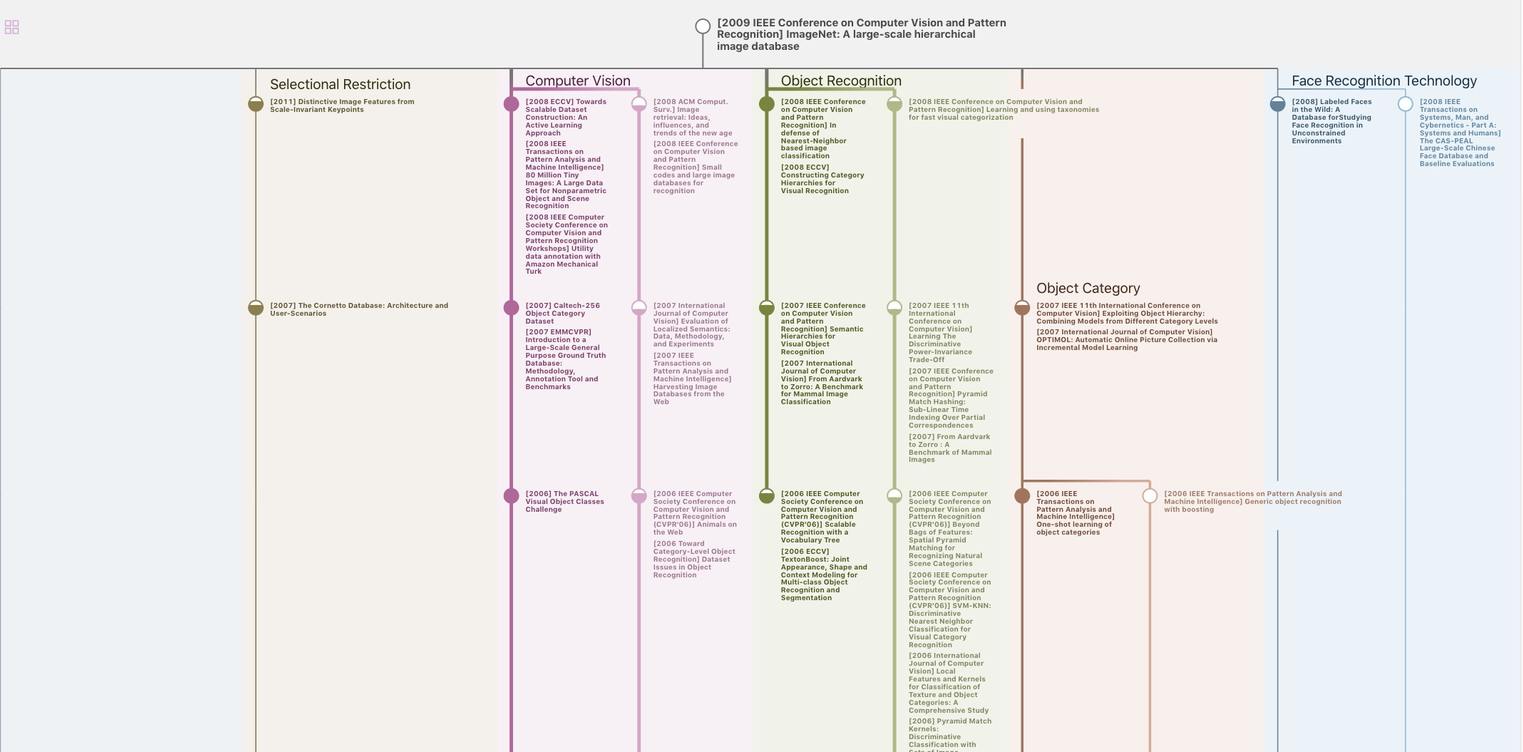
生成溯源树,研究论文发展脉络
Chat Paper
正在生成论文摘要