Online Markov Blanket Discovery With Streaming Features
2020 IEEE International Conference on Knowledge Graph (ICKG)(2020)
摘要
The Markov blanket (MB) in Bayesian networks has attracted much attention since the MB of a target attribute (T) is the minimal feature subset with maximum prediction ability for classification. Nevertheless, traditional MB discovery methods such as IAMB, HITON-MB, and MMMB are not suitable for streaming features. Meanwhile, online feature selection with streaming features (OSFSF) methods such as Alpha-investing and SAOLA, focus only on the relevance and ignores causality of the features, they cannot mine the MB, or only find the parents and children (PC) such as OSFS. Therefore, these methods have weaker interpretability and do not have sufficient prediction accuracy. We propose a novel algorithm for online markov blanket discovery with streaming features to tackle abovementioned issues, named OMBSF. OMBSF finds the MB of T containing parents, children, and spouses, discards false positives from the PC and spouse sets online, distinguishes between the PC and spouse in real-time. An empirical study demonstrates that OMBSF finds a more accurate MB when the volume of features is high and it significantly improves the prediction accuracy than other algorithms. Moreover, OMBSF obtains a bigger feature subset than that obtained by OSFS, demonstrating that OMBSF can identify the spouse in the MB that are not identified using OSFS.
更多查看译文
关键词
markov blanket,Bayesian network,streaming feature
AI 理解论文
溯源树
样例
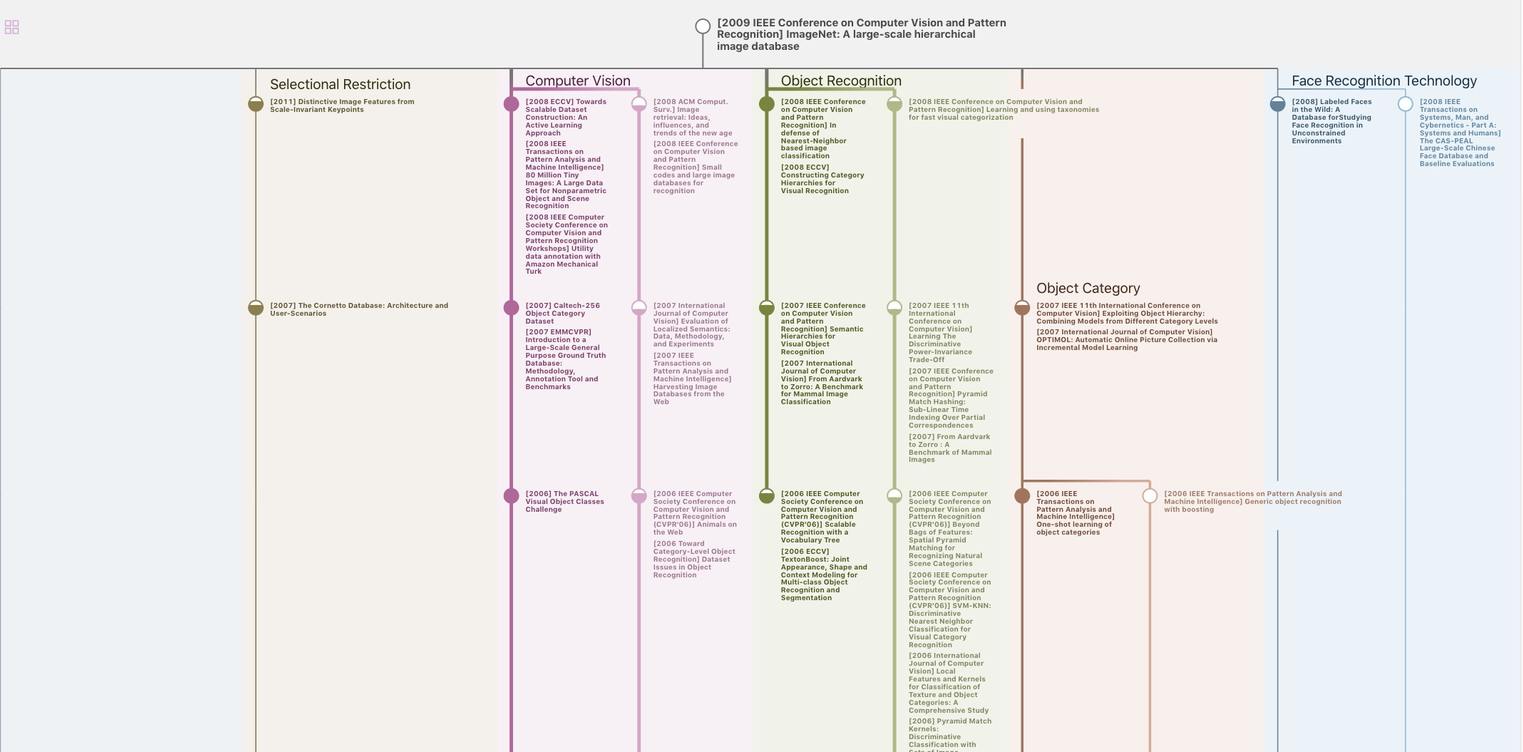
生成溯源树,研究论文发展脉络
Chat Paper
正在生成论文摘要