Tensor Dropout for Robust Learning
IEEE Journal of Selected Topics in Signal Processing(2021)
摘要
CNNs achieve high levels of performance by leveraging deep, over-parametrized neural architectures, trained on large datasets. However, they exhibit limited generalization abilities outside their training domain and lack robustness to corruptions such as noise and adversarial attacks. To improve robustness and obtain more computationally and memory efficient models, better inductive biases are needed. To provide such inductive biases, tensor layers have been successfully proposed to leverage multi-linear structure through higher-order computations. In this paper, we propose tensor dropout, a randomization technique that can be applied to tensor factorizations, such as those parametrizing tensor layers. In particular, we study tensor regression layers, parametrized by low-rank weight tensors and augmented with our proposed tensor dropout. We empirically show that our approach improves generalization for image classification on ImageNet and CIFAR-100. We also establish state-of-the-art accuracy for phenotypic trait prediction on the largest available dataset of brain MRI (U.K. Biobank), where multi-linear structure is paramount. In all cases, we demonstrate superior performance and significantly improved robustness, both to noisy inputs and to adversarial attacks. We establish the theoretical validity of our approach and the regularizing effect of tensor dropout by demonstrating the link between randomized tensor regression with tensor dropout and deterministic regularized tensor regression.
更多查看译文
关键词
Deep learning,randomized tensor regression,robustness,stochastic regularization,tensor dropout,tensor methods,tensor regression,tensor regression layers
AI 理解论文
溯源树
样例
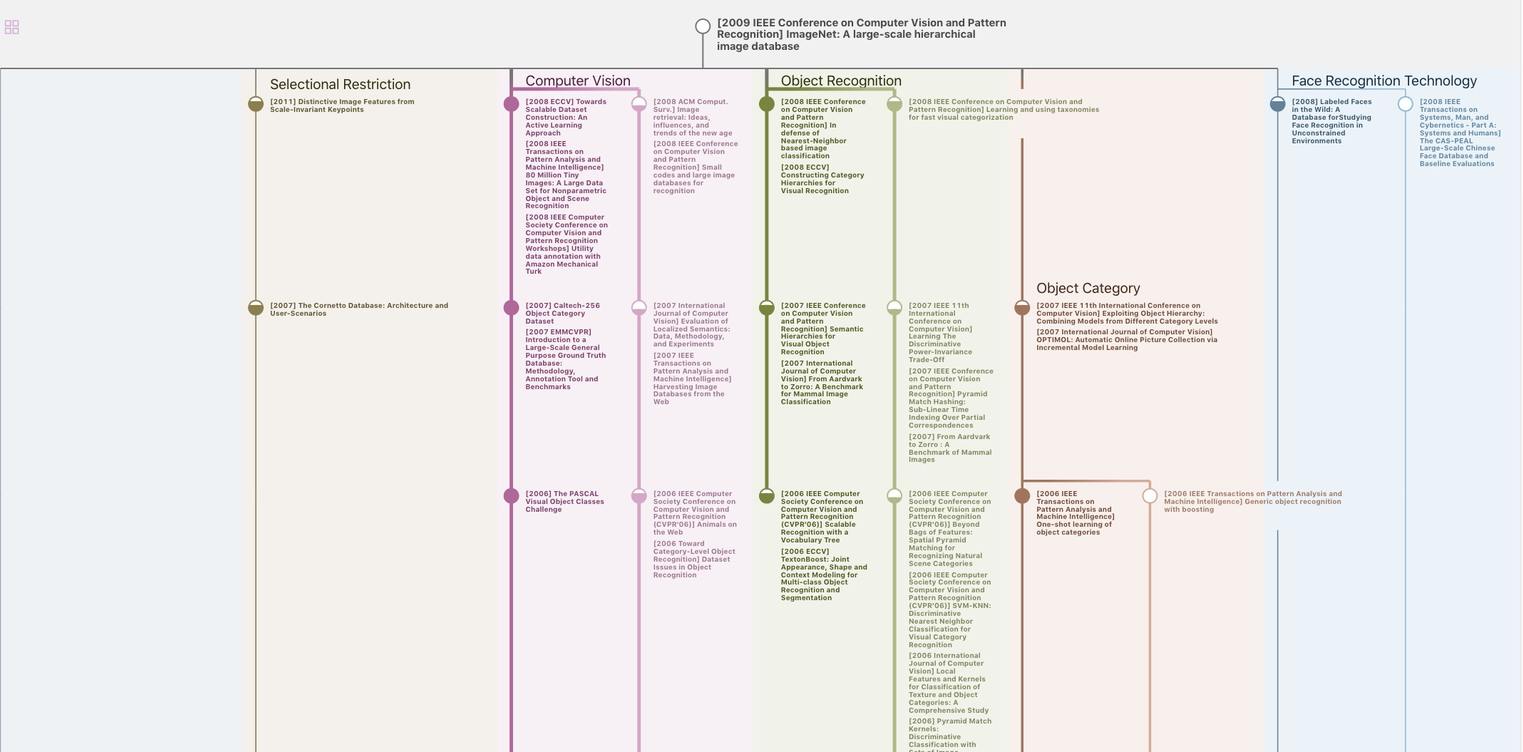
生成溯源树,研究论文发展脉络
Chat Paper
正在生成论文摘要