Semi-supervised Learning Framework for UAV Detection
2021 IEEE 32ND ANNUAL INTERNATIONAL SYMPOSIUM ON PERSONAL, INDOOR AND MOBILE RADIO COMMUNICATIONS (PIMRC)(2021)
摘要
The use of supervised learning with various sensing techniques such as audio, visual imaging, thermal sensing, RADAR, and radio frequency (RF) have been widely applied in the detection of unmanned aerial vehicles (UAV) in an environment. However, little or no attention has been given to the application of unsupervised or semi-supervised algorithms for UAV detection. In this paper, we propose a semi-supervised technique and architecture for detecting UAVs in an environment by exploiting the RF signals (i.e., fingerprints) between a UAV and its flight-controller communication under wireless inference such as Bluetooth and WiFi. By decomposing the RF signals using a two-level wavelet packet transform, we estimated the second moment statistic (i.e., variance) of the coefficients in each packet as a feature set. We developed a local outlier factor model as the UAV detection algorithm using the coefficient variances of the wavelet packets from WiFi and Bluetooth signals. When detecting the presence of RF-based UAV, we achieved an accuracy of 96.7% and 86% at a signal-to-noise ratio of 30 dB and 18 dB, respectively. The application of this approach is not limited to UAV detection as it can be extended to the detection of rogue RF devices in an environment.
更多查看译文
关键词
local outlier factor, wavelet packet transform, UAV, RF fingerprinting, detection
AI 理解论文
溯源树
样例
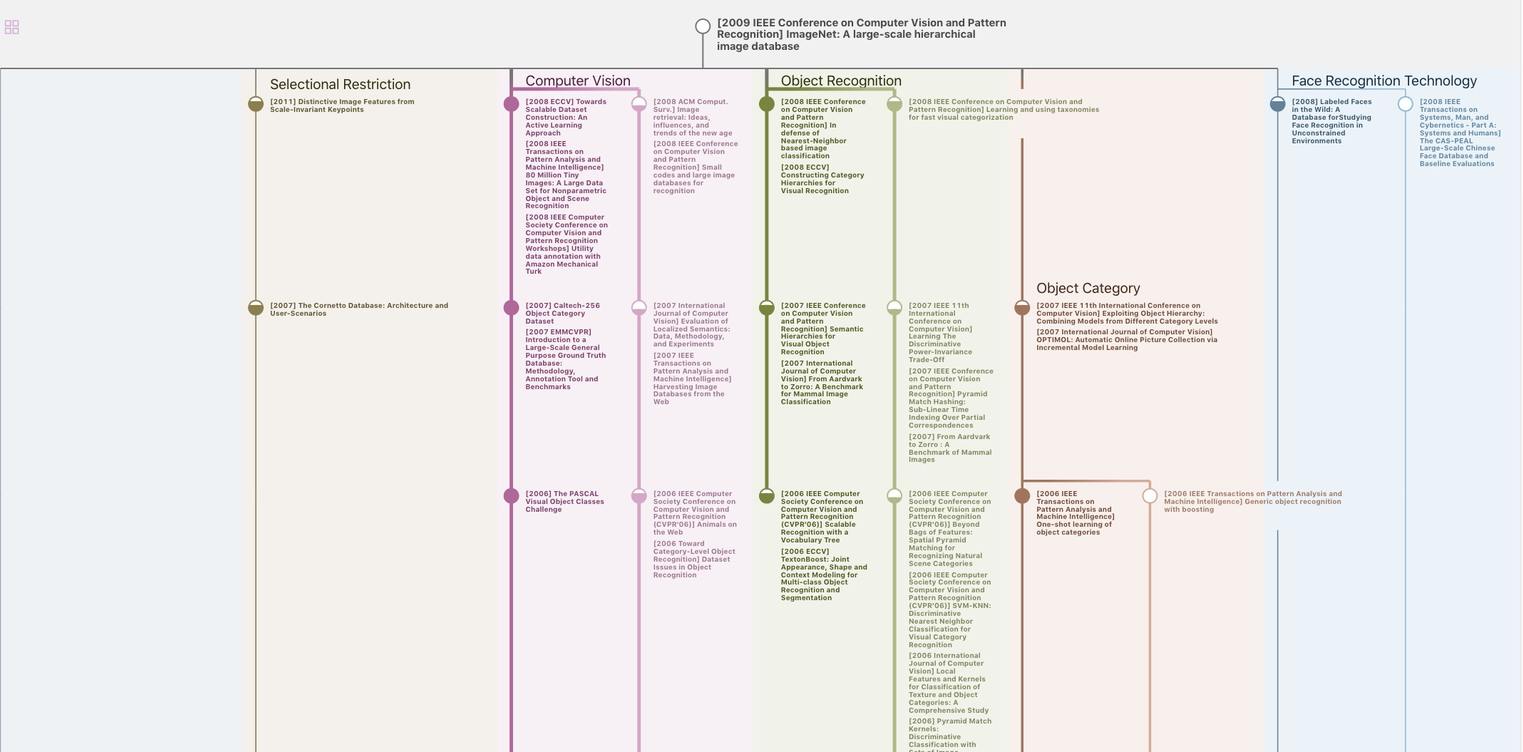
生成溯源树,研究论文发展脉络
Chat Paper
正在生成论文摘要