Reducing Negative Impact of Noise in Boolean Matrix Factorization with Association Rules
ADVANCES IN INTELLIGENT DATA ANALYSIS XIX, IDA 2021(2021)
摘要
Boolean matrix factorization (BMF) is a well-established data analytical method whose goal is to decompose a single large matrix into two, preferably smaller, matrices, carrying the same or similar information as the original matrix. In essence, it can be used to reduce data dimensionality and to provide fundamental insight into data. Existing algorithms are often negatively affected by the presence of noise in the data, which is a common case for real-world datasets. We present an initial study on an algorithm for approximate BMF that uses association rules in a novel way to identify possible noise. This allows us to suppress the impact of noise and improve the quality of results. Moreover, we show that association rules provide a suitable framework allowing the handling of noise in BMF in a justified way.
更多查看译文
关键词
Boolean matrix factorization, Formal concept analysis, Association rules, Noise reduction
AI 理解论文
溯源树
样例
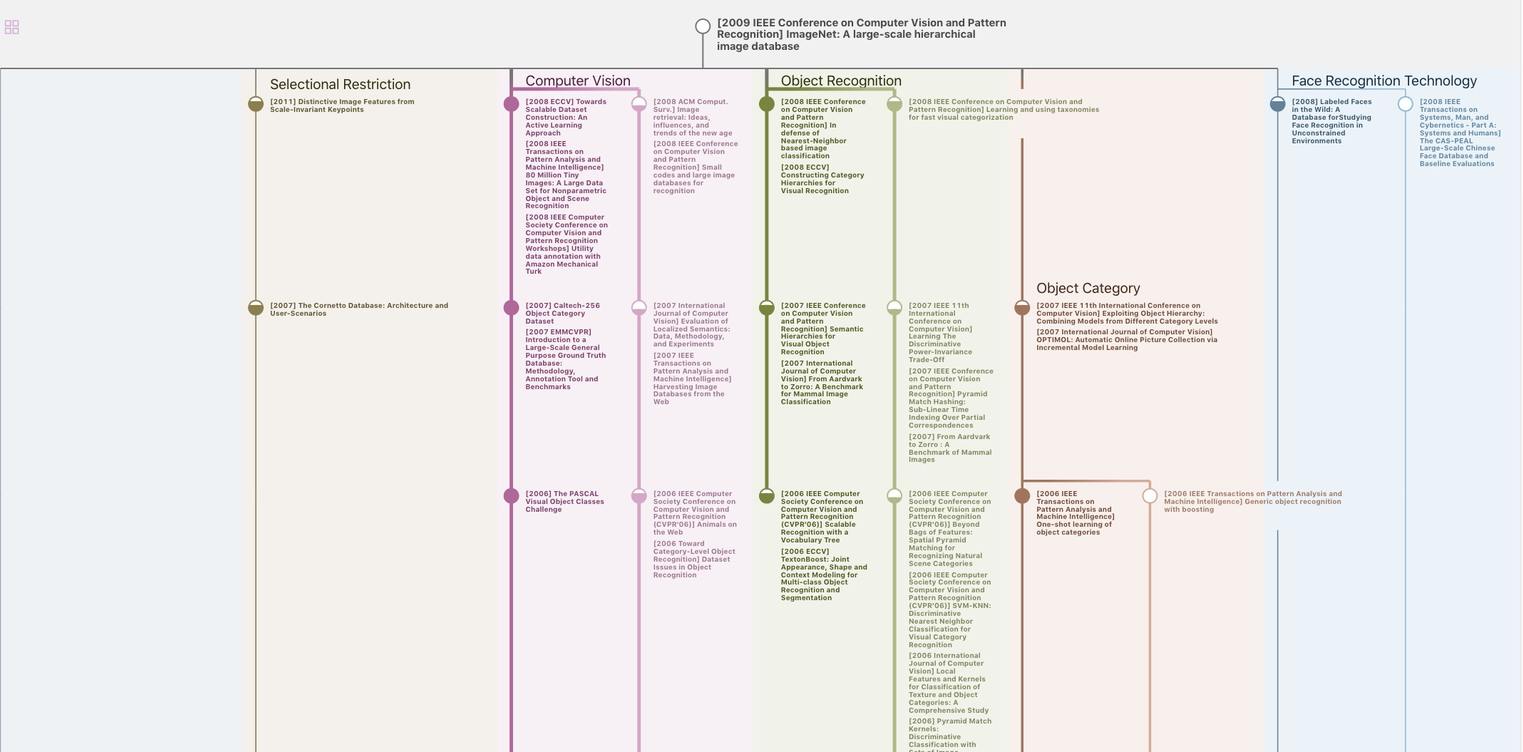
生成溯源树,研究论文发展脉络
Chat Paper
正在生成论文摘要