A Fast Multi-Locus Ridge Regression Algorithm For High-Dimensional Genome-Wide Association Studies
FRONTIERS IN GENETICS(2021)
摘要
The mixed linear model (MLM) has been widely used in genome-wide association study (GWAS) to dissect quantitative traits in human, animal, and plant genetics. Most methodologies consider all single nucleotide polymorphism (SNP) effects as random effects under the MLM framework, which fail to detect the joint minor effect of multiple genetic markers on a trait. Therefore, polygenes with minor effects remain largely unexplored in today's big data era. In this study, we developed a new algorithm under the MLM framework, which is called the fast multi-locus ridge regression (FastRR) algorithm. The FastRR algorithm first whitens the covariance matrix of the polygenic matrix K and environmental noise, then selects potentially related SNPs among large scale markers, which have a high correlation with the target trait, and finally analyzes the subset variables using a multi-locus deshrinking ridge regression for true quantitative trait nucleotide (QTN) detection. Results from the analyses of both simulated and real data show that the FastRR algorithm is more powerful for both large and small QTN detection, more accurate in QTN effect estimation, and has more stable results under various polygenic backgrounds. Moreover, compared with existing methods, the FastRR algorithm has the advantage of high computing speed. In conclusion, the FastRR algorithm provides an alternative algorithm for multi-locus GWAS in high dimensional genomic datasets.
更多查看译文
关键词
genome-wide association study, mixed linear model, multi-locus algorithm, statistical power, polygenic background, minor effect
AI 理解论文
溯源树
样例
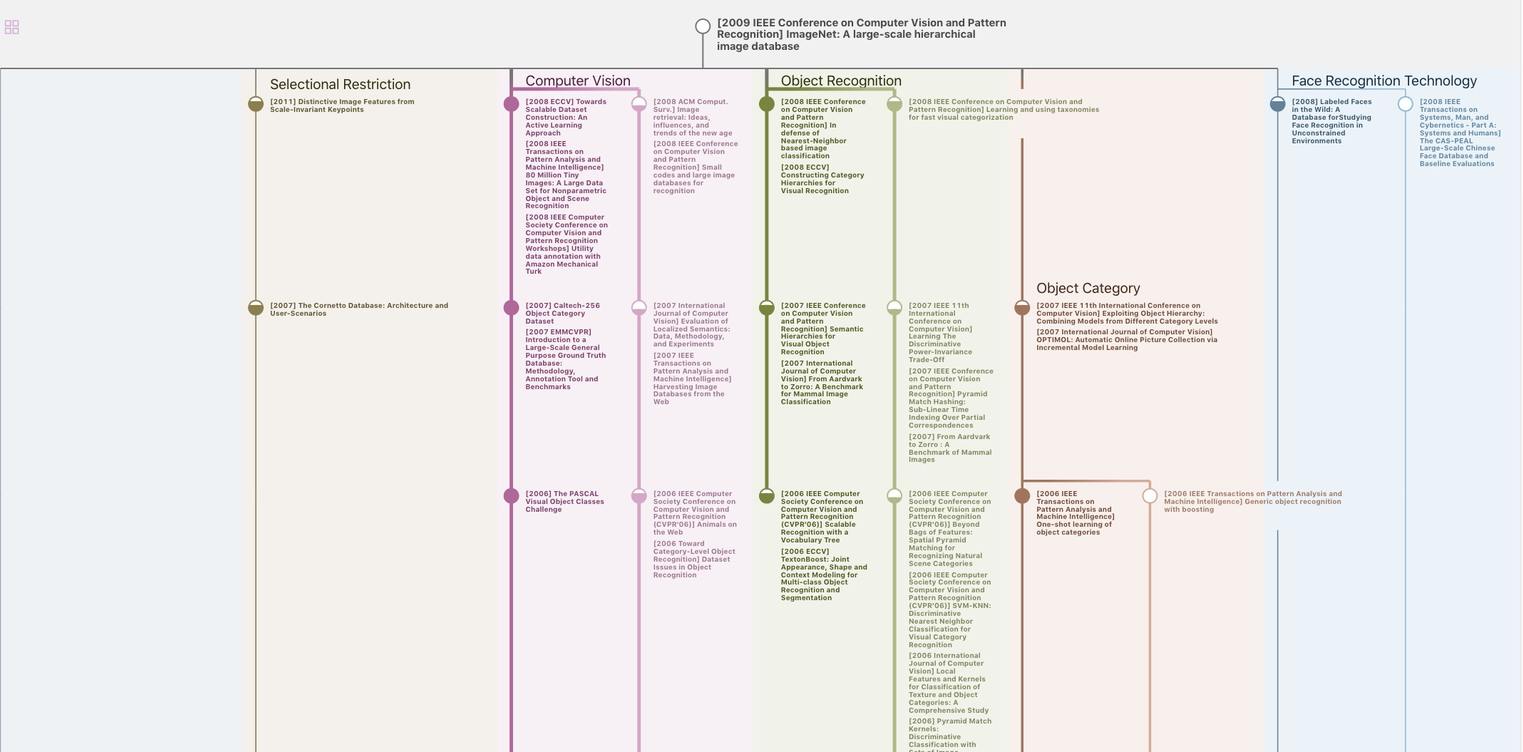
生成溯源树,研究论文发展脉络
Chat Paper
正在生成论文摘要