Piecewise-linear modelling with automated feature selection for Li-ion battery end-of-life prognosis
arxiv(2023)
摘要
The complex nature of lithium-ion battery degradation has led to many machine learning-based approaches for health forecasting being proposed in the literature. However, machine learning using sophisticated models can be computationally expensive, and although linear models are faster they can also be inflexible. Piecewise-linear models offer a compromise—a fast and flexible alternative that is not as computationally expensive as techniques such as neural networks or Gaussian process regression. Here, a piecewise-linear approach for battery health forecasting, including an automated feature selection step, is compared to a Gaussian process regression model and found to perform equally well in terms of the median error on a training dataset, and indeed somewhat better at the 95th percentile of error. The feature selection process demonstrates the benefit of limiting the correlation between inputs. Further trials found that the piecewise-linear approach was robust to changing input size and availability of training data.
更多查看译文
关键词
Feature selection,Linear model,Piecewise,Lithium-ion,Degradation,Health,Bayes
AI 理解论文
溯源树
样例
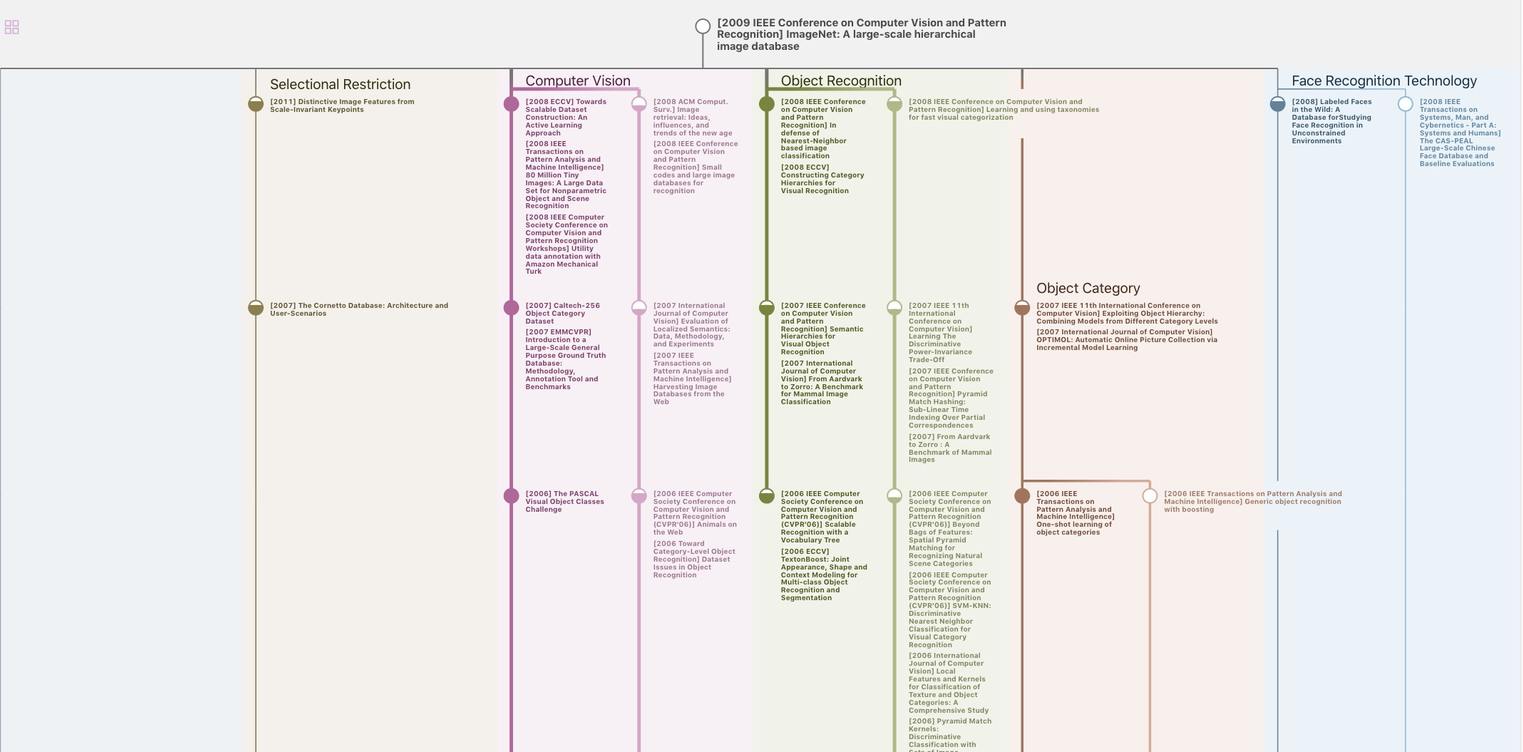
生成溯源树,研究论文发展脉络
Chat Paper
正在生成论文摘要