Pra-Net: Point Relation-Aware Network For 3d Point Cloud Analysis
IEEE TRANSACTIONS ON IMAGE PROCESSING(2021)
摘要
Learning intra-region contexts and inter-region relations are two effective strategies to strengthen feature representations for point cloud analysis. However, unifying the two strategies for point cloud representation is not fully emphasized in existing methods. To this end, we propose a novel framework named Point Relation-Aware Network (PRA-Net), which is composed of an Intra-region Structure Learning (ISL) module and an Inter-region Relation Learning (IRL) module. The ISL module can dynamically integrate the local structural information into the point features, while the IRL module captures inter-region relations adaptively and efficiently via a differentiable region partition scheme and a representative point-based strategy. Extensive experiments on several 3D benchmarks covering shape classification, keypoint estimation, and part segmentation have verified the effectiveness and the generalization ability of PRA-Net. Code will be available at https://github.com/XiwuChen/PRA-Net.
更多查看译文
关键词
Three-dimensional displays, Feature extraction, Shape, Aggregates, Kernel, Benchmark testing, Solid modeling, Point cloud, shape analysis, intra-region contexts, inter-region relations
AI 理解论文
溯源树
样例
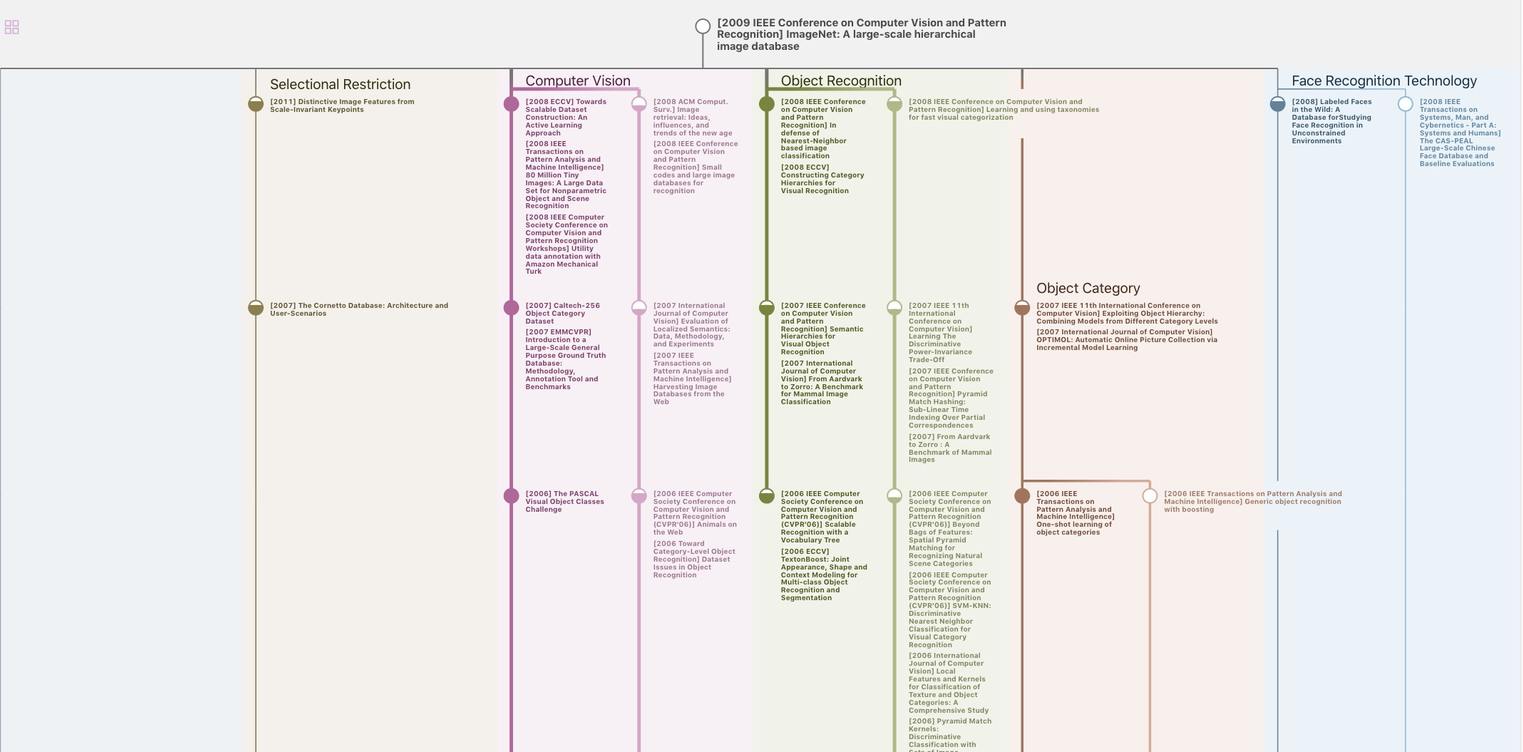
生成溯源树,研究论文发展脉络
Chat Paper
正在生成论文摘要