Attention in Attention Networks for Person Retrieval
IEEE Transactions on Pattern Analysis and Machine Intelligence(2022)
摘要
This paper generalizes the Attention in Attention (AiA) mechanism, in P. Fang et al., 2019 by employing explicit mapping in reproducing kernel Hilbert spaces to generate attention values of the input feature map. The AiA mechanism models the capacity of building inter-dependencies among the local and global features by the interaction of inner and outer attention modules. Besides a vanilla AiA module, termed linear attention with AiA, two non-linear counterparts, namely, second-order polynomial attention and Gaussian attention, are also proposed to utilize the non-linear properties of the input features explicitly, via the second-order polynomial kernel and Gaussian kernel approximation. The deep convolutional neural network, equipped with the proposed AiA blocks, is referred to as Attention in Attention Network (AiA-Net). The AiA-Net learns to extract a discriminative pedestrian representation, which combines complementary person appearance and corresponding part features. Extensive ablation studies verify the effectiveness of the AiA mechanism and the use of non-linear features hidden in the feature map for attention design. Furthermore, our approach outperforms current state-of-the-art by a considerable margin across a number of benchmarks. In addition, state-of-the-art performance is also achieved in the video person retrieval task with the assistance of the proposed AiA blocks.
更多查看译文
关键词
Algorithms,Humans,Neural Networks, Computer,Pedestrians
AI 理解论文
溯源树
样例
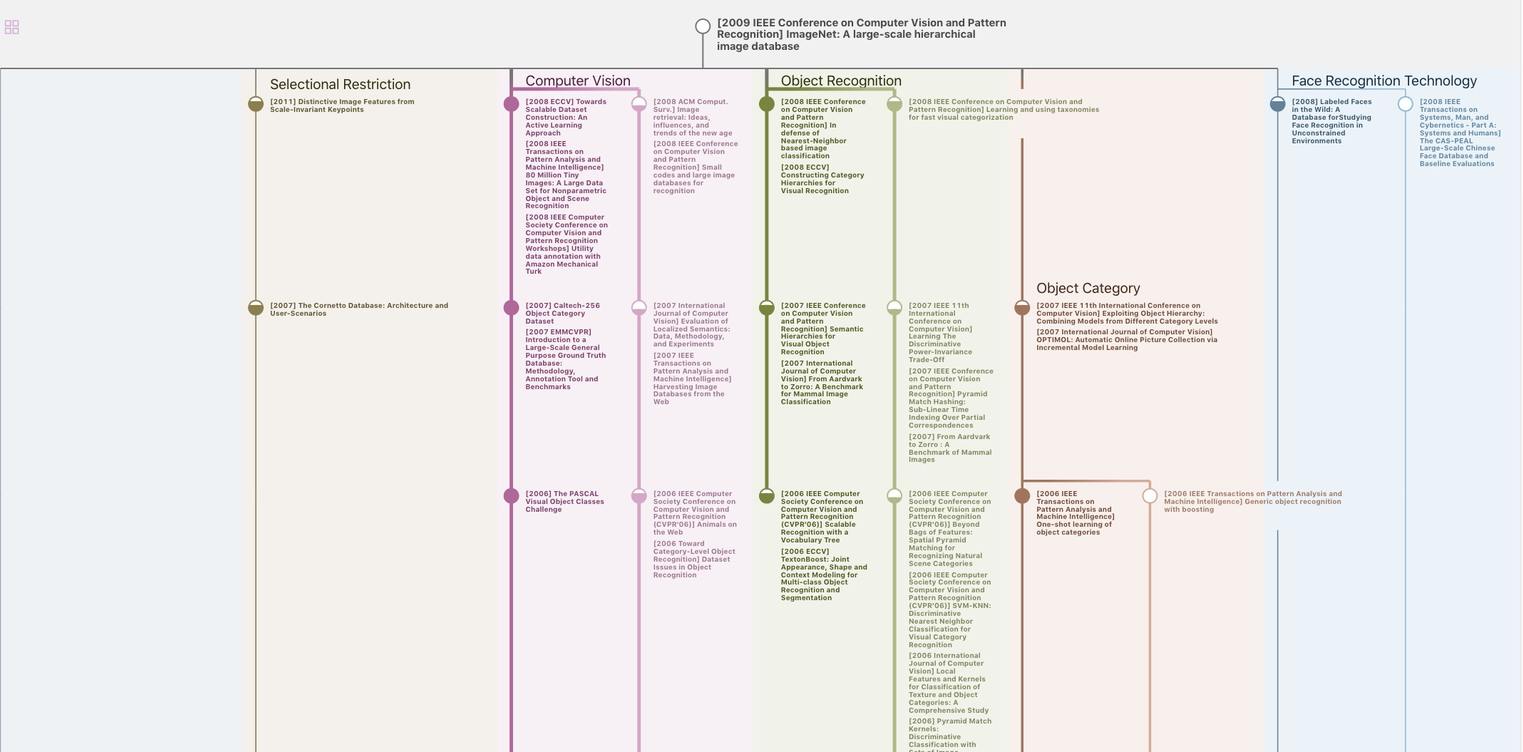
生成溯源树,研究论文发展脉络
Chat Paper
正在生成论文摘要