Spatio-temporal feature learning with reservoir computing for T-cell segmentation in live-cell Ca^2+ fluorescence microscopy
SCIENTIFIC REPORTS(2021)
摘要
dvances in high-resolution live-cell Ca^2+ imaging enabled subcellular localization of early Ca^2+ signaling events in T-cells and paved the way to investigate the interplay between receptors and potential target channels in Ca^2+ release events. The huge amount of acquired data requires efficient, ideally automated image processing pipelines, with cell localization/segmentation as central tasks. Automated segmentation in live-cell cytosolic Ca^2+ imaging data is, however, challenging due to temporal image intensity fluctuations, low signal-to-noise ratio, and photo-bleaching. Here, we propose a reservoir computing (RC) framework for efficient and temporally consistent segmentation. Experiments were conducted with Jurkat T-cells and anti-CD3 coated beads used for T-cell activation. We compared the RC performance with a standard U-Net and a convolutional long short-term memory (LSTM) model. The RC-based models (1) perform on par in terms of segmentation accuracy with the deep learning models for cell-only segmentation, but show improved temporal segmentation consistency compared to the U-Net; (2) outperform the U-Net for two-emission wavelengths image segmentation and differentiation of T-cells and beads; and (3) perform on par with the convolutional LSTM for single-emission wavelength T-cell/bead segmentation and differentiation. In turn, RC models contain only a fraction of the parameters of the baseline models and reduce the training time considerably.
更多查看译文
关键词
Biochemistry,Computational biology and bioinformatics,Engineering,Mathematics and computing,Medical research,Science,Humanities and Social Sciences,multidisciplinary
AI 理解论文
溯源树
样例
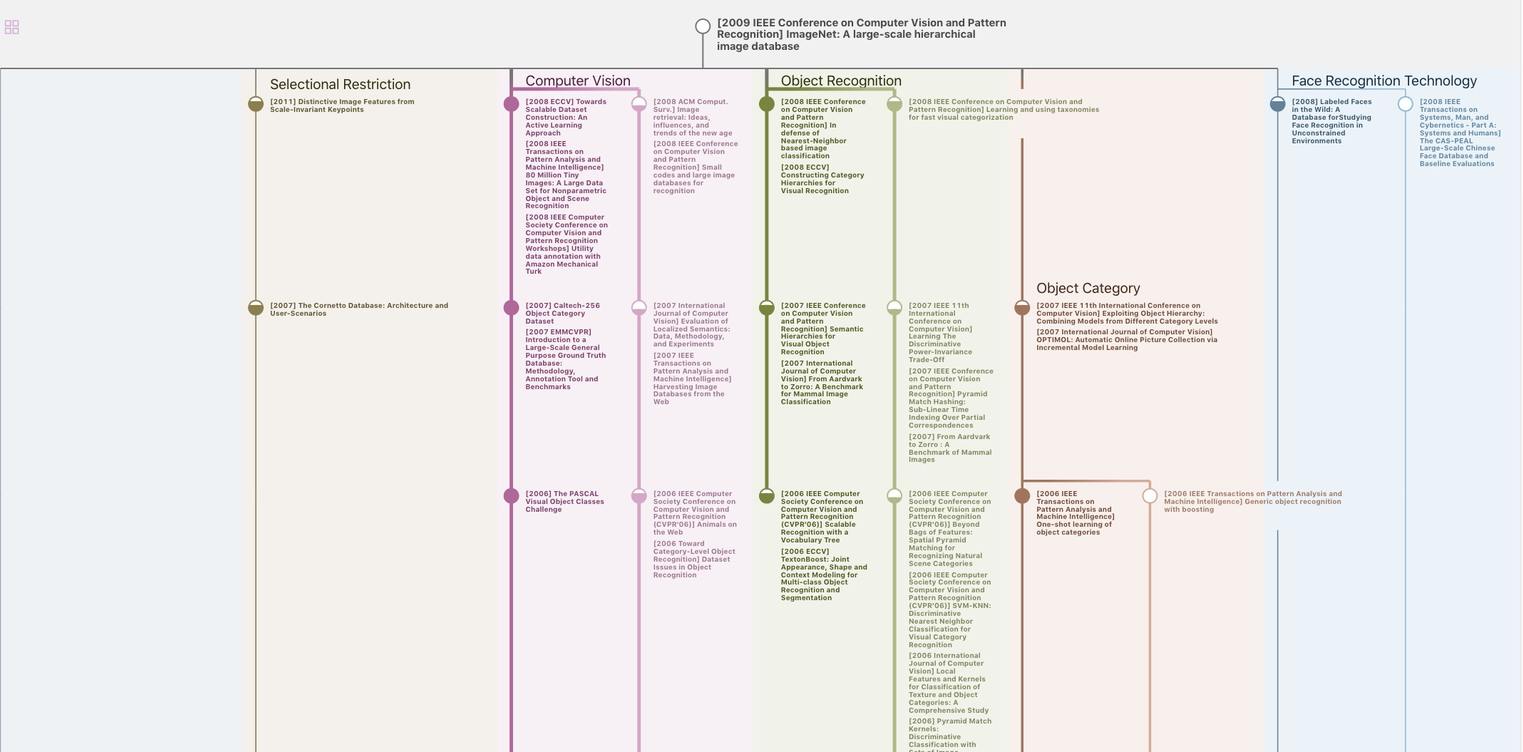
生成溯源树,研究论文发展脉络
Chat Paper
正在生成论文摘要