Immunoaizer: A Deep Learning-Based Computational Framework To Characterize Cell Distribution And Gene Mutation In Tumor Microenvironment
CANCERS(2021)
摘要
Simple SummaryA comprehensive evaluation of immune cell distribution in the tumor microenvironment (TME) and tumor gene mutation status may contribute to therapeutic optimization of cancer patients. In this study, we aimed to demonstrate that deep learning (DL)-based computational frameworks have remarkable potential as a tool to analyze the spatial distribution of immune cells and cancer cells in TME and detect tumor gene mutations. TME analysis can benefit from the computational framework, mainly due to its efficiency and low cost. Cells distribution in TME and tumor gene mutation status can be characterized accurately and efficiently. This may lead to a reduced working load of pathologists and may result in an improved and more standardized workflow.Spatial distribution of tumor infiltrating lymphocytes (TILs) and cancer cells in the tumor microenvironment (TME) along with tumor gene mutation status are of vital importance to the guidance of cancer immunotherapy and prognoses. In this work, we developed a deep learning-based computational framework, termed ImmunoAIzer, which involves: (1) the implementation of a semi-supervised strategy to train a cellular biomarker distribution prediction network (CBDPN) to make predictions of spatial distributions of CD3, CD20, PanCK, and DAPI biomarkers in the tumor microenvironment with an accuracy of 90.4%; (2) using CBDPN to select tumor areas on hematoxylin and eosin (H&E) staining tissue slides and training a multilabel tumor gene mutation detection network (TGMDN), which can detect APC, KRAS, and TP53 mutations with area-under-the-curve (AUC) values of 0.76, 0.77, and 0.79. These findings suggest that ImmunoAIzer could provide comprehensive information of cell distribution and tumor gene mutation status of colon cancer patients efficiently and less costly; hence, it could serve as an effective auxiliary tool for the guidance of immunotherapy and prognoses. The method is also generalizable and has the potential to be extended for application to other types of cancers other than colon cancer.
更多查看译文
关键词
deep learning, cell distribution, biomarker, tumor gene mutation, tumor microenvironment (TME), semi-supervised learning, hematoxylin and eosin (H&, E)
AI 理解论文
溯源树
样例
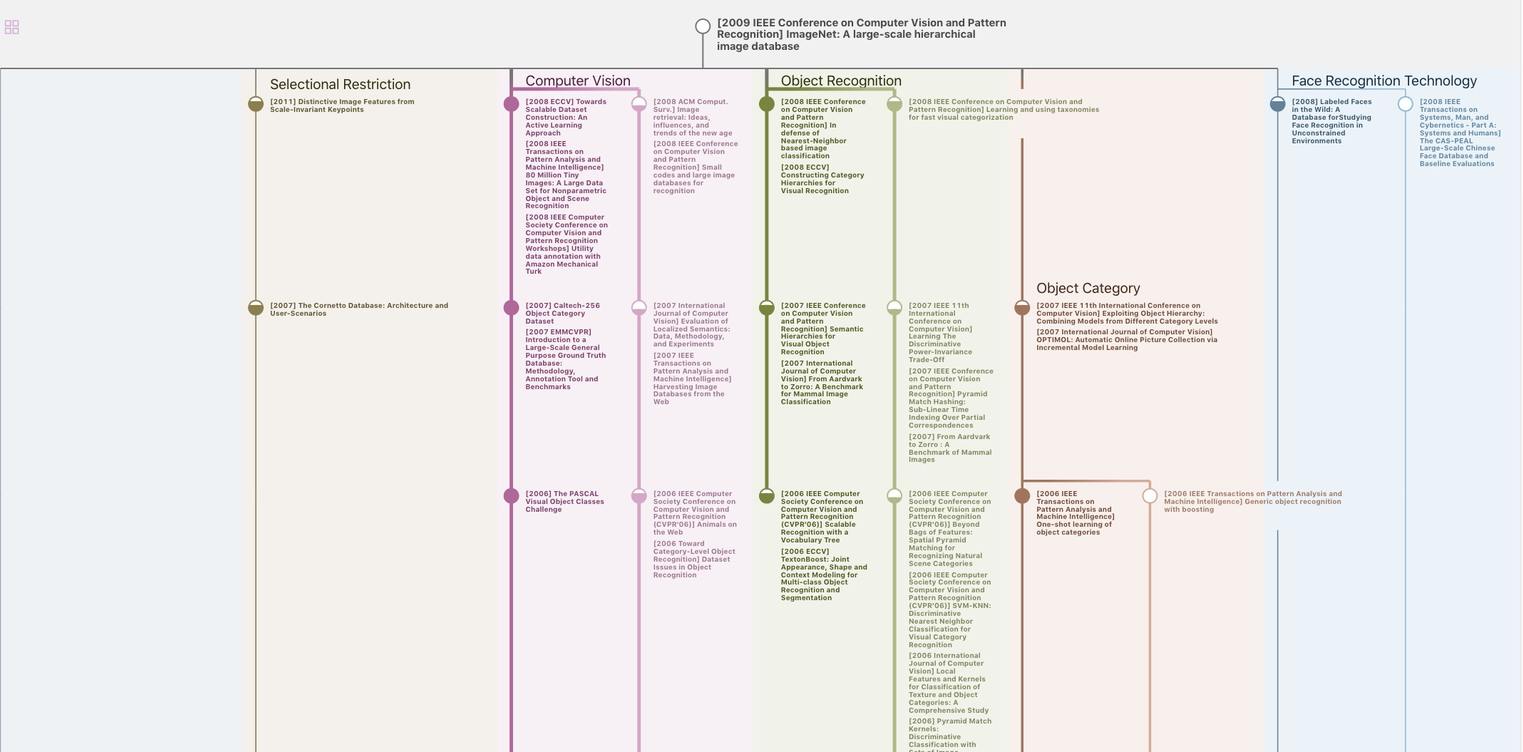
生成溯源树,研究论文发展脉络
Chat Paper
正在生成论文摘要