Inference of Upcoming Human Grasp Using EMG During Reach-to-Grasp Movement
arxiv(2022)
摘要
Electromyography (EMG) data has been extensively adopted as an intuitive interface for instructing human-robot collaboration. A major challenge of the real-time detection of human grasp intent is the identification of dynamic EMG from hand movements. Previous studies mainly implemented steady-state EMG classification with a small number of grasp patterns on dynamic situations, which are insufficient to generate differentiated control regarding the muscular activity variation in practice. In order to better detect dynamic movements, more EMG variability could be integrated into the model. However, only limited research were concentrated on such detection of dynamic grasp motions, and most existing assessments on non-static EMG classification either require supervised ground-truth timestamps of the movement status, or only contain limited kinematic variations. In this study, we propose a framework for classifying dynamic EMG signals into gestures, and examine the impact of different movement phases, using an unsupervised method to segment and label the action transitions. We collected and utilized data from large gesture vocabularies with multiple dynamic actions to encode the transitions from one grasp intent to another based on common sequences of the grasp movements. The classifier for identifying the gesture label was constructed afterwards based on the dynamic EMG signal, with no supervised annotation of kinematic movements required. Finally, we evaluated the performances of several training strategies using EMG data from different movement phases, and explored the information revealed from each phase. All experiments were evaluated in a real-time style with the performance transitions over time presented.
更多查看译文
关键词
electromyography (EMG) signals, dynamic EMG, gesture classification, human intent inference, machine learning
AI 理解论文
溯源树
样例
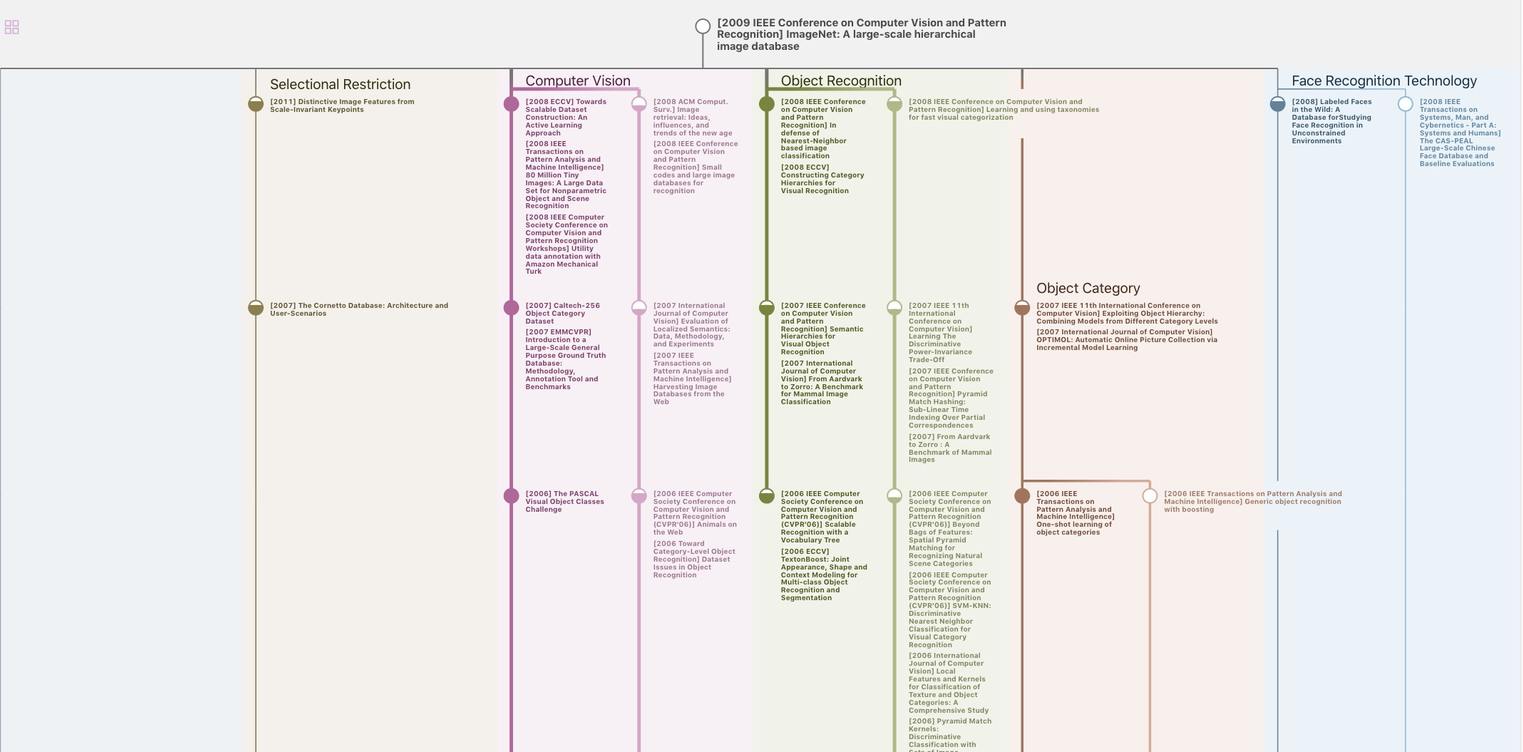
生成溯源树,研究论文发展脉络
Chat Paper
正在生成论文摘要