Development of an accessible 10-year Digital CArdioVAscular (DiCAVA) risk assessment: a UK Biobank study
EUROPEAN HEART JOURNAL - DIGITAL HEALTH(2021)
摘要
Aims Cardiovascular diseases (CVDs) are among the leading causes of death worldwide. Predictive scores providing personalized risk of developing CVD are increasingly used in clinical practice. Most scores, however, utilize a homogenous set of features and require the presence of a physician. The aim was to develop a new risk model (DiCAVA) using statistical and machine learning techniques that could be applied in a remote setting. A secondary goal was to identify new patient-centric variables that could be incorporated into CVD risk assessments. Methods and results Across 466 052 participants, Cox proportional hazards (CPH) and DeepSurv models were trained using 608 variables derived from the UK Biobank to investigate the 10-year risk of developing a CVD. Data-driven feature selection reduced the number of features to 47, after which reduced models were trained. Both models were compared to the Framingham score. The reduced CPH model achieved a c-index of 0.7443, whereas DeepSurv achieved a c-index of 0.7446. Both CPH and DeepSurv were superior in determining the CVD risk compared to Framingham score. Minimal difference was observed when cholesterol and blood pressure were excluded from the models (CPH: 0.741, DeepSurv: 0.739). The models show very good calibration and discrimination on the test data. Conclusion We developed a cardiovascular risk model that has very good predictive capacity and encompasses new variables. The score could be incorporated into clinical practice and utilized in a remote setting, without the need of including cholesterol. Future studies will focus on external validation across heterogeneous samples. [GRAPHICS] .
更多查看译文
关键词
Cardiovascular disease,Prediction,Risk modelling,Machine learning,Lifestyle
AI 理解论文
溯源树
样例
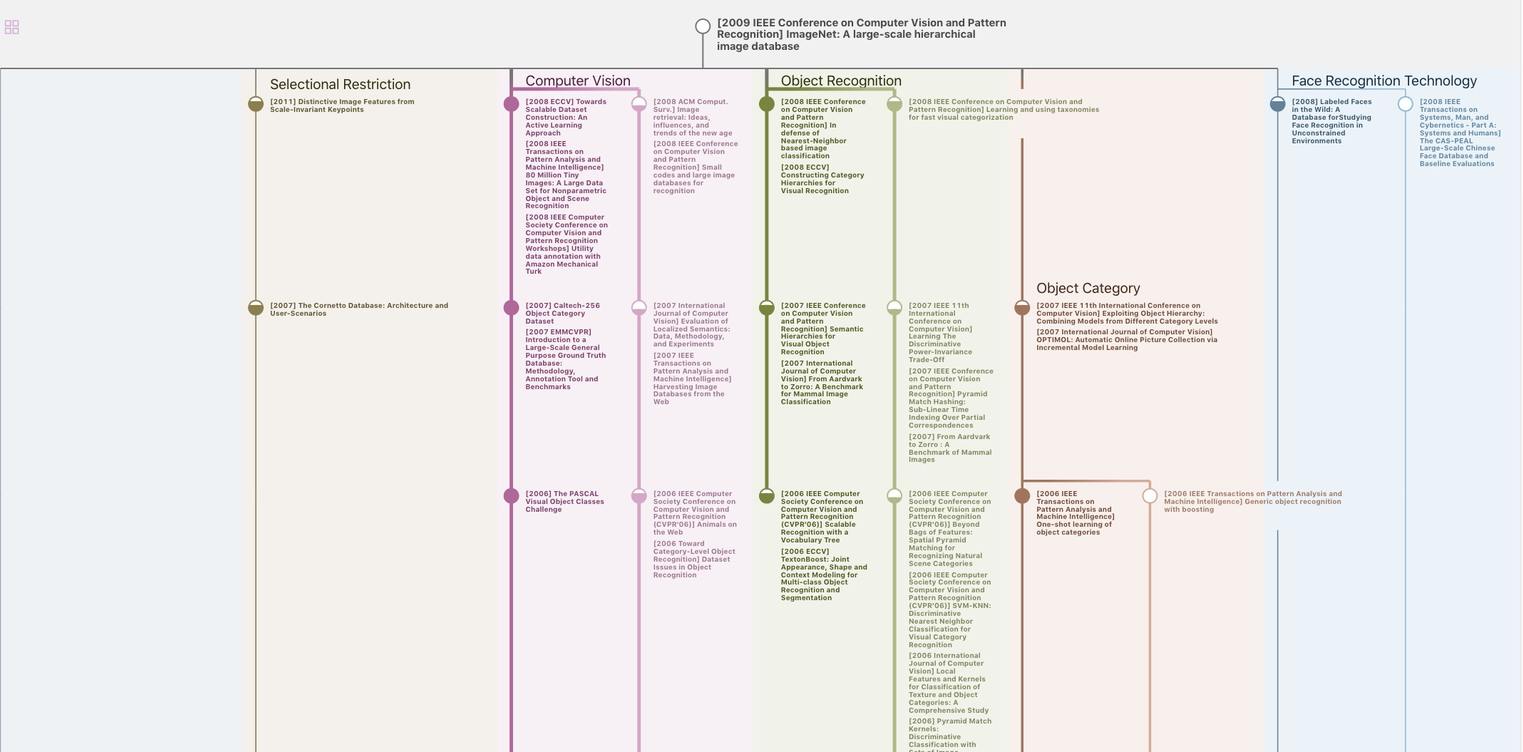
生成溯源树,研究论文发展脉络
Chat Paper
正在生成论文摘要