Improving BERT Pretraining with Syntactic Supervision
ArXiv(2021)
摘要
Bidirectional masked Transformers have become the core theme in the current NLP landscape. Despite their impressive benchmarks, a recurring theme in recent research has been to question such models' capacity for syntactic generalization. In this work, we seek to address this question by adding a supervised, token-level supertagging objective to standard unsupervised pretraining, enabling the explicit incorporation of syntactic biases into the network's training dynamics. Our approach is straightforward to implement, induces a marginal computational overhead and is general enough to adapt to a variety of settings. We apply our methodology on Lassy Large, an automatically annotated corpus of written Dutch. Our experiments suggest that our syntax-aware model performs on par with established baselines, despite Lassy Large being one order of magnitude smaller than commonly used corpora.
更多查看译文
关键词
bert pretraining,syntactic supervision
AI 理解论文
溯源树
样例
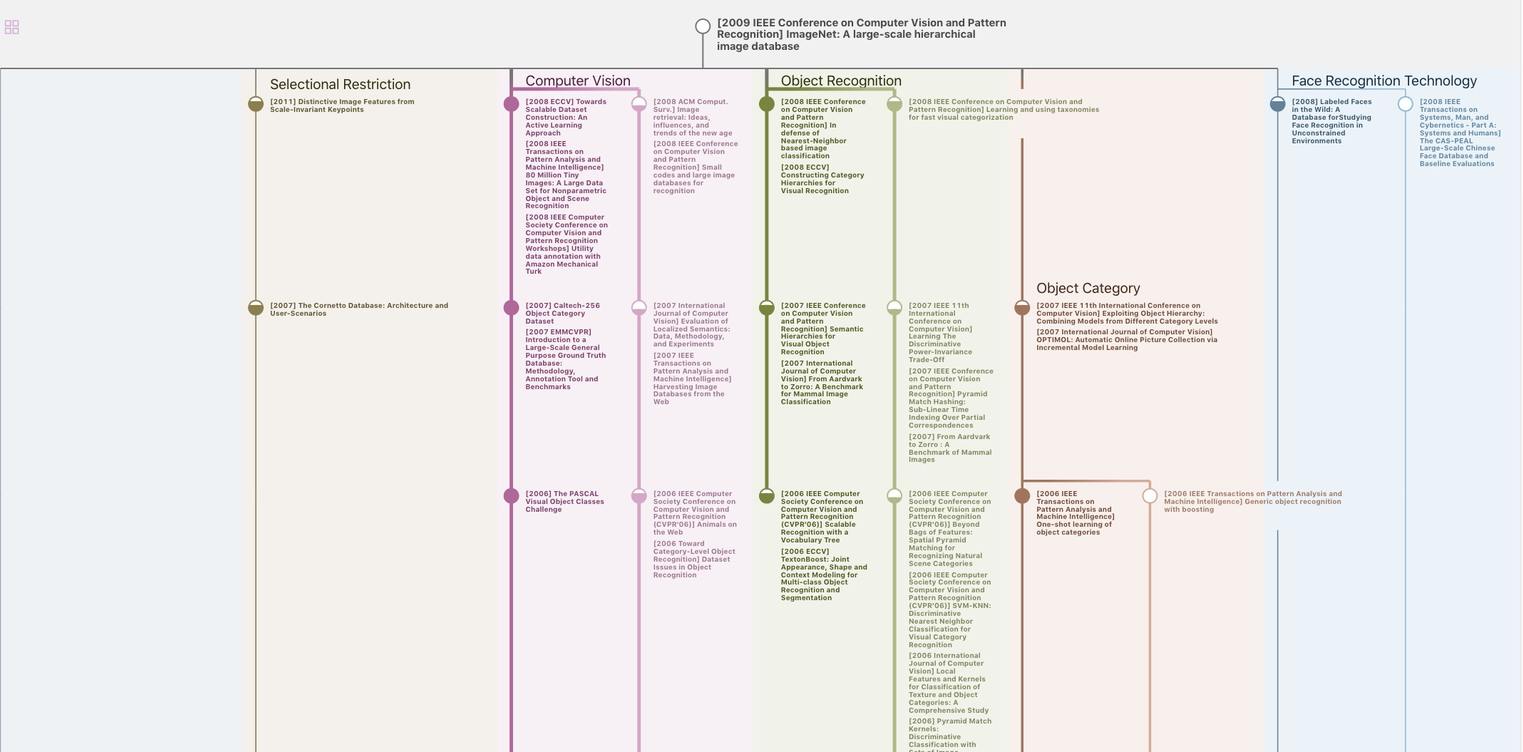
生成溯源树,研究论文发展脉络
Chat Paper
正在生成论文摘要