Cycle and Semantic Consistent Adversarial Domain Adaptation for Reducing Simulation-to-Real Domain Shift in LiDAR Bird's Eye View.
ITSC(2021)
摘要
The performance of object detection methods based on LiDAR information is heavily impacted by the availability of training data, usually limited to certain laser devices. As a result, the use of synthetic data is becoming popular when training neural network models, as both sensor specifications and driving scenarios can be generated ad-hoc. However, bridging the gap between virtual and real environments is still an open challenge, as current simulators cannot completely mimic real LiDAR operation. To tackle this issue, domain adaptation strategies are usually applied, obtaining remarkable results on vehicle detection when applied to range view (RV) and bird's eye view (BEV) projections while failing for smaller road agents. In this paper, we present a BEV domain adaptation method based on CycleGAN that uses prior semantic classification in order to preserve the information of small objects of interest during the domain adaptation process. The quality of the generated BEVs has been evaluated using a state-of-the-art 3D object detection framework at KITTI 3D Object Detection Benchmark. The obtained results show the advantages of the proposed method over the existing alternatives.
更多查看译文
关键词
object detection methods,LiDAR information,training data,laser devices,synthetic data,neural network models,sensor specifications,driving scenarios,virtual environments,LiDAR operation,vehicle detection,smaller road agents,BEV domain adaptation method,KITTI 3D object detection benchmark,simulation-to-real domain shift,LiDAR bird's eye view,range view,semantic classification
AI 理解论文
溯源树
样例
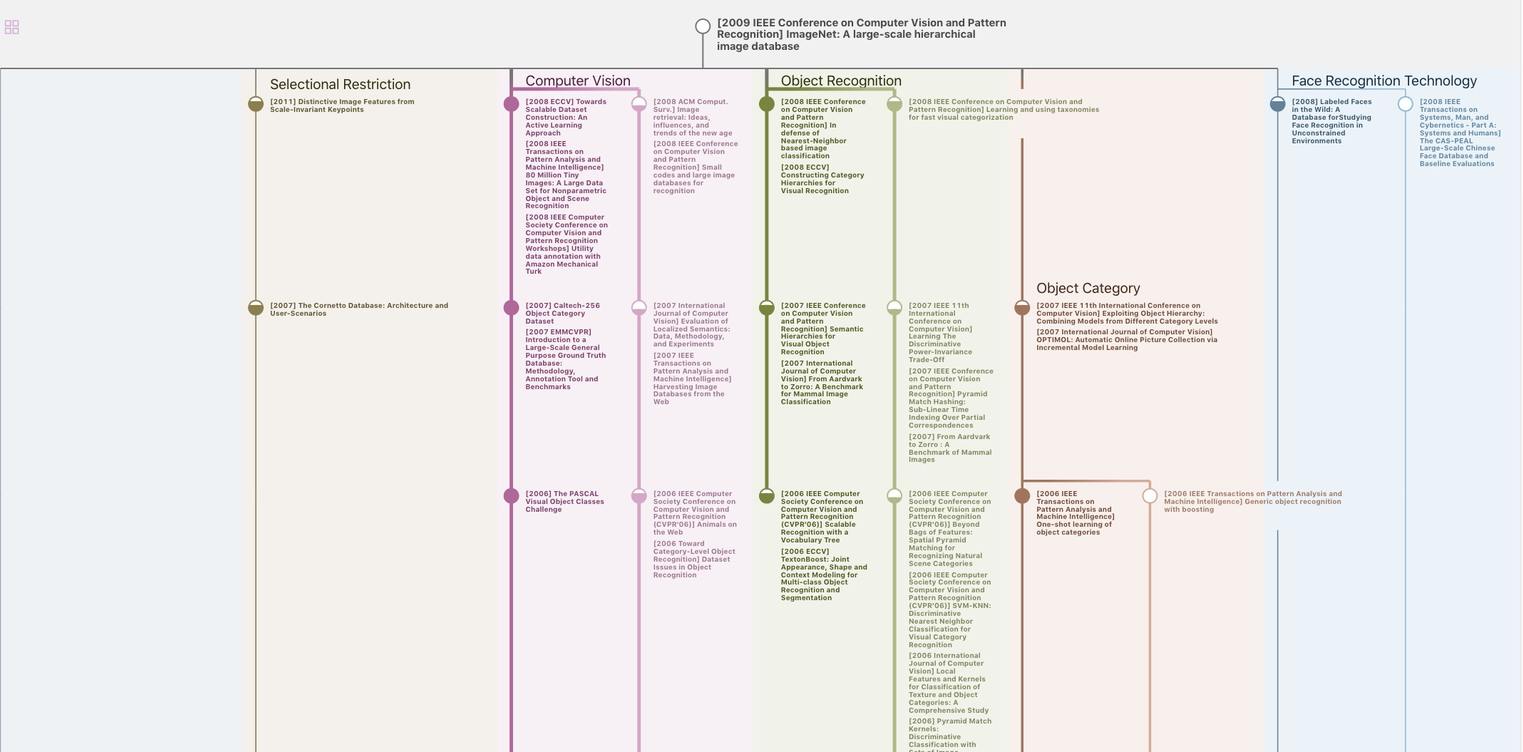
生成溯源树,研究论文发展脉络
Chat Paper
正在生成论文摘要