Are Explanations Helpful? A Comparative Study of the Effects of Explanations in AI-Assisted Decision-Making
Intelligent User Interfaces(2021)
摘要
ABSTRACTThis paper contributes to the growing literature in empirical evaluation of explainable AI (XAI) methods by presenting a comparison on the effects of a set of established XAI methods in AI-assisted decision making. Specifically, based on our review of previous literature, we highlight three desirable properties that ideal AI explanations should satisfy—improve people’s understanding of the AI model, help people recognize the model uncertainty, and support people’s calibrated trust in the model. Through randomized controlled experiments, we evaluate whether four types of common model-agnostic explainable AI methods satisfy these properties on two types of decision making tasks where people perceive themselves as having different levels of domain expertise in (i.e., recidivism prediction and forest cover prediction). Our results show that the effects of AI explanations are largely different on decision making tasks where people have varying levels of domain expertise in, and many AI explanations do not satisfy any of the desirable properties for tasks that people have little domain expertise in. Further, for decision making tasks that people are more knowledgeable, feature contribution explanation is shown to satisfy more desiderata of AI explanations, while the explanation that is considered to resemble how human explain decisions (i.e., counterfactual explanation) does not seem to improve calibrated trust. We conclude by discussing the implications of our study for improving the design of XAI methods to better support human decision making.
更多查看译文
关键词
interpretable machine learning, explainable AI, trust, trust calibration, human-subject experiments
AI 理解论文
溯源树
样例
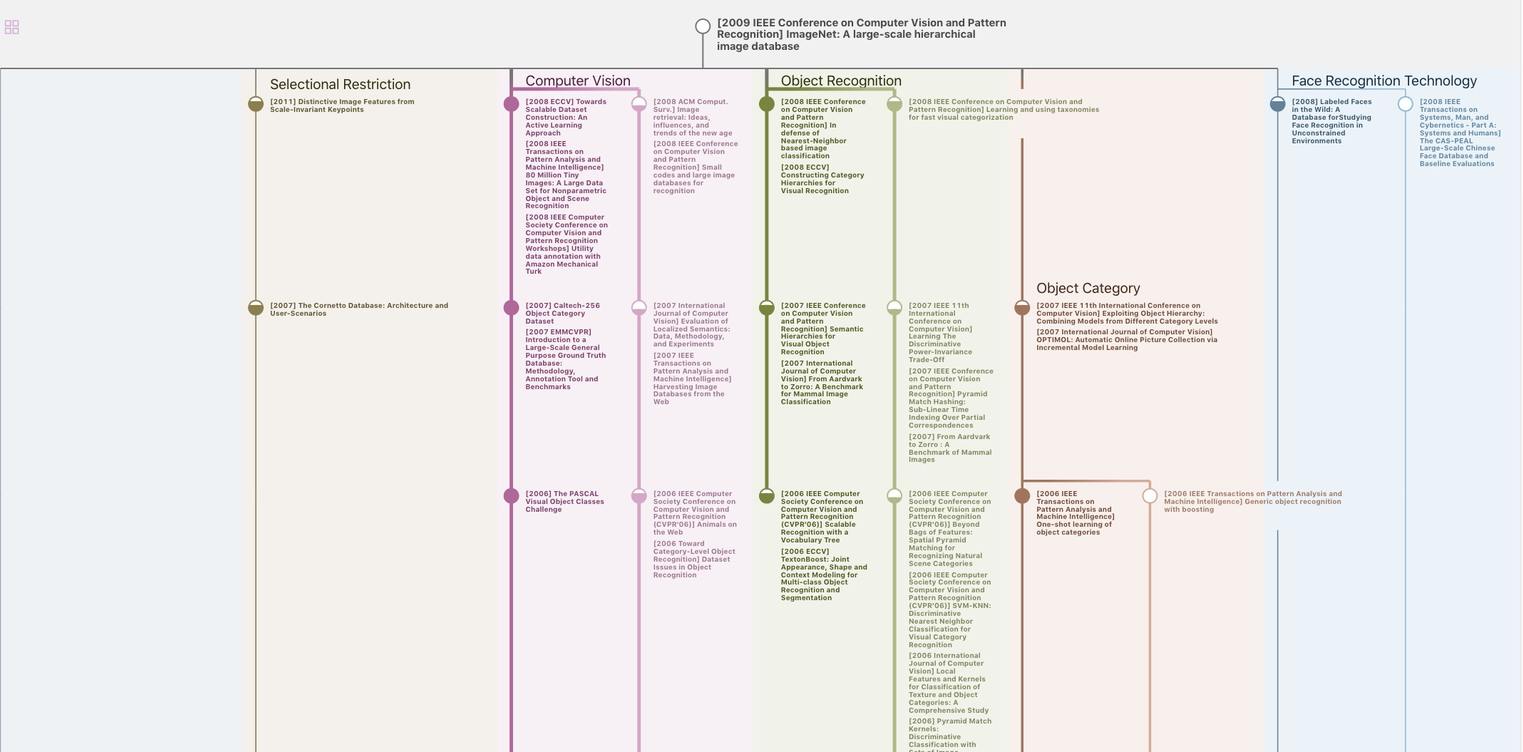
生成溯源树,研究论文发展脉络
Chat Paper
正在生成论文摘要