In-Situ Classification Of Cattle Behavior Using Accelerometry Data
COMPUTERS AND ELECTRONICS IN AGRICULTURE(2021)
摘要
We consider the problem of real-time classification of cattle behavior using accelerometry data on resource constrained sensor nodes. We develop a pipeline of preprocessing, feature extraction, and classification specifically designed for performing inference on sensor-node embedded systems. The dataset on which we base our investigations was collected by tri-axial accelerometer sensors on collar tags fitted to ten cattle for about five weeks and annotated with associated behaviors using approximately 19 animal-hours of recorded visual observations. We analyze the statistical and spectral properties of the gathered accelerometry data. Based on the insights gained from the analysis, we devise a small set of computationally efficient yet powerful features to extract from the accelerometry data that are useful for building cattle behavior classification statistical models. We examine the classification performance of several supervised machine-learning algorithms on our dataset. We only consider algorithms whose learned model and inference process are suitable for implementation on embedded systems with limited computing resources. The cross-validated performance evaluation results show that, by using the proposed features, several computationally-efficient discriminative models can yield good classification accuracy in distinguishing four biologically important cattle behavior classes, namely, grazing, ruminating, resting, and other (a collective of all other behaviors). Particularly, this is achieved without incurring any significant burden on embedded system resources in terms of energy, computation, or memory.
更多查看译文
关键词
Animal behavior classification, Embedded systems, Feature engineering, Inertial measurements, In-situ classification, Precision agriculture, Wearable sensors
AI 理解论文
溯源树
样例
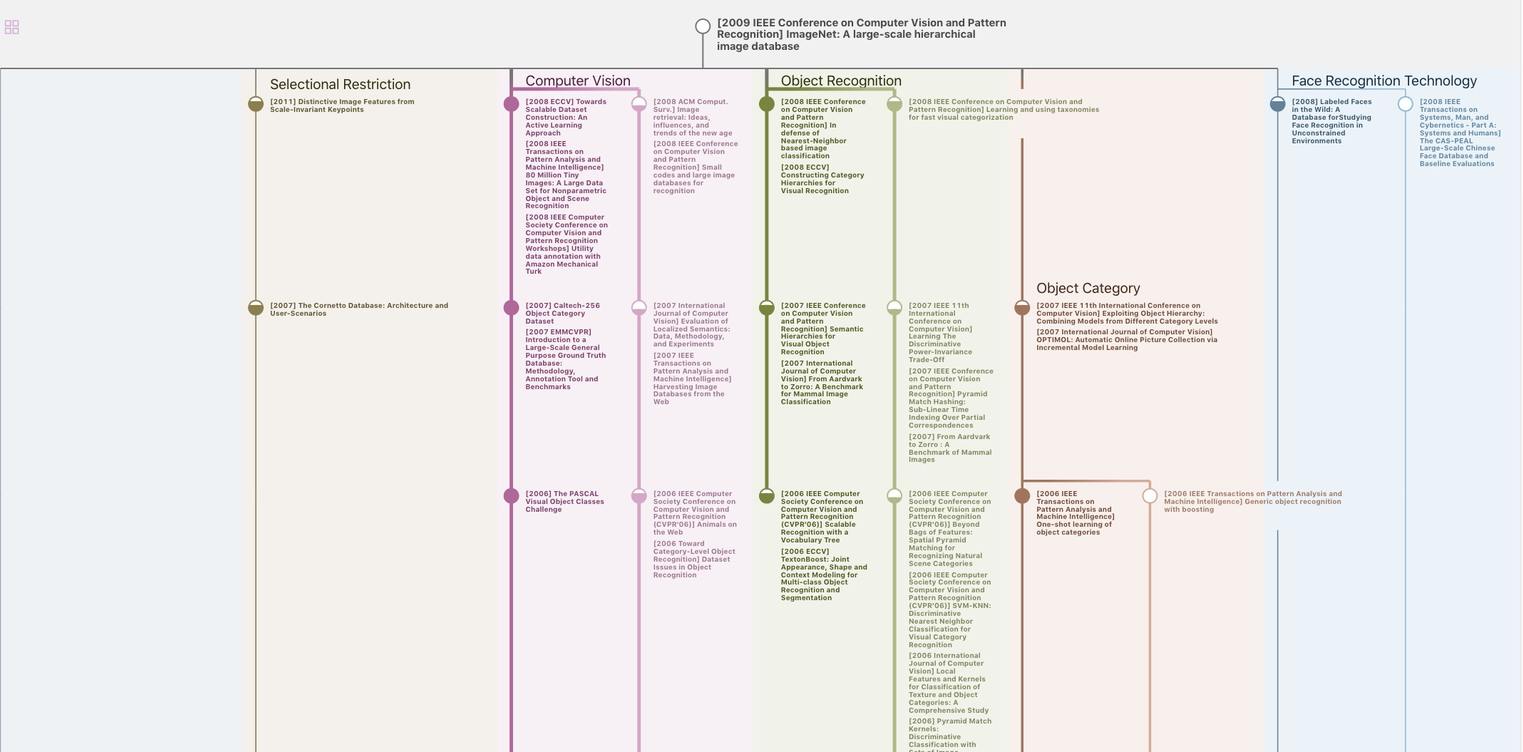
生成溯源树,研究论文发展脉络
Chat Paper
正在生成论文摘要