Exploiting Cloze-Questions for Few-Shot Text Classification and Natural Language Inference.
EACL(2021)
摘要
Some NLP tasks can be solved in a fully unsupervised fashion by providing a pretrained language model with “task descriptions” in natural language (e.g., Radford et al., 2019). While this approach underperforms its supervised counterpart, we show in this work that the two ideas can be combined: We introduce Pattern-Exploiting Training (PET), a semi-supervised training procedure that reformulates input examples as cloze-style phrases to help language models understand a given task. These phrases are then used to assign soft labels to a large set of unlabeled examples. Finally, standard supervised training is performed on the resulting training set. For several tasks and languages, PET outperforms supervised training and strong semi-supervised approaches in low-resource settings by a large margin.
更多查看译文
关键词
few shot text classification,cloze questions,language inference
AI 理解论文
溯源树
样例
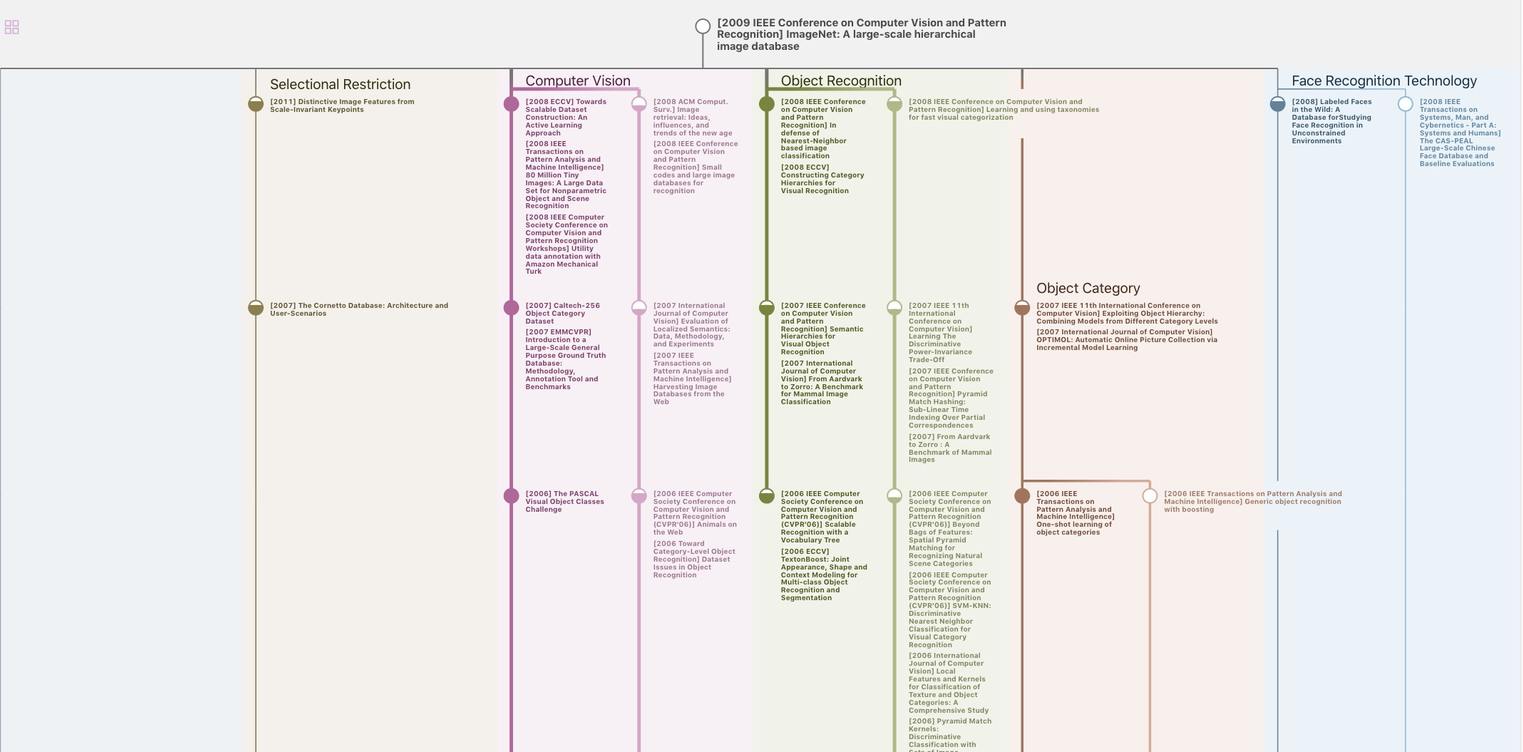
生成溯源树,研究论文发展脉络
Chat Paper
正在生成论文摘要