Operator Shifting for General Noisy Matrix Systems
SIAM Journal on Mathematics of Data Science(2022)
摘要
In the computational sciences, one must often estimate model parameters from data subject to noise and uncertainty, leading to inaccurate results. In order to improve the accuracy of models with noisy parameters, we consider the problem of reducing error in a linear system with the operator corrupted by noise. Our contribution in this paper is to extend the elliptic operator shifting framework from Etter and Ying, 2020 to the general nonsymmetric matrix case. Roughly, the operator shifting technique is a matrix analogue of the James--Stein estimator. The key insight is that a shift of the matrix inverse estimate in an appropriately chosen direction will reduce average error. In our extension, we interrogate a number of questions---namely, whether or not shifting towards the origin for general matrix inverses always reduces error as it does in the elliptic case. We show that this is usually the case, but that there are three key features of the general nonsingular matrices that allow for counterexamples not possible in the symmetric case. We prove that when these possibilities are eliminated by the assumption of noise symmetry and the use of the residual norm as the error metric, the optimal shift is always towards the origin, mirroring results from Etter and Ying, 2020. We also investigate behavior in the small noise regime and other scenarios. We conclude by presenting numerical experiments (with accompanying source code) inspired by reinforcement learning to demonstrate that operator shifting can yield substantial reductions in error.
更多查看译文
关键词
operator shifting, random matrices, Monte Carlo, polynomial expansion, asymmetric matrices, noise reduction
AI 理解论文
溯源树
样例
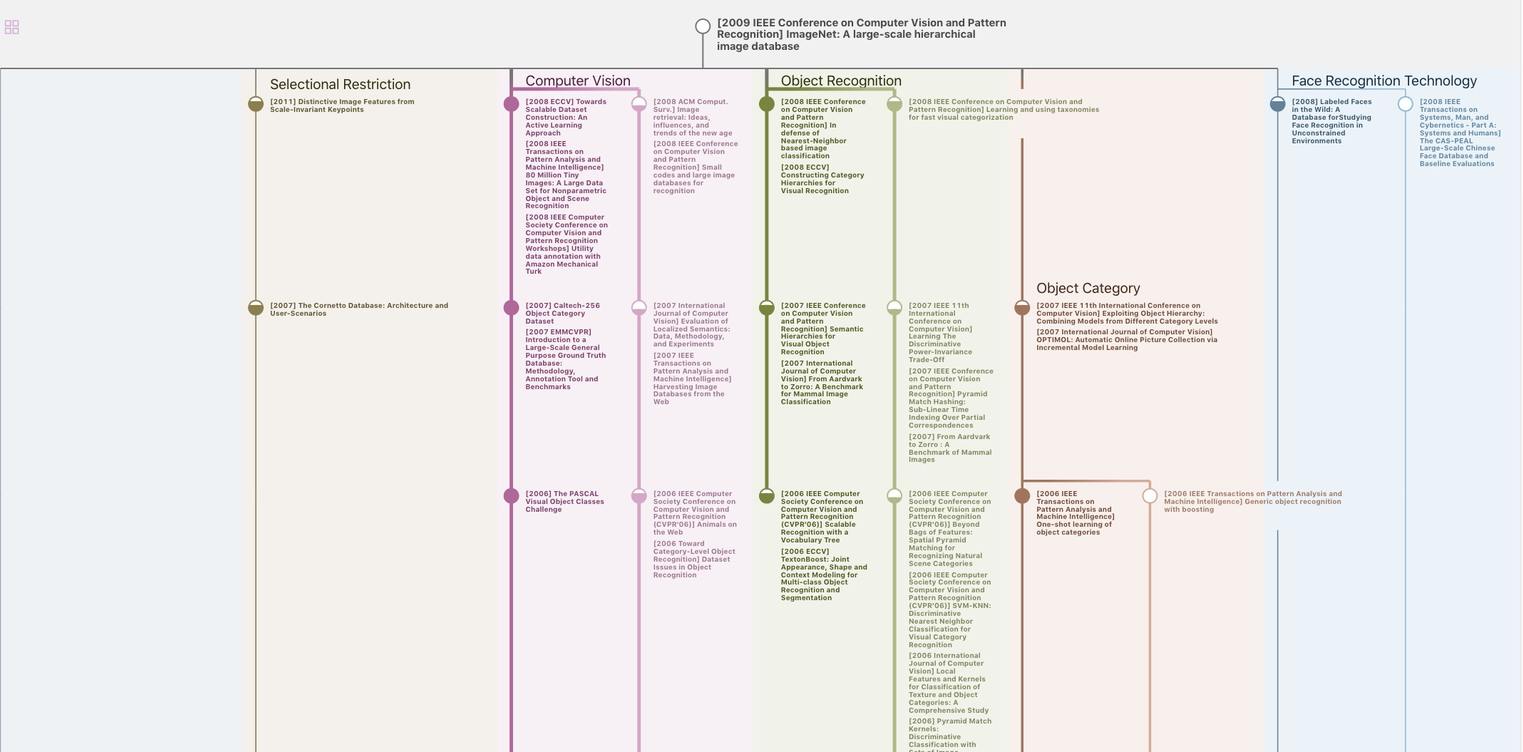
生成溯源树,研究论文发展脉络
Chat Paper
正在生成论文摘要