Constrained minimum energy designs
STATISTICS AND COMPUTING(2021)
摘要
Space-filling designs are important in computer experiments, which are critical for building a cheap surrogate model that adequately approximates an expensive computer code. Many design construction techniques in the existing literature are only applicable for rectangular bounded space, but in real-world applications, the input space can often be non-rectangular because of constraints on the input variables. One solution to generate designs in a constrained space is to first generate uniformly distributed samples in the feasible region, and then use them as the candidate set to construct the designs. Sequentially constrained Monte Carlo (SCMC) is the state-of-the-art technique for candidate generation, but it still requires large number of constraint evaluations, which is problematic especially when the constraints are expensive to evaluate. Thus, to reduce constraint evaluations and improve efficiency, we propose the constrained minimum energy design (CoMinED) that utilizes recent advances in deterministic sampling methods. Extensive simulation results on 15 benchmark problems with dimensions ranging from 2 to 13 are provided for demonstrating the improved performance of CoMinED over the existing methods.
更多查看译文
关键词
Computer experiment, Experimental design, Space-filling designs, Sequential Monte Carlo
AI 理解论文
溯源树
样例
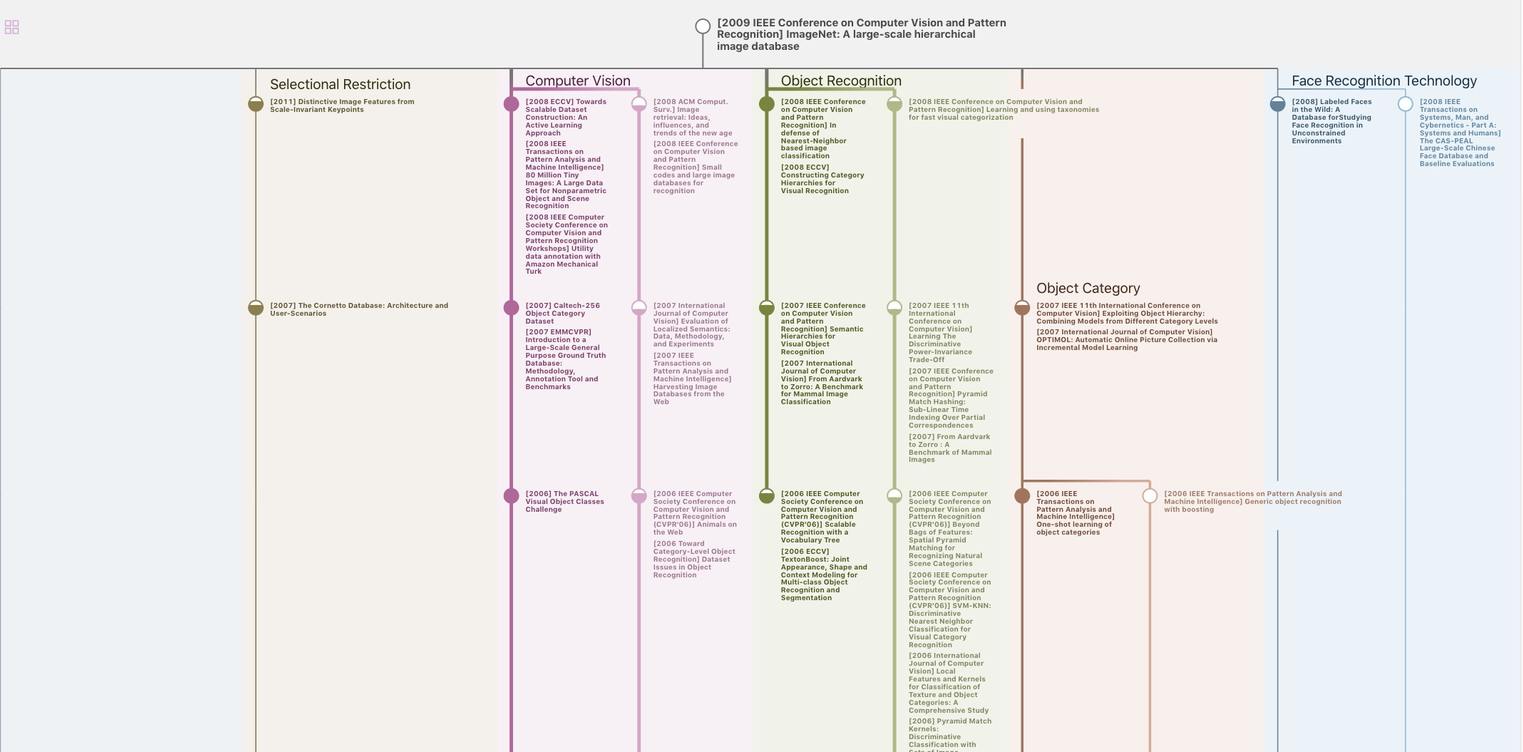
生成溯源树,研究论文发展脉络
Chat Paper
正在生成论文摘要