ECLIPSE: Envisioning CLoud Induced Perturbations in Solar Energy
Applied Energy(2022)
摘要
Efficient integration of solar energy into the electricity mix depends on a reliable anticipation of its intermittency. A promising approach to forecasting the temporal variability of solar irradiance resulting from the cloud cover dynamics is based on the analysis of sequences of ground-taken sky images or satellite observations. Despite encouraging results, a recurrent limitation of existing deep learning approaches lies in the ubiquitous tendency of reacting to past observations rather than actively anticipating future events. This leads to a frequent temporal lag and limited ability to predict sudden events. To address this challenge, we introduce ECLIPSE, a spatio-temporal neural network architecture that models cloud motion from sky images to not only predict future irradiance levels and associated uncertainties, but also segmented images, which provide richer information on the local irradiance map. We show that ECLIPSE anticipates critical events and reduces temporal delay while generating visually realistic futures. The model characteristics and properties are investigated with an ablation study and a comparative study on the benefits and different ways to integrate auxiliary data into the modelling. The model predictions are also interpreted through an analysis of the principal spatio-temporal components learned during network training.
更多查看译文
关键词
Solar energy,Nowcasting,Computer vision,Deep learning,Sky images,Satellite images
AI 理解论文
溯源树
样例
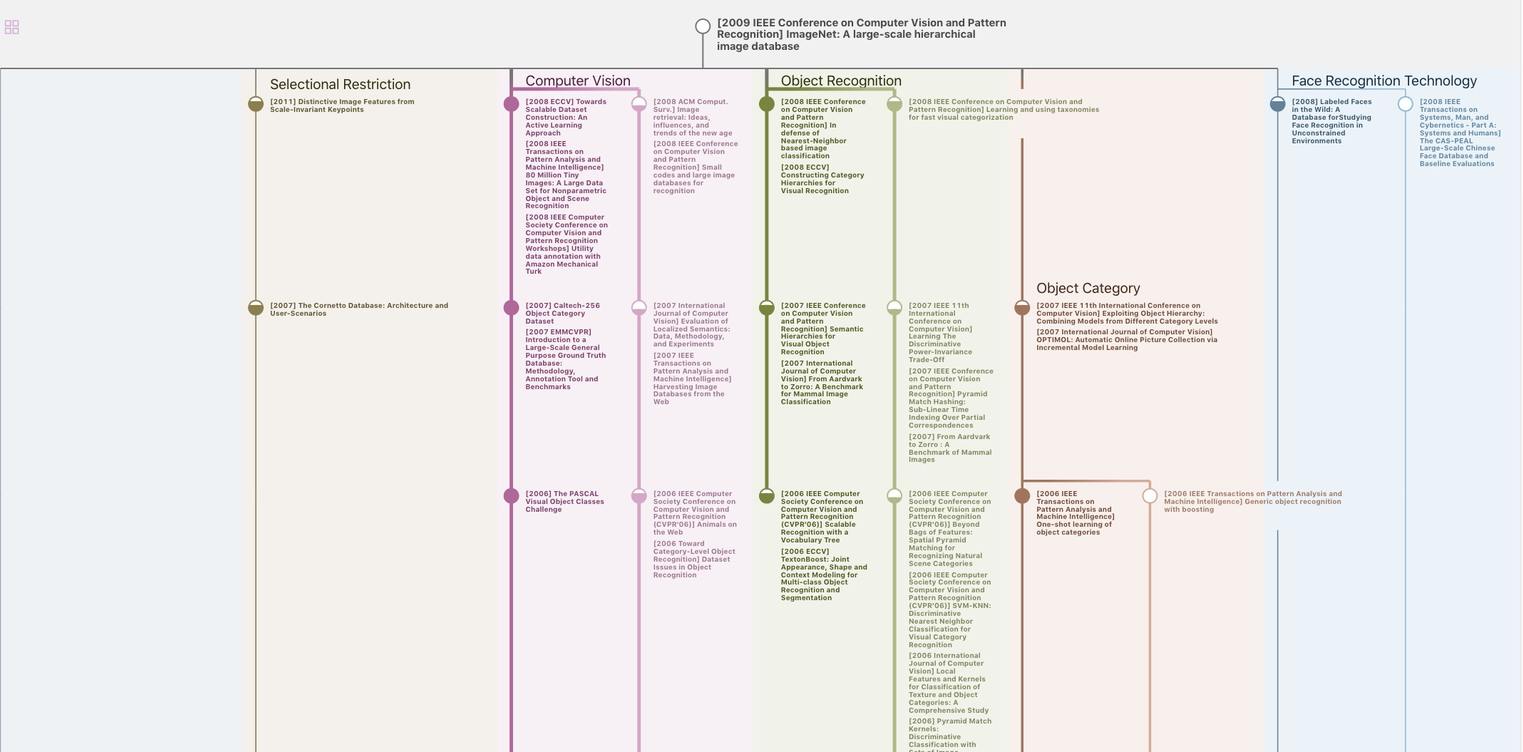
生成溯源树,研究论文发展脉络
Chat Paper
正在生成论文摘要