Impact of Spatial Frequency Based Constraints on Adversarial Robustness
2021 INTERNATIONAL JOINT CONFERENCE ON NEURAL NETWORKS (IJCNN)(2021)
摘要
Adversarial examples mainly exploit changes to input pixels to which humans are not sensitive to, and arise from the fact that models make decisions based on uninterpretable features. Interestingly, cognitive science reports that the process of interpretability for human classification decision relies predominantly on low spatial frequency components. In this paper, we investigate the robustness to adversarial perturbations of models enforced during training to leverage information corresponding to different spatial frequency ranges. We show that it is tightly linked to the spatial frequency characteristics of the data at stake. Indeed, depending on the data set, the same constraint may results in very different level of robustness (up to 0.41 adversarial accuracy difference). To explain this phenomenon, we conduct several experiments to enlighten influential factors such as the level of sensitivity to high frequencies, and the transferability of adversarial perturbations between original and low-pass filtered inputs.
更多查看译文
关键词
deep neural network, adversarial examples, frequency analysis
AI 理解论文
溯源树
样例
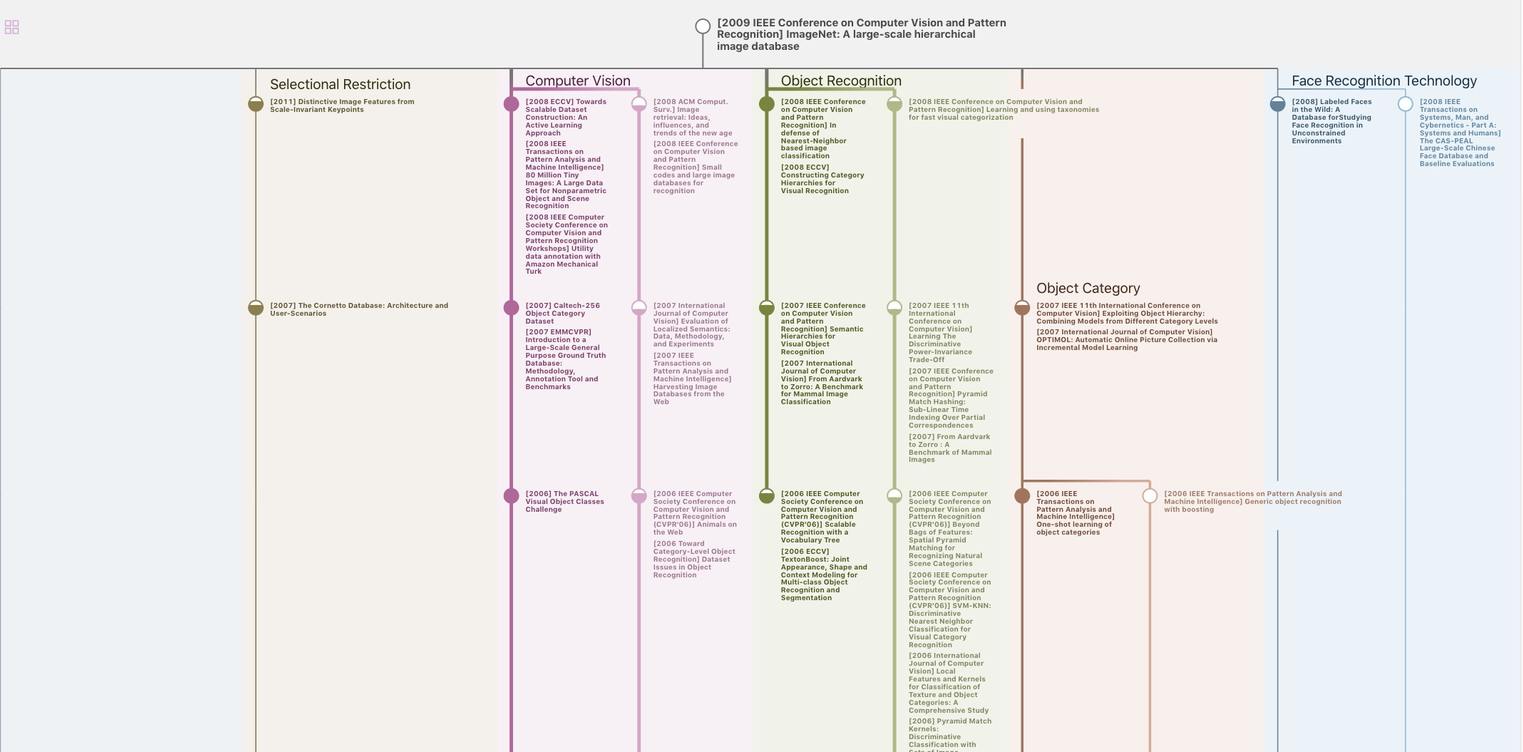
生成溯源树,研究论文发展脉络
Chat Paper
正在生成论文摘要