HodgeNet: learning spectral geometry on triangle meshes
ACM Transactions on Graphics(2021)
摘要
AbstractConstrained by the limitations of learning toolkits engineered for other applications, such as those in image processing, many mesh-based learning algorithms employ data flows that would be atypical from the perspective of conventional geometry processing. As an alternative, we present a technique for learning from meshes built from standard geometry processing modules and operations. We show that low-order eigenvalue/eigenvector computation from operators parameterized using discrete exterior calculus is amenable to efficient approximate backpropagation, yielding spectral per-element or per-mesh features with similar formulas to classical descriptors like the heat/wave kernel signatures. Our model uses few parameters, generalizes to high-resolution meshes, and exhibits performance and time complexity on par with past work.
更多查看译文
关键词
Machine learning, meshes, operators
AI 理解论文
溯源树
样例
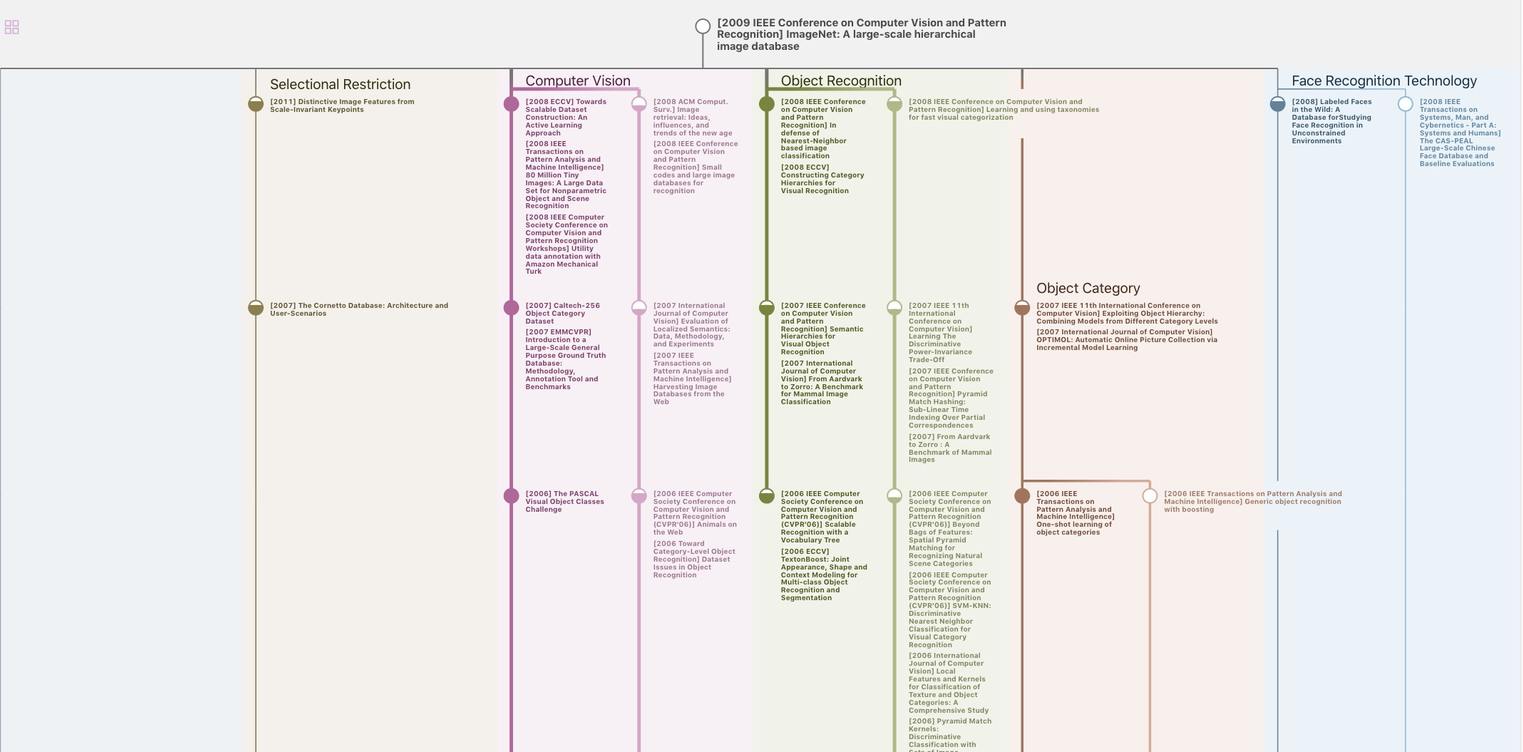
生成溯源树,研究论文发展脉络
Chat Paper
正在生成论文摘要