Multimodal Contrastive Training for Visual Representation Learning
2021 IEEE/CVF CONFERENCE ON COMPUTER VISION AND PATTERN RECOGNITION, CVPR 2021(2021)
摘要
We develop an approach to learning visual representations that embraces multimodal data, driven by a combination of intra- and inter-modal similarity preservation objectives. Unlike existing visual pre-training methods, which solve a proxy prediction task in a single domain, our method exploits intrinsic data properties within each modality and semantic information from cross-modal correlation simultaneously, hence improving the quality of learned visual representations. By including multimodal training in a unified framework with different types of contrastive losses, our method can learn more powerful and generic visual features. We first train our model on COCO and evaluate the learned visual representations on various downstream tasks including image classification, object detection, and instance segmentation. For example, the visual representations pre-trained on COCO by our method achieve state-of-the-art top-I validation accuracy of 55.3% on ImageNet classification, under the common transfer protocol. We also evaluate our method on the large-scale Stock images dataset and show its effectiveness on multi-label image tagging, and cross-modal retrieval tasks.
更多查看译文
关键词
multimodal contrastive training,learning,representation
AI 理解论文
溯源树
样例
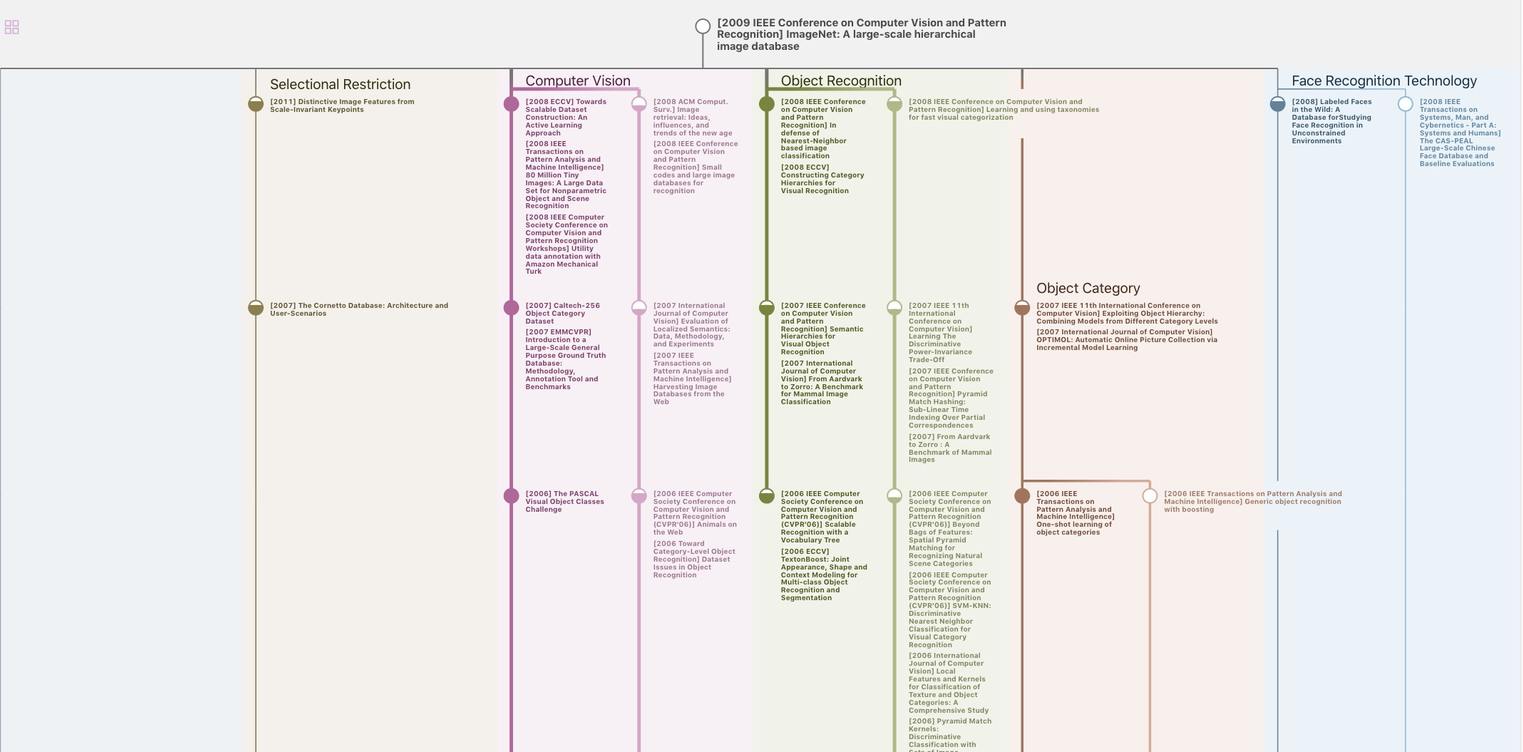
生成溯源树,研究论文发展脉络
Chat Paper
正在生成论文摘要