Learning Bayes-optimal dendritic opinion pooling
arxiv(2022)
Abstract
In functional network models, neurons are commonly conceptualized as linearly summing presynaptic inputs before applying a non-linear gain function to produce output activity. In contrast, synaptic coupling between neurons in the central nervous system is regulated by dynamic permeabilities of ion channels. So far, the computational role of these membrane conductances remains unclear and is often considered an artifact of the biological substrate. Here we demonstrate that conductance-based synaptic coupling allow neurons to represent, process and learn uncertainties. We suggest that membrane potentials and conductances on dendritic branches code opinions with associated reliabilities. The biophysics of the membrane combines these opinions by taking account their reliabilities, and the soma thus acts as a decision maker. We derive a gradient-based plasticity rule, allowing neurons to learn desired target distributions and weight synaptic inputs by their relative reliabilities. Our theory explains various experimental findings on the system and single-cell level related to multi-sensory integration, and makes testable predictions on dendritic integration and synaptic plasticity.
MoreTranslated text
AI Read Science
Must-Reading Tree
Example
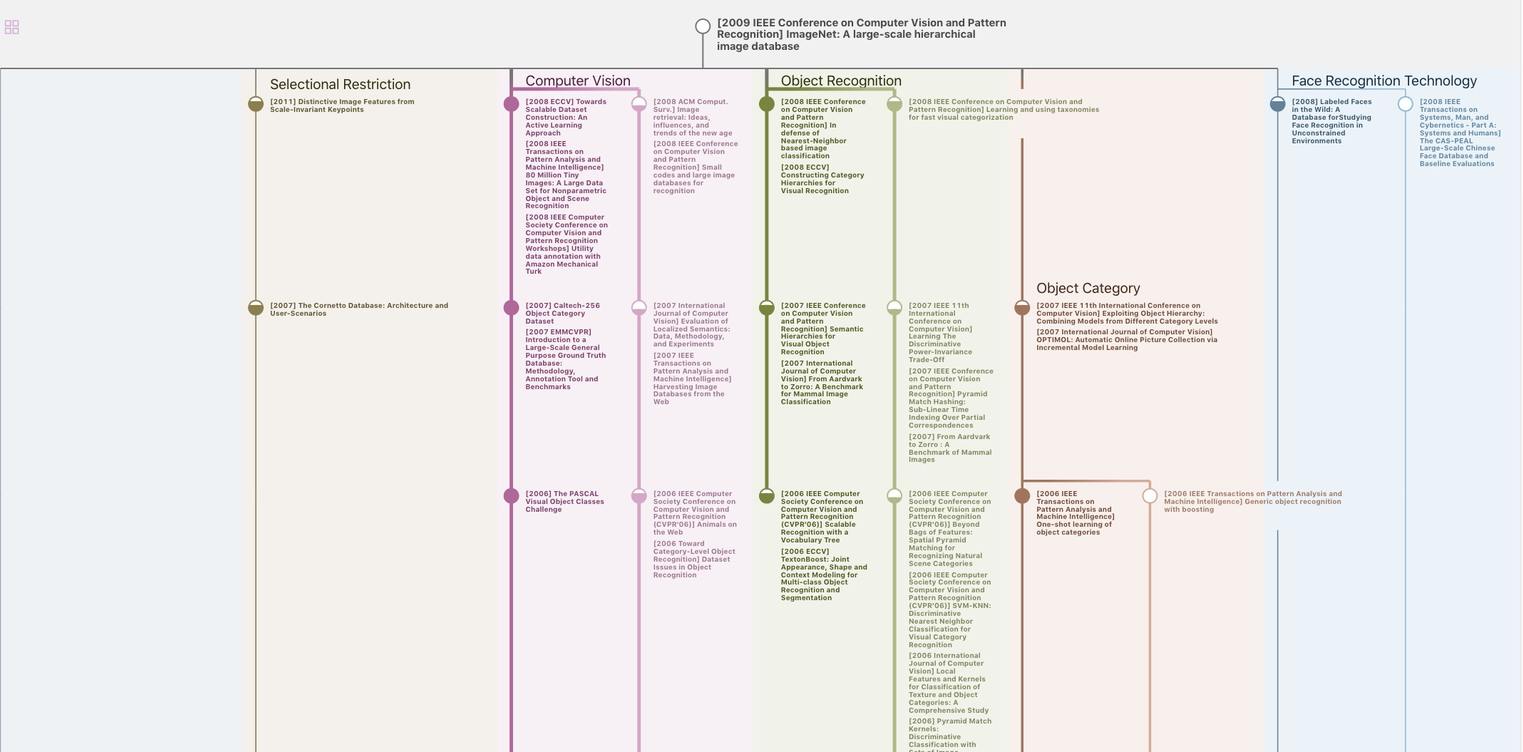
Generate MRT to find the research sequence of this paper
Chat Paper
Summary is being generated by the instructions you defined