Action Unit Memory Network for Weakly Supervised Temporal Action Localization
2021 IEEE/CVF CONFERENCE ON COMPUTER VISION AND PATTERN RECOGNITION, CVPR 2021(2021)
摘要
Weakly supervised temporal action localization aims to detect and localize actions in untrimmed videos with only video-level labels during training. However, without framelevel annotations, it is challenging to achieve localization completeness and relieve background interference. In this paper, we present an Action Unit Memory Network (AUMN) for weakly supervised temporal action localization, which can mitigate the above two challenges by learning an action unit memory bank. In the proposed AUMN, two attention modules are designed to update the memory bank adaptively and learn action units specific classifiers. Furthermore, three effective mechanisms (diversity, homogeneity and sparsity) are designed to guide the updating of the memory network. To the best of our knowledge, this is the first work to explicitly model the action units with a memory network. Extensive experimental results on two standard benchmarks (THUMOS14 and ActivityNet) demonstrate that our AUMN performs favorably against state-of-the-art methods. Specifically, the average mAP of IoU thresholds from 0.1 to 0.5 on the THUMOS14 dataset is significantly improved from 47.0% to 52.1%.
更多查看译文
关键词
weakly supervised temporal action,action unit memory network,localization
AI 理解论文
溯源树
样例
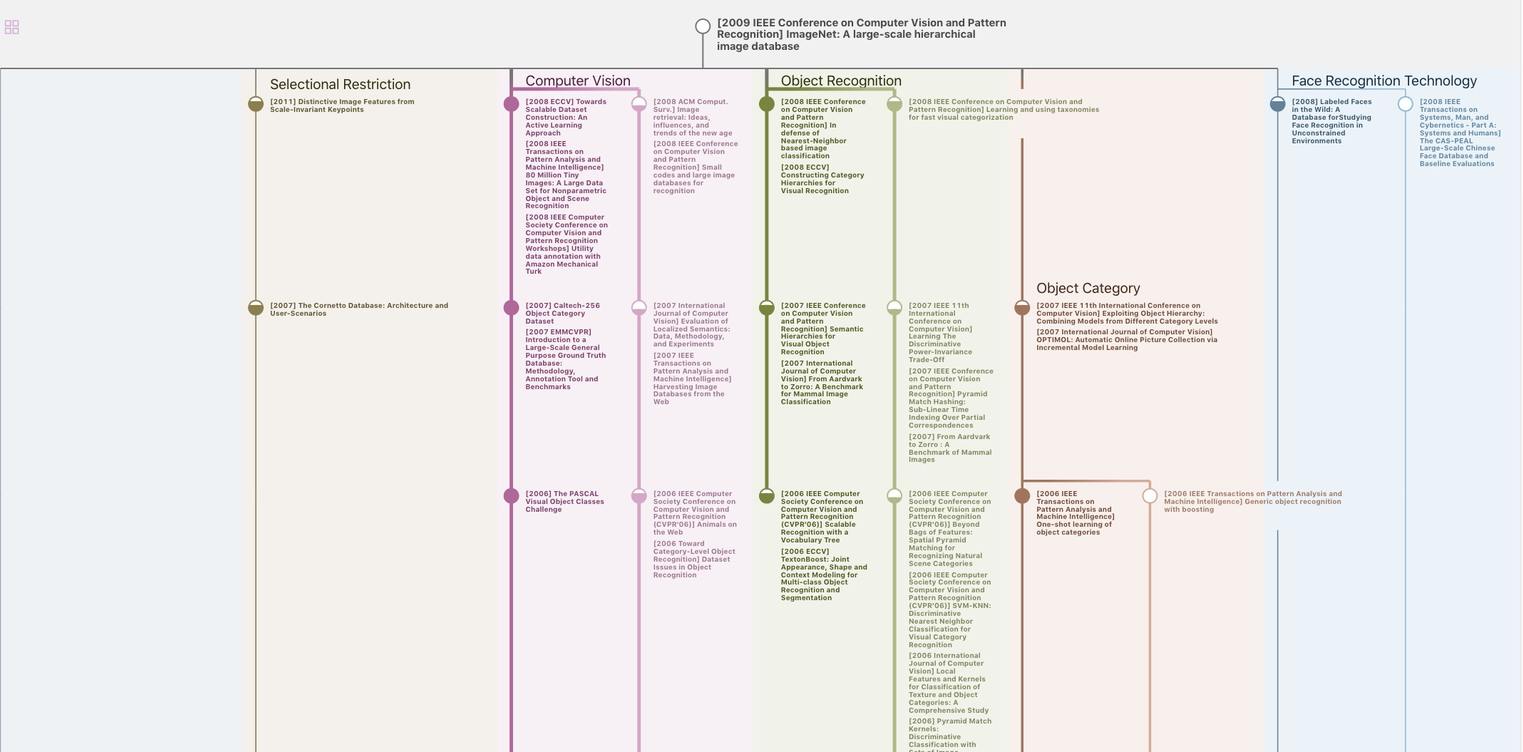
生成溯源树,研究论文发展脉络
Chat Paper
正在生成论文摘要