Automatic Sewer Cracks Localization using Deformable Bounding Boxes.
CSAI(2020)
摘要
The underground sewage system is a crucial part of the infrastructure of any modern municipality across the globe. The underground sewage system is a crucial part of the infrastructure of any modern municipality across the globe. However, with the passage of time, sewer pipes systematically become prone to distortion depending upon the degree of usage and location factors such as the passage of heavy traffic nearby or deep-rooted trees. So, the regular inspection of these pipes requires a lot of technical attention as failure to do so can cause a huge financial and environmental disaster. In this regard, CCTV inspection is considered an effective way of monitoring buried pipes worldwide. As these drainage pipes are extensively spread across several miles, their inspection becomes a time-consuming, tiring, and expensive task. In this study, we propose a crack localization framework by taking the advantages of deep learning algorithms. The framework focuses on the proper organization of input data by using deformable bounding boxes to label the images and prepare them in a suitable format for training the model. An effective Convolutional Neural Network (CNN) model is proposed which addresses the problems of detecting arbitrary-shaped objects, is implemented. The proposed model provides the best tradeoff between speed and accuracy as it uses a segmentation head consisting of a convolutional block with two pivotal attention modules in a sequential manner that can enhance the quality of extracted features with very low computational cost, to support the lightweight ResNet backbone detection algorithm. The model trained with 3150 unique crack image samples, with 700 extra images for validation and testing. To determine the performance of the model, final ground-truth results are compared with testing input bounding box values and other state-of-the-art quantitative metrics e.g. Precision × Recall curve and Average Precision (AP) are explained in which model showed AP value of 80.06%. The model showed the output images in which cracks are localized with excellent accuracy.
更多查看译文
AI 理解论文
溯源树
样例
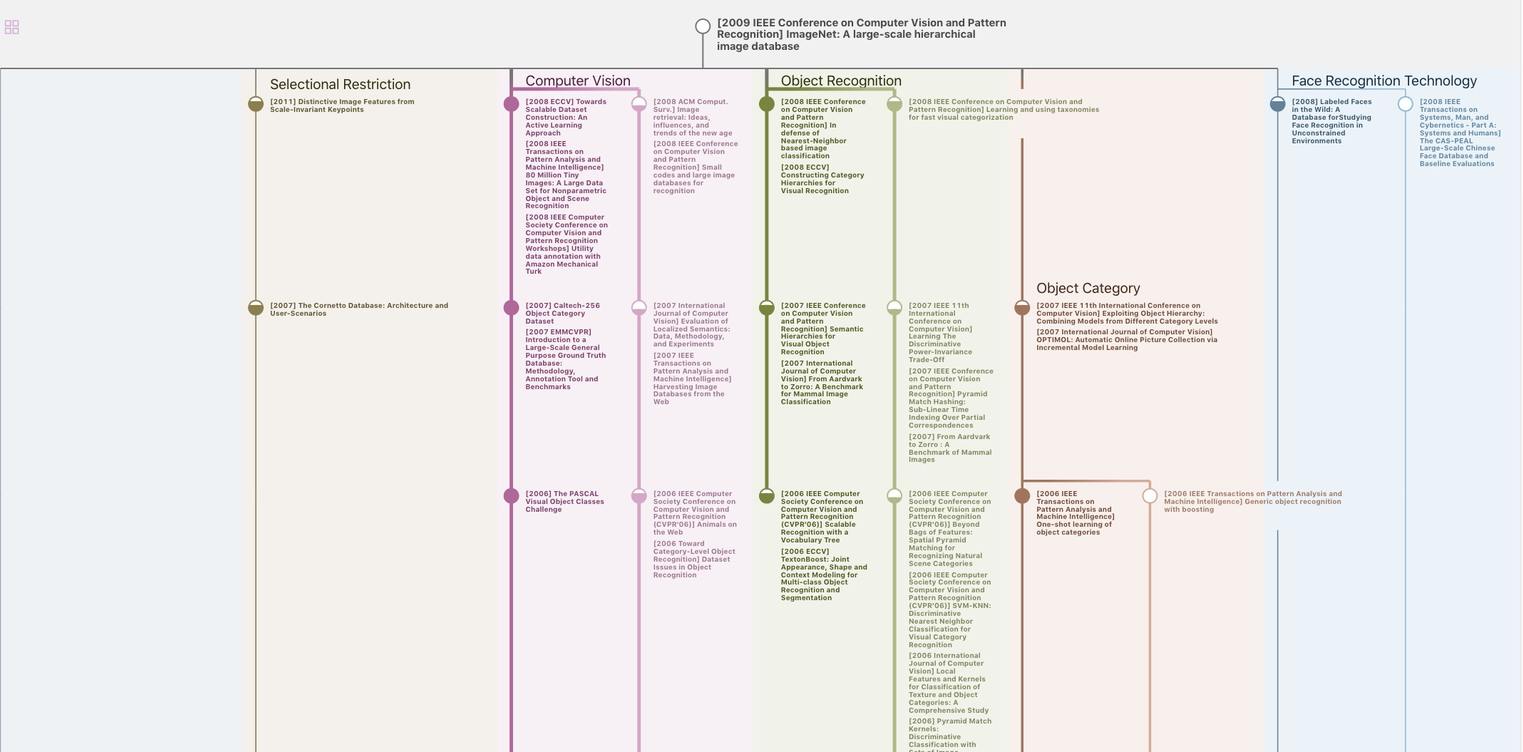
生成溯源树,研究论文发展脉络
Chat Paper
正在生成论文摘要