Automated quantitative thin slice volumetric low dose CT analysis predicts disease severity in COVID-19 patients
CLINICAL IMAGING(2021)
摘要
Purpose: This study aimed to identify predictive (bio-)markers for COVID-19 severity derived from automated quantitative thin slice low dose volumetric CT analysis, clinical chemistry and lung function testing. Methods: Seventy-four COVID-19 patients admitted between March 16th and June 3rd 2020 to the Asklepios Lung Clinic Munich-Gauting, Germany, were included in the study. Patients were categorized in a non-severe group including patients hospitalized on general wards only and in a severe group including patients requiring intensive care treatment. Fully automated quantification of CT scans was performed via IMBIO CT Lung Texture analysisTM software. Predictive biomarkers were assessed with receiver-operator-curve and likelihood analysis. Results: Fifty-five patients (44% female) presented with non-severe COVID-19 and 19 patients (32% female) with severe disease. Five fatalities were reported in the severe group. Accurate automated CT analysis was possible with 61 CTs (82%). Disease severity was linked to lower residual normal lung (72.5% vs 87%, p = 0.003), increased ground glass opacities (GGO) (8% vs 5%, p = 0.031) and increased reticular pattern (8% vs 2%, p = 0.025). Disease severity was associated with advanced age (76 vs 59 years, p = 0.001) and elevated serum Creactive protein (CRP, 92.2 vs 36.3 mg/L, p < 0.001), lactate dehydrogenase (LDH, 485 vs 268 IU/L, p < 0.001) and oxygen supplementation (p < 0.001) upon admission. Predictive risk factors for the development of severe COVID-19 were oxygen supplementation, LDH >313 IU/L, CRP >71 mg/L, <70% normal lung texture, >12.5% GGO and >4.5% reticular pattern. Conclusion: Automated low dose CT analysis upon admission might be a useful tool to predict COVID-19 severity in patients.
更多查看译文
关键词
COVID-19 pneumonia,Low dose volumetric CT,CT analysis
AI 理解论文
溯源树
样例
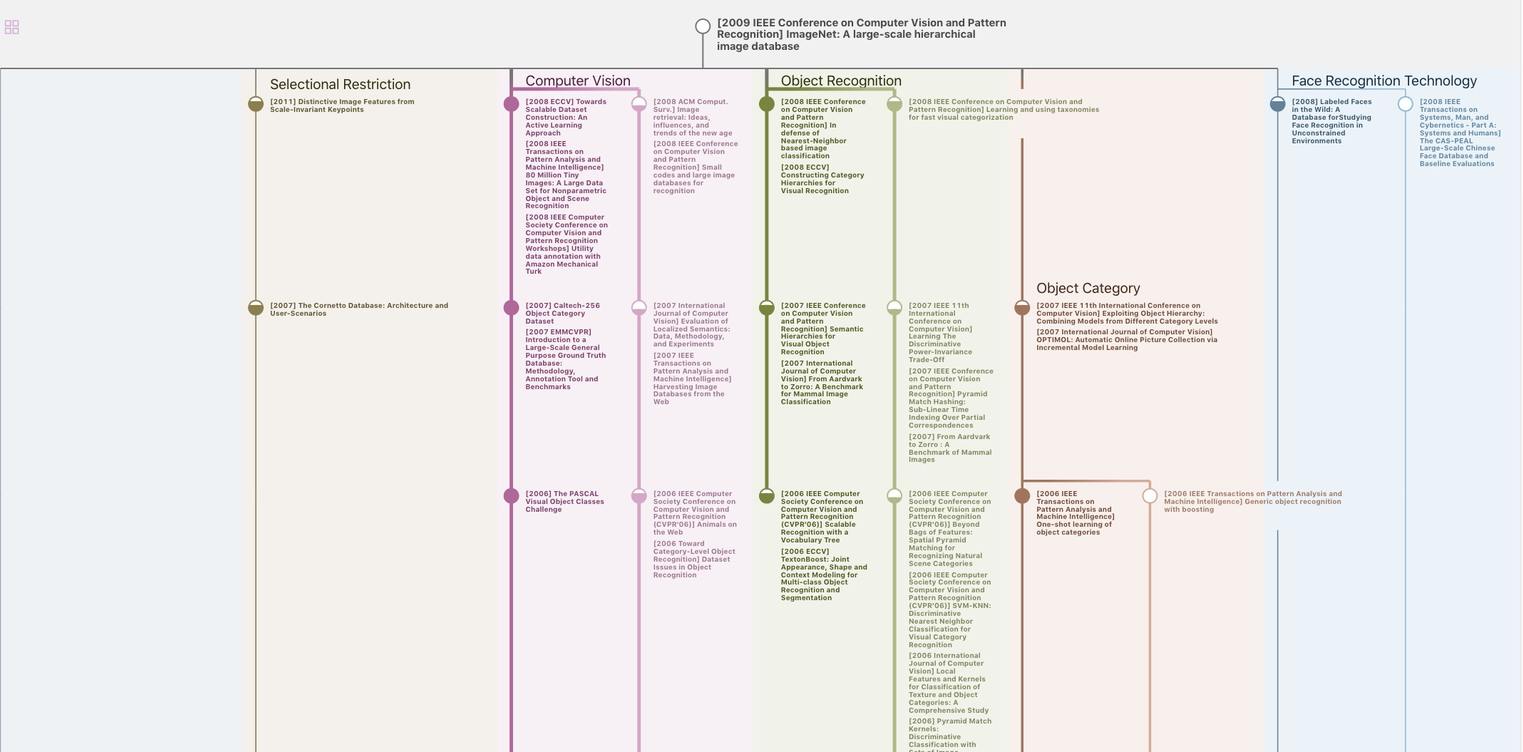
生成溯源树,研究论文发展脉络
Chat Paper
正在生成论文摘要