Learned Spatial Representations for Few-shot Talking-Head Synthesis.
ICCV(2021)
摘要
We propose a novel approach for few-shot talking-head synthesis. While recent works in neural talking heads have produced promising results, they can still produce images that do not preserve the identity of the subject in source images. We posit this is a result of the entangled representation of each subject in a single latent code that models 3D shape information, identity cues, colors, lighting and even background details. In contrast, we propose to factorize the representation of a subject into its spatial and style components. Our method generates a target frame in two steps. First, it predicts a dense spatial layout for the target image. Second, an image generator utilizes the predicted layout for spatial denormalization and synthesizes the target frame. We experimentally show that this disentangled representation leads to a significant improvement over previous methods, both quantitatively and qualitatively.
更多查看译文
关键词
Image and video synthesis,Neural generative models,Representation learning
AI 理解论文
溯源树
样例
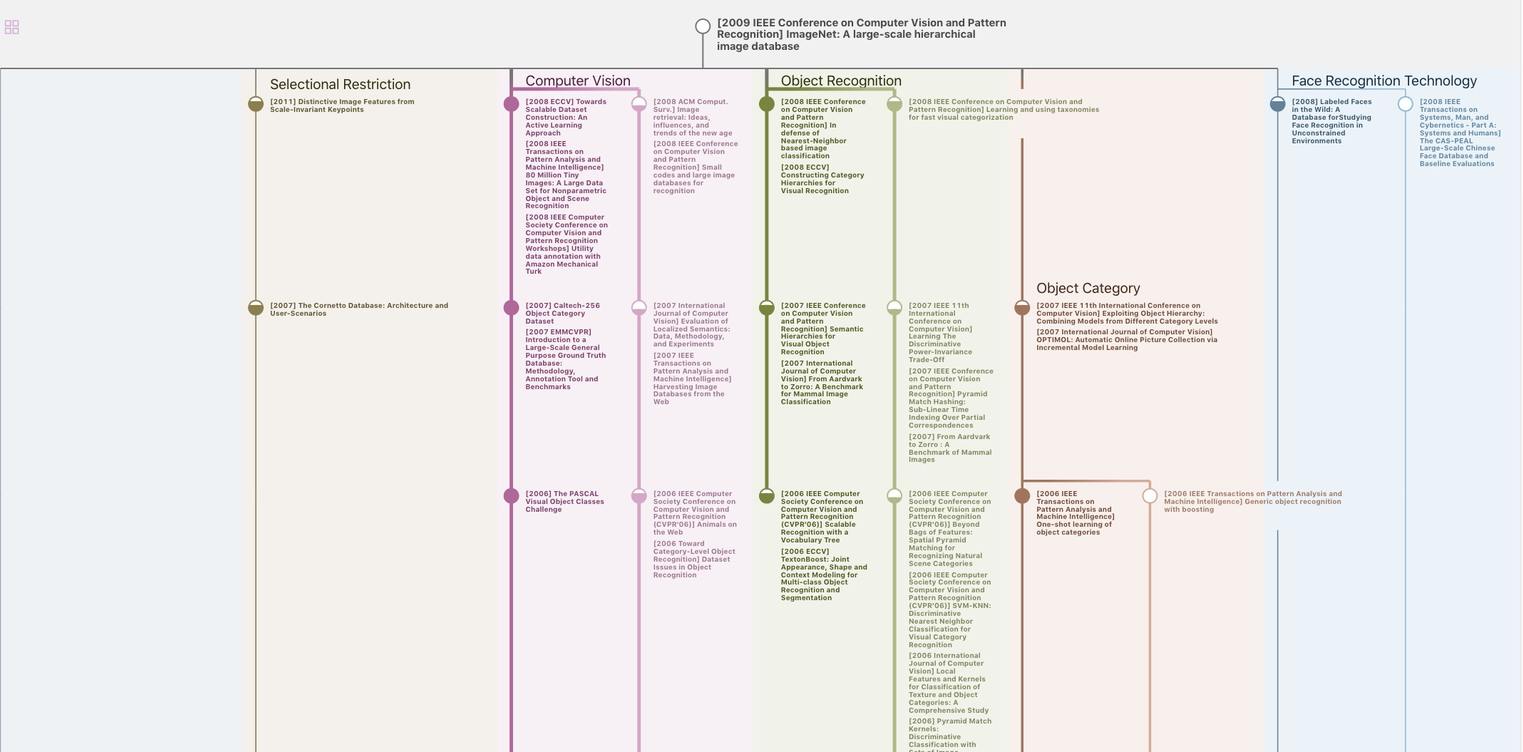
生成溯源树,研究论文发展脉络
Chat Paper
正在生成论文摘要