Optical Gas Sensing With Liquid Crystal Droplets And Convolutional Neural Networks
SENSORS(2021)
摘要
Liquid crystal (LC)-based materials are promising platforms to develop rapid, miniaturised and low-cost gas sensor devices. In hybrid gel films containing LC droplets, characteristic optical texture variations are observed due to orientational transitions of LC molecules in the presence of distinct volatile organic compounds (VOC). Here, we investigate the use of deep convolutional neural networks (CNN) as pattern recognition systems to analyse optical textures dynamics in LC droplets exposed to a set of different VOCs. LC droplets responses to VOCs were video recorded under polarised optical microscopy (POM). CNNs were then used to extract features from the responses and, in separate tasks, to recognise and quantify the vapours exposed to the films. The impact of droplet diameter on the results was also analysed. With our classification models, we show that a single individual droplet can recognise 11 VOCs with small structural and functional differences (F1-score above 93%). The optical texture variation pattern of a droplet also reflects VOC concentration changes, as suggested by applying a regression model to acetone at 0.9-4.0% (v/v) (mean absolute errors below 0.25% (v/v)). The CNN-based methodology is thus a promising approach for VOC sensing using responses from individual LC-droplets.
更多查看译文
关键词
liquid crystal, gas sensing, volatile organic compound, CNN, LSTM, YOLO
AI 理解论文
溯源树
样例
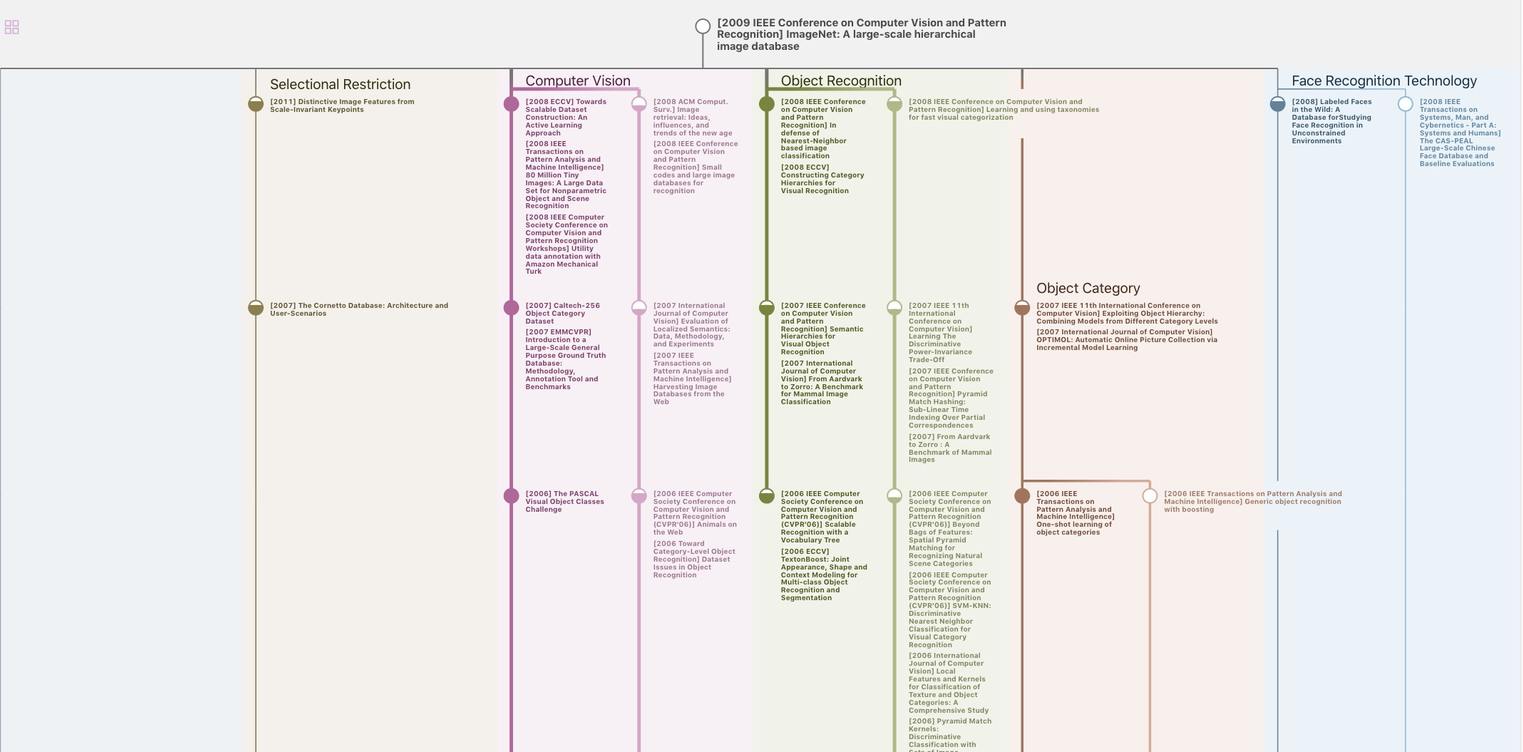
生成溯源树,研究论文发展脉络
Chat Paper
正在生成论文摘要