Using Meta Reinforcement Learning to Bridge the Gap between Simulation and Experiment in Energy Demand Response
e-Energy(2021)
摘要
Our team is proposing to run a full-scale energy demand response experiment in an office building. Although this is an exciting endeavor which will provide value to the community, collecting training data for the reinforcement learning agent is costly and will be limited. In this work, we apply a meta-learning architecture to warm start the experiment with simulated tasks, to increase sample efficiency. We present results that demonstrate a similar a step up in complexity still corresponds with better learning.
更多查看译文
关键词
meta reinforcement learning,energy demand response,reinforcement learning,simulation
AI 理解论文
溯源树
样例
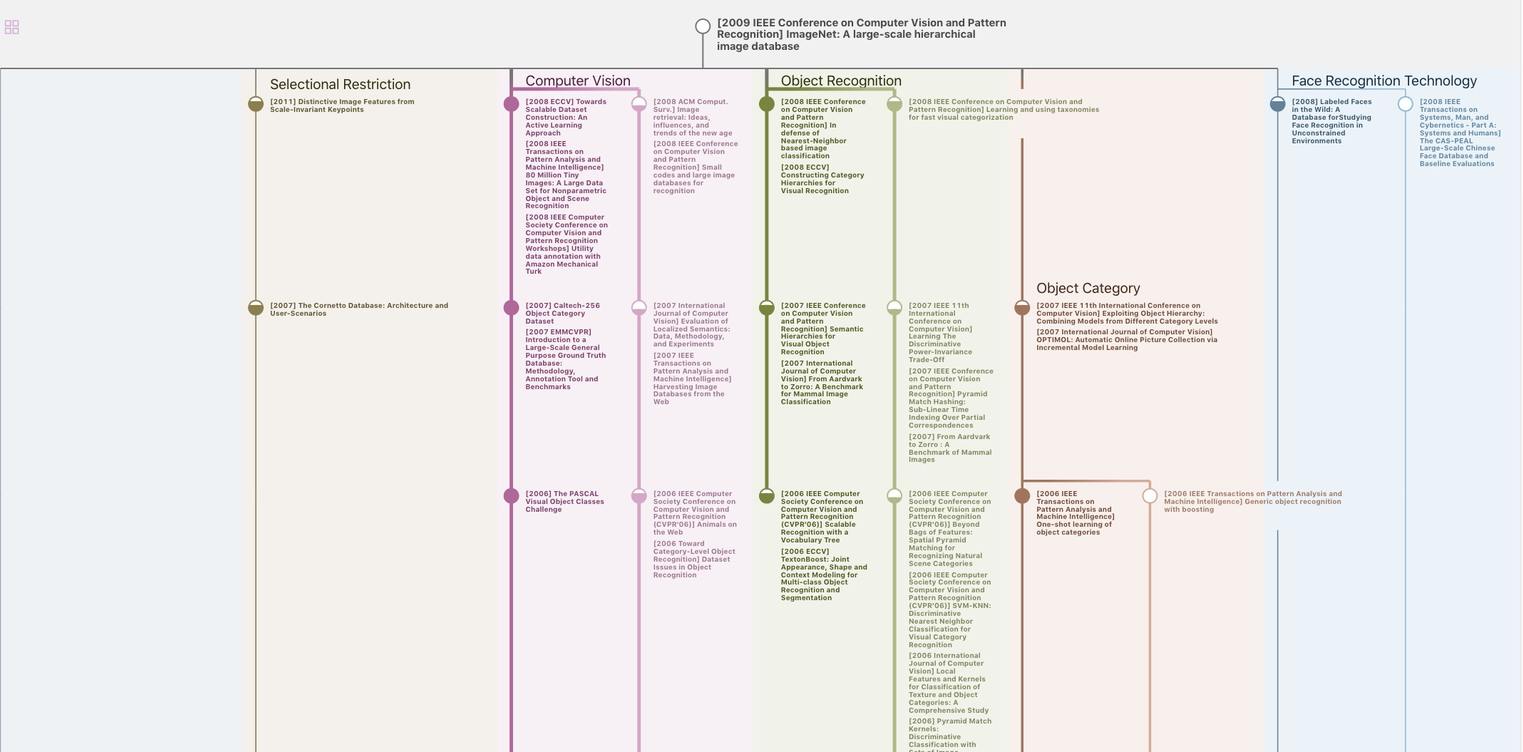
生成溯源树,研究论文发展脉络
Chat Paper
正在生成论文摘要