Updatable Siamese tracker with two-stage one-shot learning
PATTERN RECOGNITION(2024)
摘要
Offline-trained Siamese networks have realized very promising tracking precision and efficiency. However, the performance is still limited by the drawbacks in online update. Traditional strategies cannot tackle the irregular variations of object and the sampling noise, so it is quite risky to adopt them to update Siamese trackers. In this paper, we present a two-stage one-shot learner by exploring the learning scheme of Siamese network, which reveals there are two key issues during online update, i.e., feature fusion and feature comparison. Based on this finding, we propose an updatable Siamese tracker by introducing two independent transformers (SiamTOL). Concretely, a Cross-aware transformer is designed to combine the features of the initial and the dynamic templates, while a Decoder-favored transformer is exploited to compare the fusing template and the search region. By combining these transformers, our tracker is able to adequately model the feature dependencies between multi-frame object samples. Extensive experimental results on several popular benchmarks well manifest that the proposed approach achieves the leading performance, and outperforms other state-of-the-art trackers
更多查看译文
关键词
Visual tracking,Siamese network,Online update,One-shot learning,Transformer
AI 理解论文
溯源树
样例
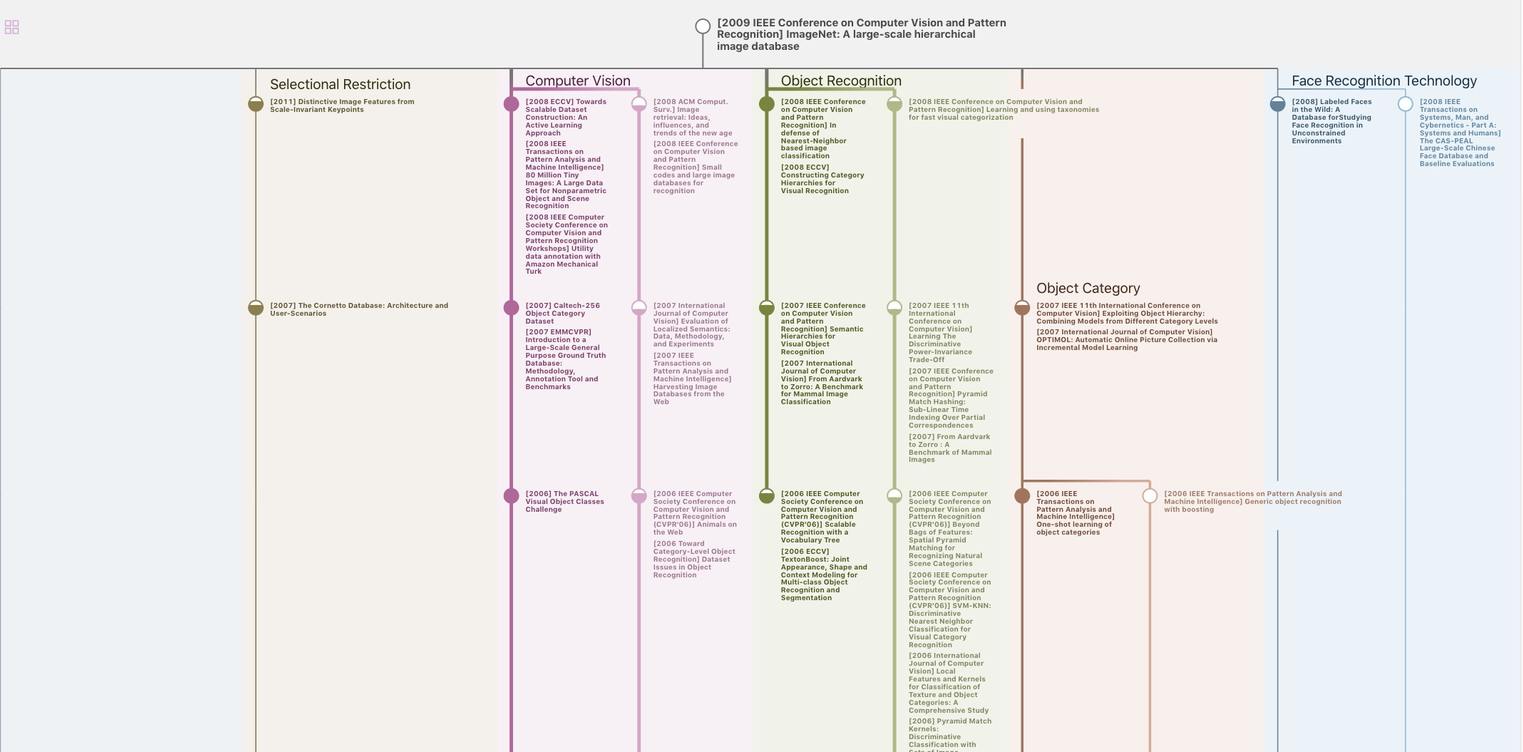
生成溯源树,研究论文发展脉络
Chat Paper
正在生成论文摘要