Gradient-Based Localization and Spatial Attention for Confidence Measure in Fine-Grained Recognition Using Deep Neural Networks.
Proceedings of the AAAI Conference on Artificial Intelligence(2021)
摘要
Both theoretical and practical problems in deep learning classification benefit from assessing uncertainty prediction. In addition, current state-of-the-art methods in this area are computationally expensive: for example, (Loquercio, Segu, and Scaramuzza 2020) is a general method for uncertainty estimation in deep learning that relies on Monte-Carlo sampling. We propose a new, efficient confidence measure later dubbed Over-MAP that utilizes a measure of overlap between structural attention mechanisms and segmentation methods. It does not rely on sampling or retraining. We show that the classification confidence increases with the degree of overlap. The associated confidence and identification tools are conceptually simple, efficient and of high practical interest as they allow for weeding out misleading examples in training data. Our measure is currently deployed in the real-world on widely used platforms to annotate large-scale data efficiently.
更多查看译文
AI 理解论文
溯源树
样例
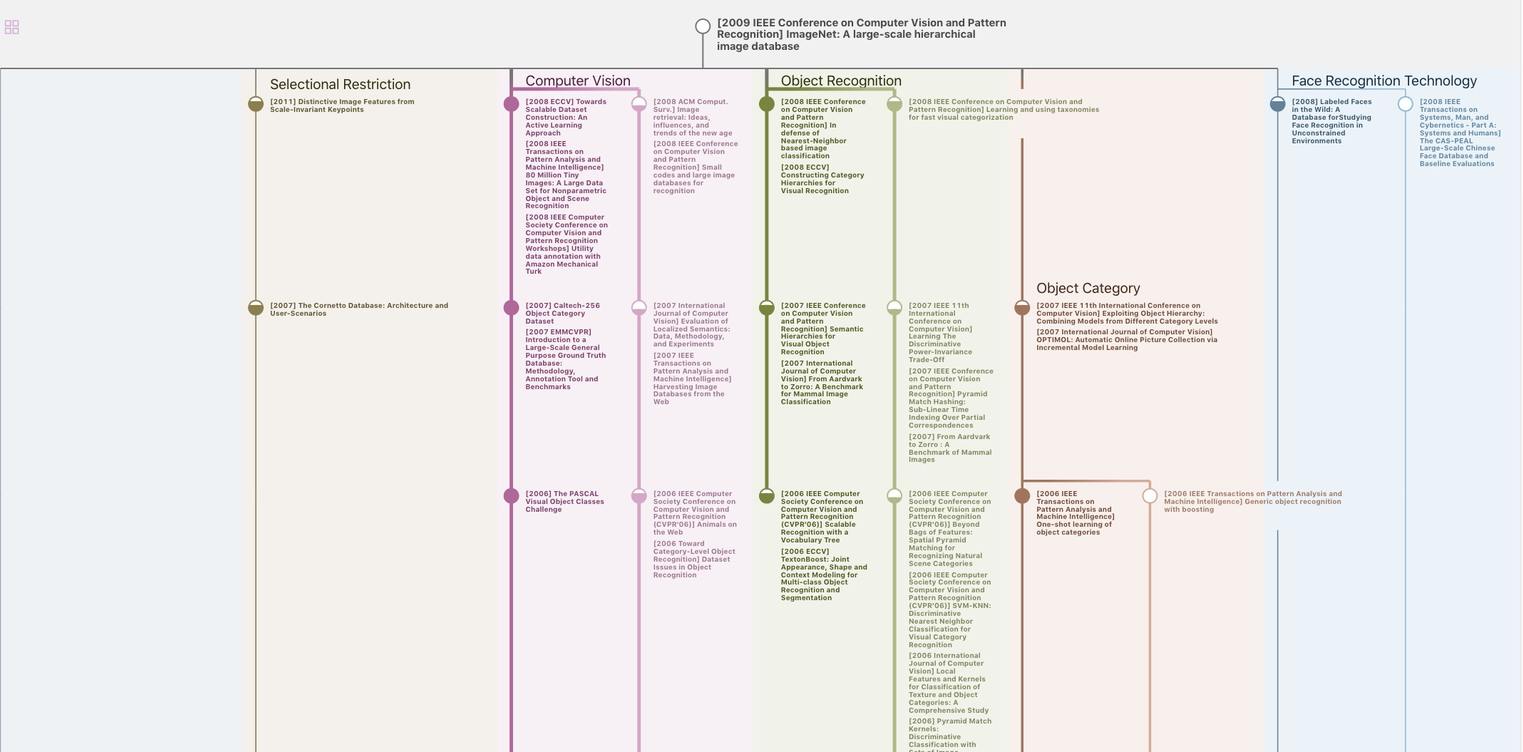
生成溯源树,研究论文发展脉络
Chat Paper
正在生成论文摘要