Adapting Wireless Network Configuration From Simulation to Reality via Deep Learning-Based Domain Adaptation
IEEE-ACM TRANSACTIONS ON NETWORKING(2023)
摘要
Today, wireless mesh networks (WMNs) are deployed globally to support various applications, such as industrial automation, military operations, and smart energy. Significant efforts have been made in the literature to facilitate their deployments and optimize their performance. However, configuring a WMN well is challenging because the network configuration is a complex process, which involves theoretical computation, simulation, and field testing, among other tasks. Our study shows that the models for network configuration prediction learned from simulations may not work well in physical networks because of the simulation-to-reality gap. In this paper, we employ deep learning-based domain adaptation to close the gap and leverage a teacher-student neural network and a physical sampling method to transfer the network configuration knowledge learned from a simulated network to its corresponding physical network. Experimental results show that our method effectively closes the gap and increases the accuracy of predicting a good network configuration that allows the network to meet performance requirements from 30.10% to 70.24% by learning robust machine learning models from a large amount of inexpensive simulation data and a few costly field testing measurements.
更多查看译文
关键词
Wireless sensor networks,Routing,Adaptation models,Wireless networks,Neural networks,Testing,Process control,Network configuration,wireless mesh networks,industrial wireless networks,domain adaptation
AI 理解论文
溯源树
样例
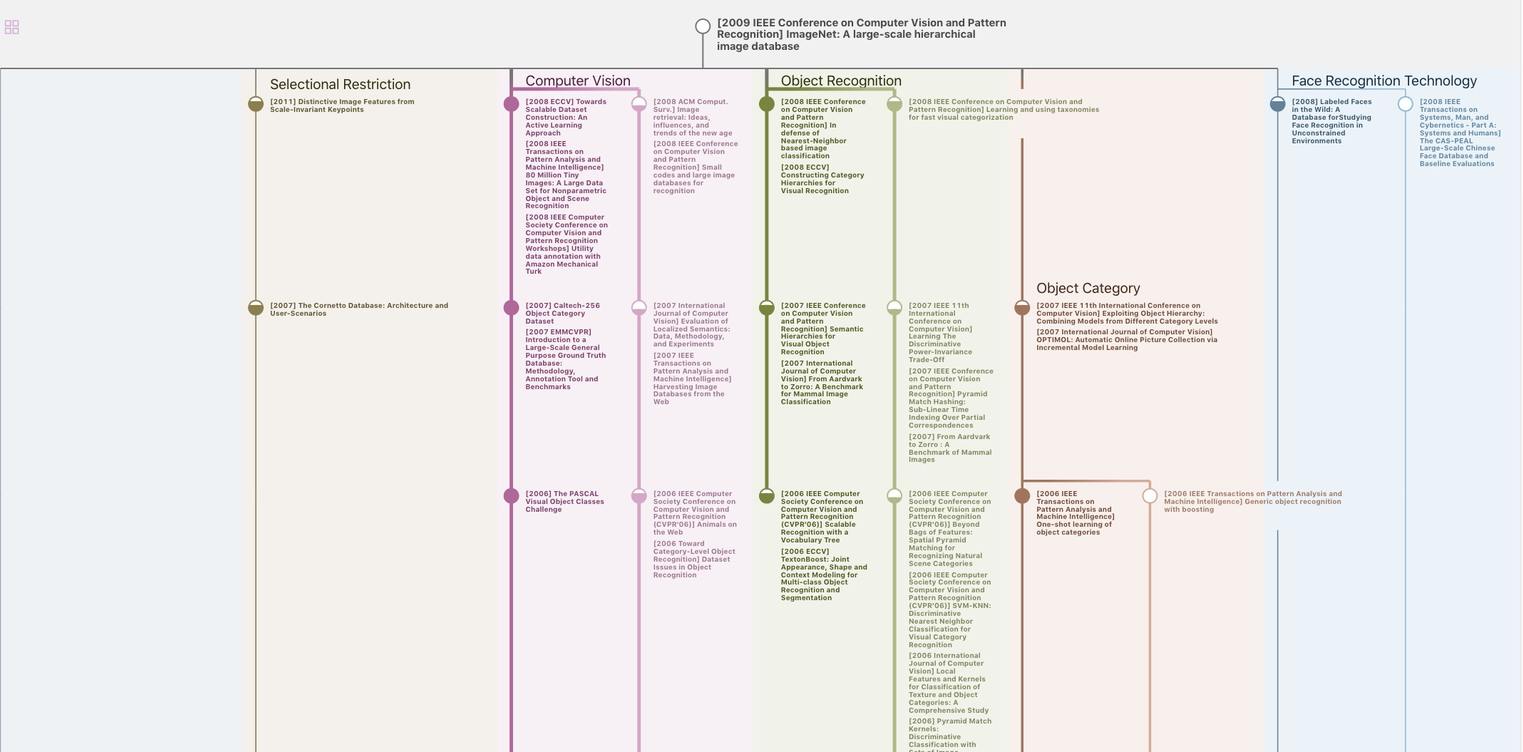
生成溯源树,研究论文发展脉络
Chat Paper
正在生成论文摘要