A High Reliable Computing Offloading Strategy Using Deep Reinforcement Learning for IoVs in Edge Computing
JOURNAL OF GRID COMPUTING(2021)
摘要
In view of the serious problems of increasing delay energy consumption and decreasing service quality caused by complex network state and massive computing data in Internet of vehicles (IOT), a high reliable computing offloading strategy based on edge computing is proposed in this paper. Firstly, the architecture of the Internet of vehicles is designed. The real-time business of terminal vehicles is directly unloaded to the mobile edge computing (MEC) equipment for processing, which reduces the high transmission delay of data on the core network. The combination of software defined network (SDN) and MEC is used to provide flexible network control and centralized resource management for the Internet of vehicles. Then, according to the computing model, communication model and privacy protection model, a joint computing offload and resource allocation strategy is proposed. Finally, taking the shortest time delay and minimum computing cost as the optimization objectives, Q-learning is used to solve the problem to achieve the optimization of unloading strategy, that is, the optimal allocation of communication and computing resources, and the system security is the best. Based on the Matlab simulation platform, the system model is built to carry out the experimental test. The results show that compared with other strategies, the unloading strategy can achieve fast convergence and reduce the system overhead effectively, and the computation complexity, data size and the number of computation nodes have the least impact on the delay.
更多查看译文
关键词
Internet of vehicles, Edge computing, Computing offloading, Software defined network, Privacy protection, Deep reinforcement learning algorithm
AI 理解论文
溯源树
样例
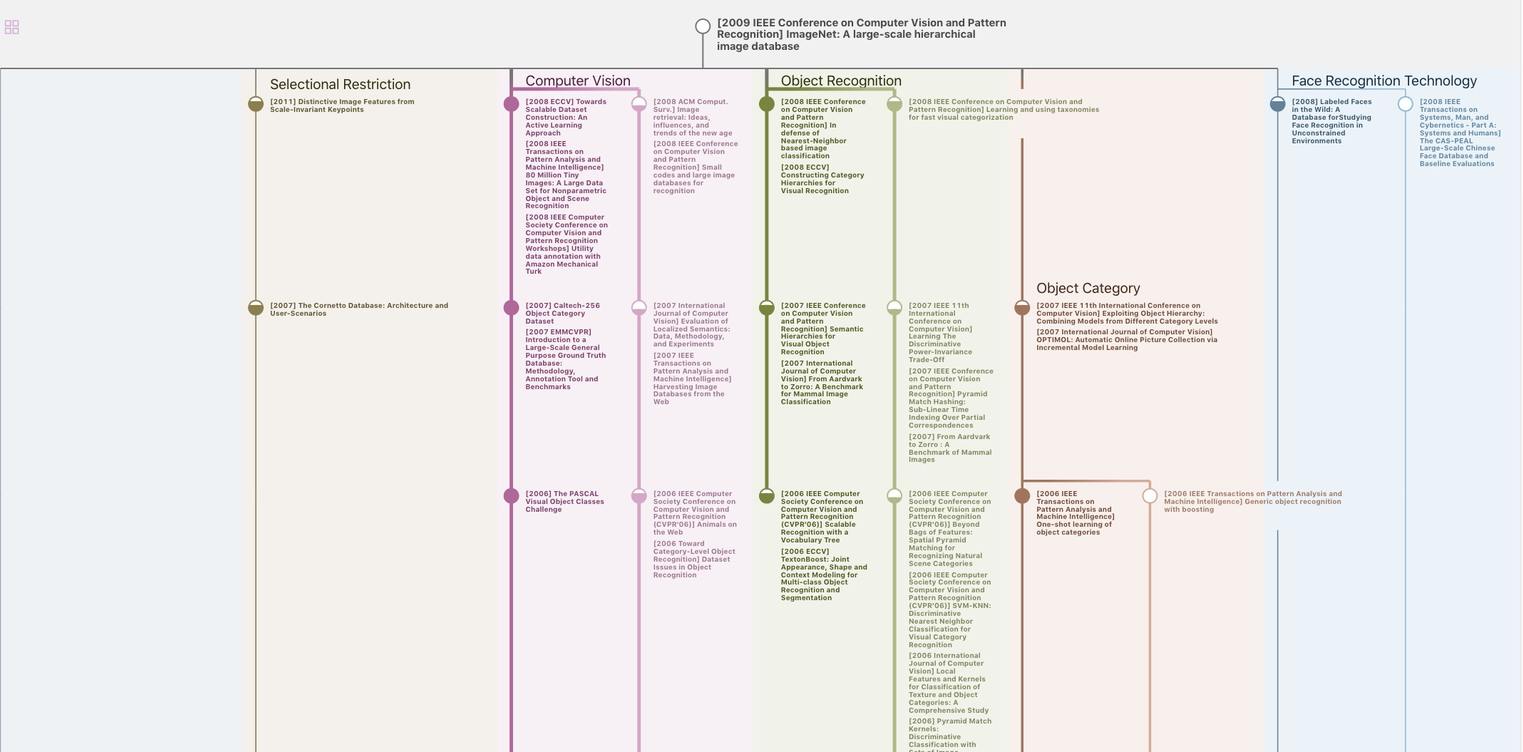
生成溯源树,研究论文发展脉络
Chat Paper
正在生成论文摘要