Visual Foresight Trees for Object Retrieval From Clutter With Nonprehensile Rearrangement
IEEE Robotics and Automation Letters(2022)
摘要
This letter considers the problem of retrieving an object from many tightly packed objects using a combination of robotic pushing and grasping actions. Object retrieval in dense clutter is an important skill for robots to operate in households and everyday environments effectively. The proposed solution, Visual Foresight Tree (
VFT
), intelligently rearranges the clutter surrounding a target object so that it can be grasped easily. Rearrangement with nested nonprehensile actions is challenging as it requires predicting complex object interactions in a combinatorially large configuration space of multiple objects. We first show that a deep neural network can be trained to accurately predict the poses of the packed objects when the robot pushes one of them. The predictive network provides visual foresight and is used in a tree search as a state transition function in the space of scene images. The tree search returns a sequence of consecutive push actions yielding the best arrangement of the clutter for grasping the target object. Experiments in simulation and using a real robot and objects show that the proposed approach outperforms model-free techniques as well as model-based myopic methods both in terms of success rates and the number of executed actions, on several challenging tasks. A video introducing
VFT
, with robot experiments, is accessible at
https://youtu.be/7cL-hmgvyec
. The full source code is available at
https://github.com/arc-l/vft
.
更多查看译文
关键词
Deep learning in grasping and manipulation,learning from experience,visual learning
AI 理解论文
溯源树
样例
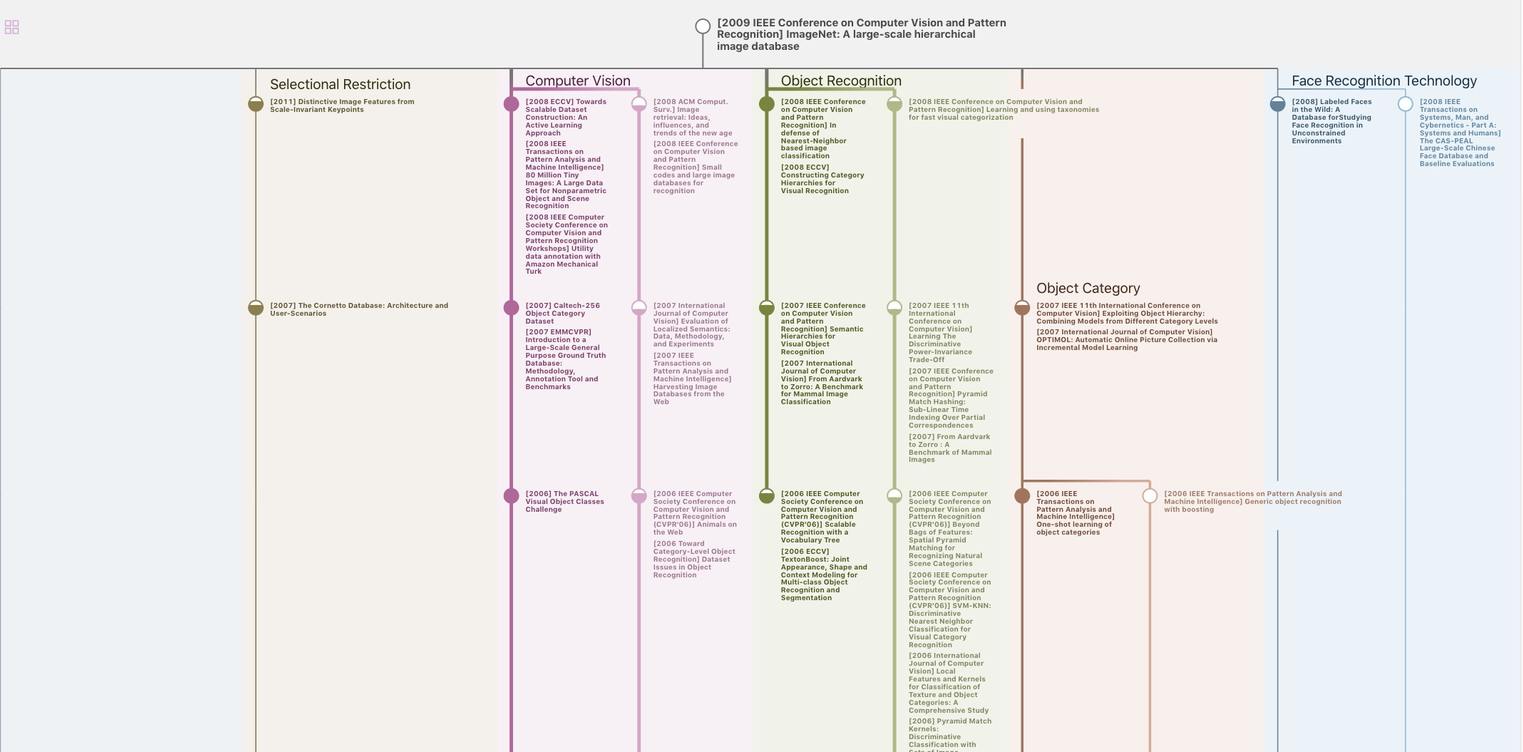
生成溯源树,研究论文发展脉络
Chat Paper
正在生成论文摘要