Convolutional Neural Network to Detect Deep Low-Frequency Tremors from Seismic Waveform Images
TRENDS AND APPLICATIONS IN KNOWLEDGE DISCOVERY AND DATA MINING, PAKDD 2021(2021)
摘要
The installation of dense seismometer arrays in Japan approximately 20 years ago has led to the discovery of deep low-frequency tremors, which are oscillations clearly different from ordinary earthquakes. As such tremors may be related to large earthquakes, it is an important issue in seismology to investigate tremors that occurred before establishing dense seismometer arrays. We use deep learning aiming to detect evidence of tremors from past seismic data of more than 50 years ago, when seismic waveforms were printed on paper. First, we construct a convolutional neural network (CNN) based on the ResNet architecture to extract tremors from seismic waveform images. Experiments applying the CNN to synthetic images generated according to seismograph paper records show that the trained model can correctly determine the presence of tremors in the seismic waveforms. In addition, the gradient-weighted class activation mapping clearly indicates the tremor location on each image. Thus, the proposed CNN has a strong potential for detecting tremors on numerous paper records, which can enable to deepen the understanding of the relations between tremors and earthquakes.
更多查看译文
关键词
Deep low-frequency tremor, Convolutional neural network, ResNet, Gradient-weighted class activation mapping
AI 理解论文
溯源树
样例
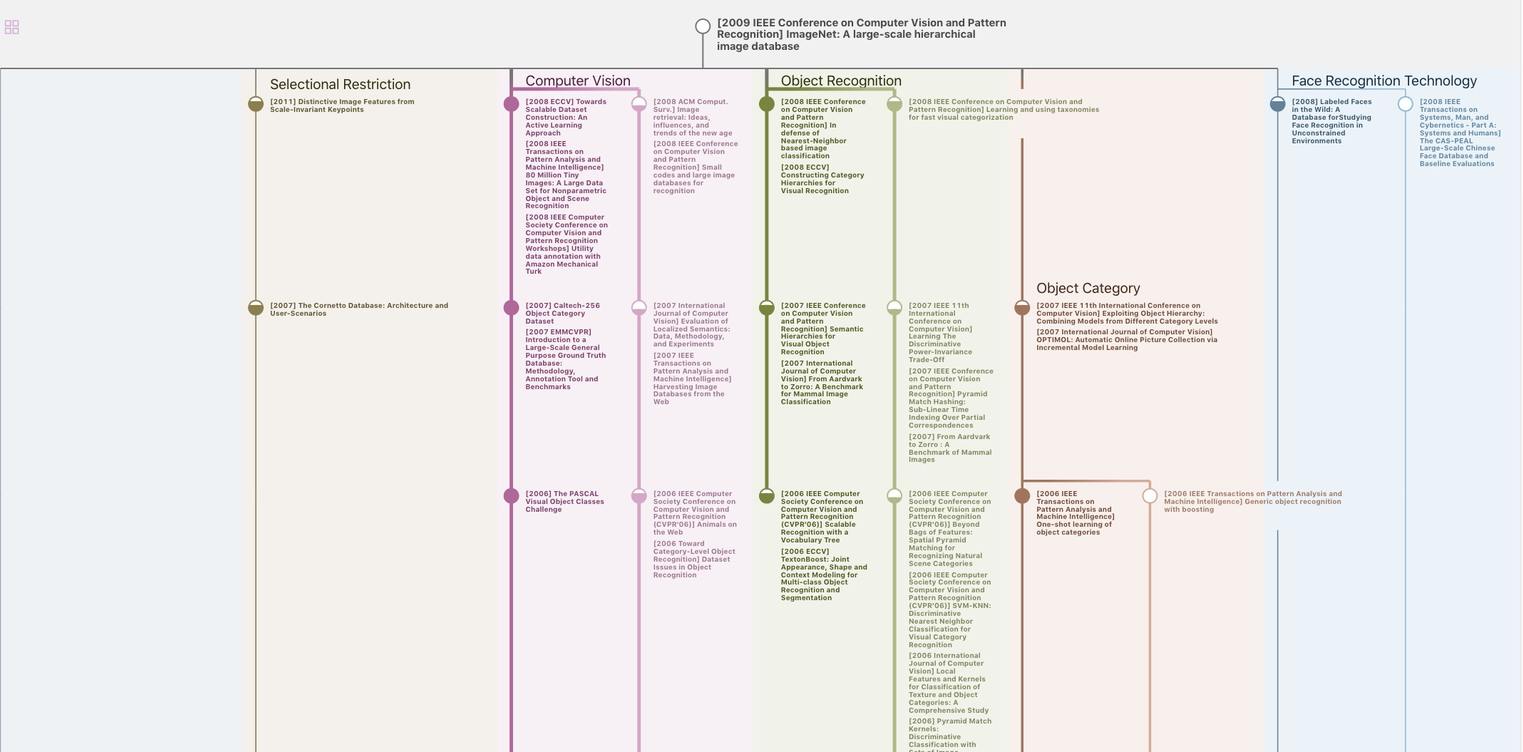
生成溯源树,研究论文发展脉络
Chat Paper
正在生成论文摘要