From Early Biological Models To Cnns: Do They Look Where Humans Look?
2020 25TH INTERNATIONAL CONFERENCE ON PATTERN RECOGNITION (ICPR)(2020)
摘要
Early hierarchical computational visual models as well as recent deep neural networks have been inspired by the functioning of the primate visual cortex system. Although much effort has been made to dissect neural networks to visualize the features they learn at the individual units, the scope of the visualizations has been limited to a categorization of the features in terms of their semantic level. Considering the ability humans have to select high semantic level regions of a scene, the question whether neural networks can match this ability, and if similarity with humans attention is correlated with neural networks performance naturally arise. To address this question we propose a pipeline to select and compare sets of feature points that maximally activate individual networks units to human fixations. We extract features from a variety of neural networks, from early hierarchical models such as HMAX up to recent deep convolutional neural netwoks such as Densnet, to compare them to human fixations. Experiments over the ETD database show that human fixations correlate with CNNs features from deep layers significantly better than with random sets of points, while they do not with features extracted from the first layers of CNNs, nor with the HMAX features, which seem to have low semantic level compared with the features that respond to the automatically learned filters of CNNs. It also turns out that there is a correlation between CNN's human similarity and classification performance.
更多查看译文
关键词
primate visual cortex system,high semantic level regions,feature points,CNN features,HMAX features,biological models,hierarchical computational visual models,CNN human similarity,deep convolutional neural netwoks,individual networks units,Densnet
AI 理解论文
溯源树
样例
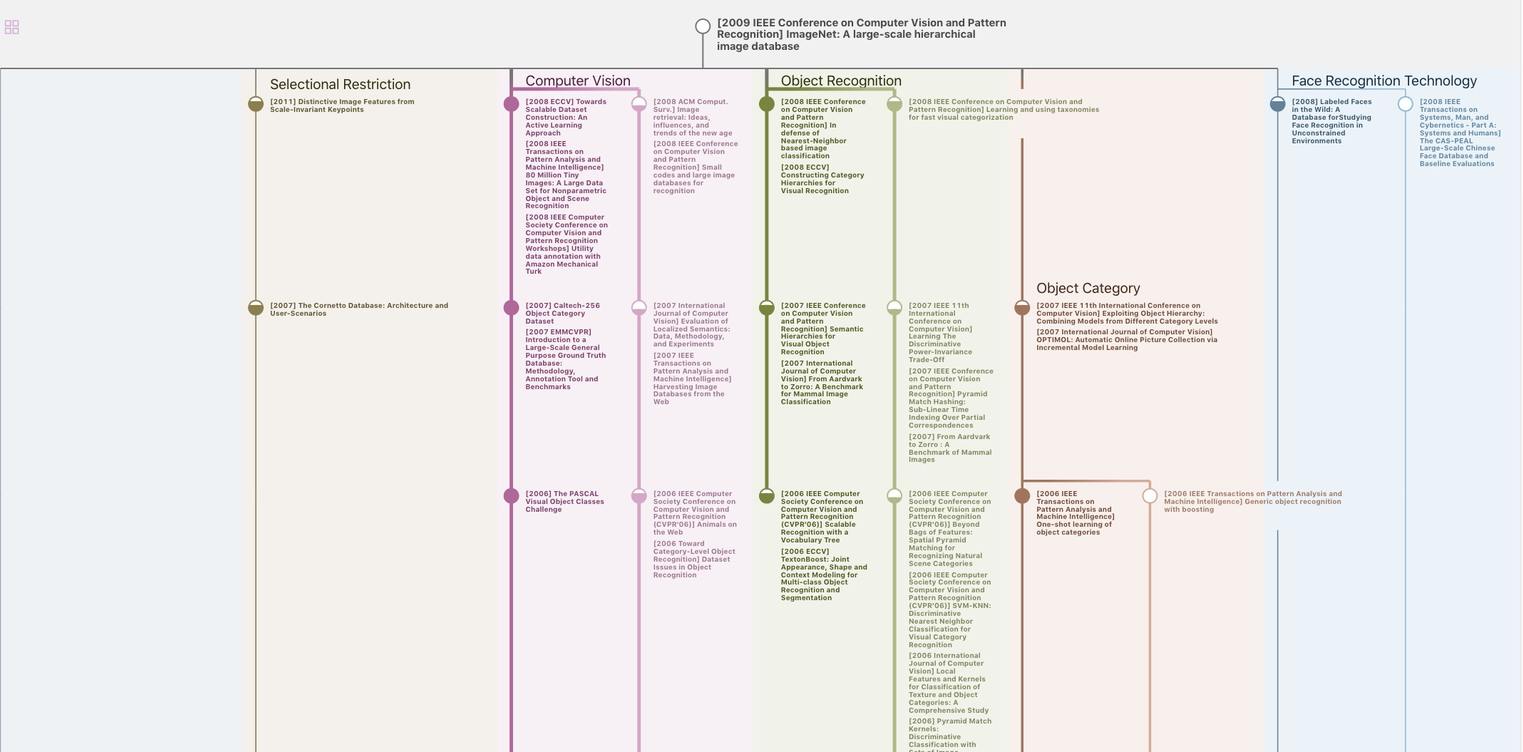
生成溯源树,研究论文发展脉络
Chat Paper
正在生成论文摘要