Second-order Attention Guided Convolutional Activations for Visual Recognition
2020 25TH INTERNATIONAL CONFERENCE ON PATTERN RECOGNITION (ICPR)(2021)
摘要
Recently, modeling deep convolutional activations by the global second-order pooling has shown great advance on visual recognition tasks. However, most of the existing deep second-order statistical models mainly compute second-order statistics of activations of the last convolutional layer as image representations, and they seldom introduce second-order statistics into earlier layers to better fit network topology, thus limiting the representational ability to a certain extent. Motivated by the flexibility of attention blocks that are commonly plugged into intermediate layers of deep convolutional networks (ConvNets), this work makes an attempt to combine deep second-order statistics with attention mechanisms in ConvNets, and further proposes a novel Second-order Attention Guided Network (SoAG-Net) for visual recognition. More specifically, SoAG-Net involves several SoAG modules seemingly inserted into intermediate layers of the network, in which SoAG collects second-order statistics of convolutional activations by polynomial kernel approximation to predict channel-wise attention maps utilized for guiding the learning of convolutional activations through tensor scaling along channel dimension. SoAG improves the nonlinearity of ConvNets and enables ConvNets to fit more complicated distribution of convolutional activations. Experiment results on three commonly used datasets illuminate that SoAG-Net outperforms its counterparts and achieves competitive performance with state-of-the-art models under the same backbone.
更多查看译文
关键词
second-order statistics,channel attention,deep convolutional networks,visual recognition
AI 理解论文
溯源树
样例
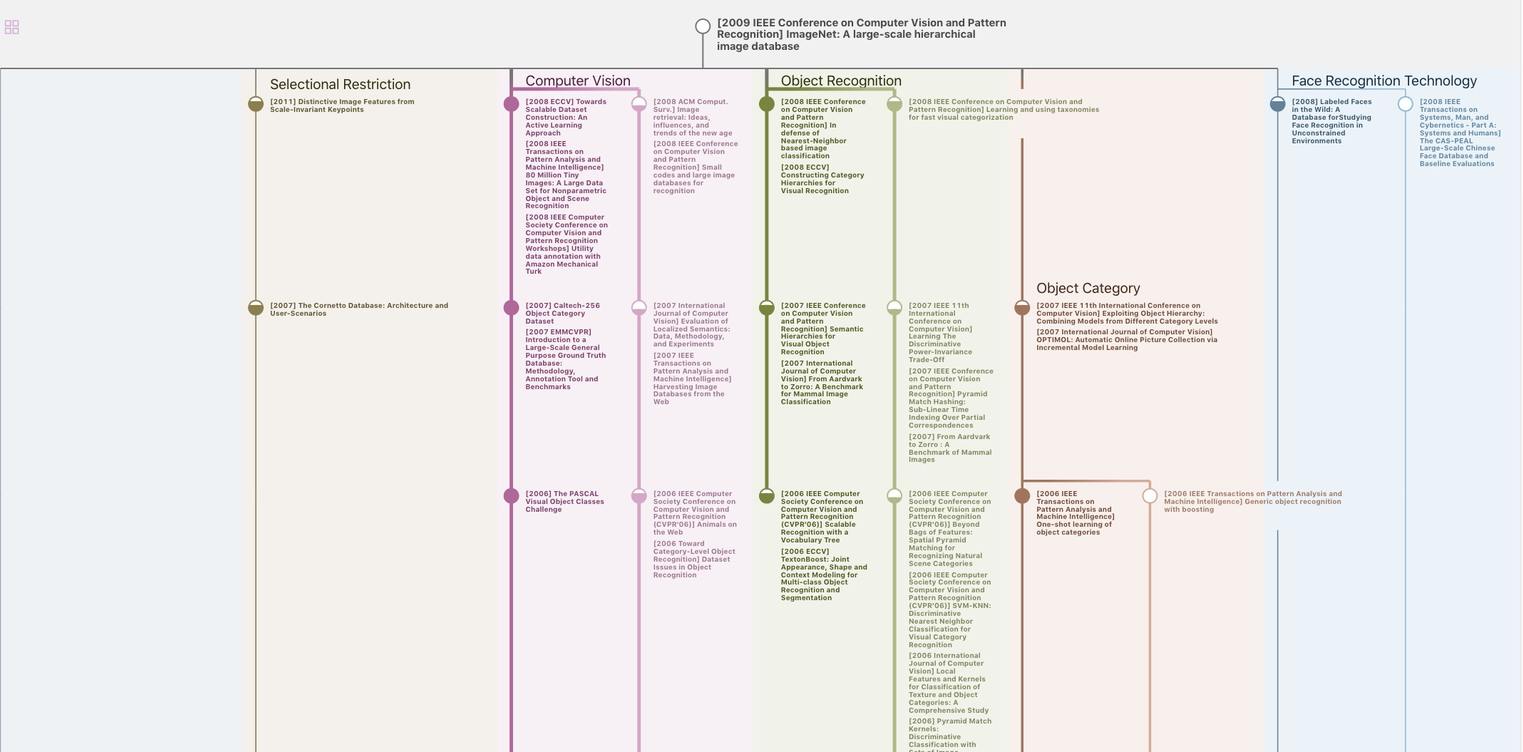
生成溯源树,研究论文发展脉络
Chat Paper
正在生成论文摘要